ColO-RAN: Developing Machine Learning-Based xApps for Open RAN Closed-Loop Control on Programmable Experimental Platforms
IEEE Transactions on Mobile Computing(2023)
摘要
Cellular networks are undergoing a radical transformation toward disaggregated, fully virtualized, and programmable architectures with increasingly heterogeneous devices and applications. In this context, the open architecture standardized by the O-RAN Alliance enables algorithmic and hardware-independent Radio Access Network (RAN) adaptation through closed-loop control. O-RAN introduces Machine Learning (ML)-based network control and automation algorithms as so-called
xApps
running on RAN Intelligent Controllers . However, in spite of the new opportunities brought about by the Open RAN, advances in ML-based network automation have been slow, mainly because of the unavailability of large-scale datasets and experimental testing infrastructure. This slows down the development and widespread adoption of Deep Reinforcement Learning (DRL) agents on real networks, delaying progress in intelligent and autonomous RAN control. In this paper, we address these challenges by discussing insights and practical solutions for the design, training, testing, and experimental evaluation of DRL-based closed-loop control in the Open RAN. To this end, we introduce ColO-RAN, the first publicly-available large-scale O-RAN testing framework with software-defined radios-in-the-loop. Building on the scale and computational capabilities of the Colosseum wireless network emulator, ColO-RAN enables ML research at scale using O-RAN components, programmable base stations, and a “wireless data factory.” Specifically, we design and develop three exemplary xApps for DRL-based control of RAN slicing, scheduling and online model training, and evaluate their performance on a cellular network with 7 softwarized base stations and 42 users. Finally, we showcase the portability of ColO-RAN to different platforms by deploying it on Arena, an indoor programmable testbed. The lessons learned from the ColO-RAN implementation and the extensive results from our first-of-its-kind large-scale evaluation highlight the importance of experimental frameworks for the development of end-to-end intelligent RAN control pipelines, from data analysis to the design and testing of DRL agents. They also provide insights on the challenges and benefits of DRL-based adaptive control, and on the trade-offs associated to training on a live RAN. ColO-RAN and the collected large-scale dataset are publicly available to the research community.
更多查看译文
关键词
programmable experimental platforms,xapps,open colo-ran,control,learning-based,closed-loop
AI 理解论文
溯源树
样例
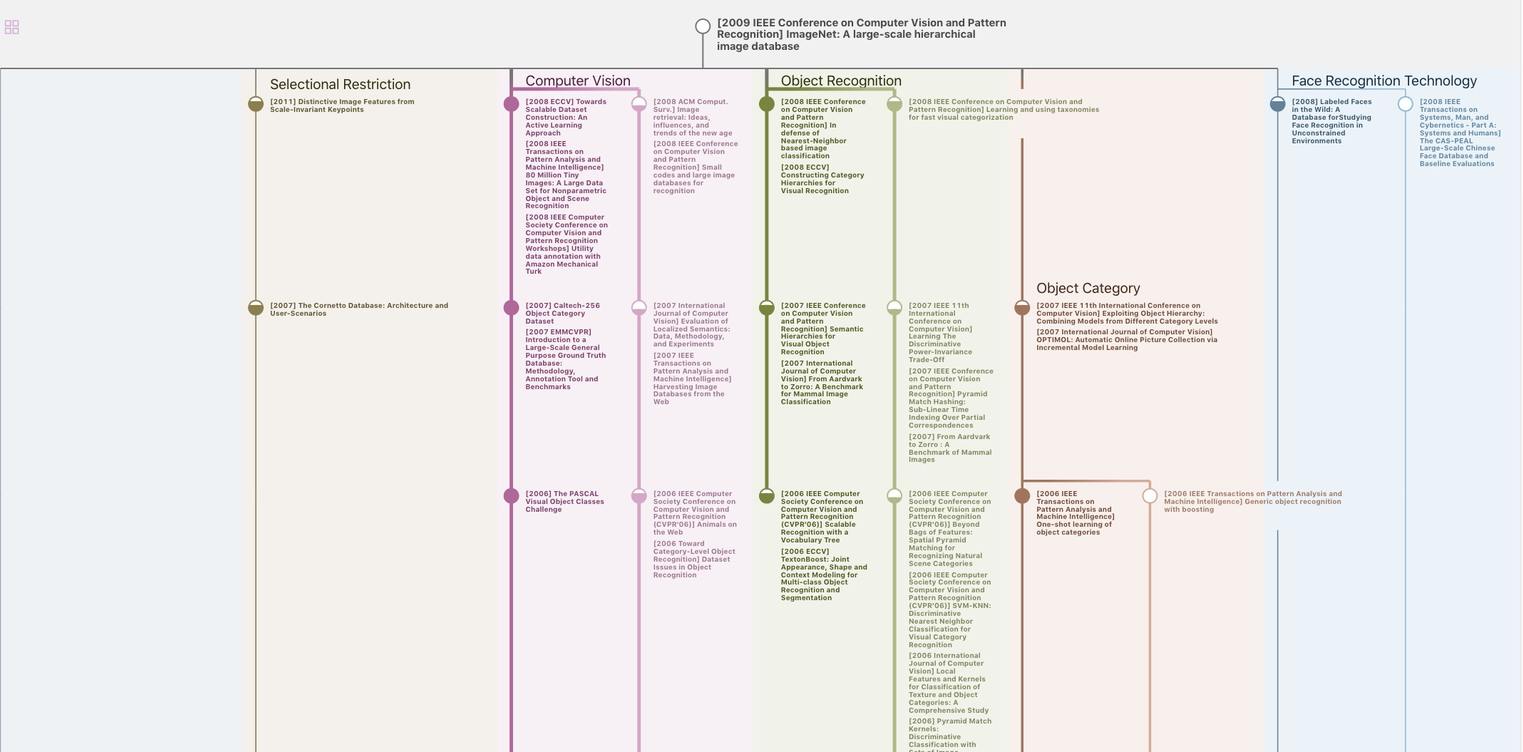
生成溯源树,研究论文发展脉络
Chat Paper
正在生成论文摘要