Adaptation: Blessing or Curse for Higher Way Meta-Learning.
IEEE Trans. Artif. Intell.(2024)
摘要
The prevailing literature typically assesses the ef-fectiveness of Meta-learning (ML) approaches on tasks that involve no more than 20 classes. However, we challenge this convention by conducting a more complex and natural task setup to test the fundamental initialization, metric, and optimization approaches. In particular, we increase the number of classes in the Omniglot and tieredImagenet datasets to 200 and 90, respectively. Interestingly, we observe that as the number of classes increases, ML approaches perform in reverse order of their degree of adaptation, with ProtoNet outperforming ANIL and MAML. ProtoNet, which does not require adaptation, is marginally affected by the increase in task complexity, while ANIL and MAML are highly affected. Despite performing full feature backbone and classifier adaptation, MetaLSTM++ exhibits an intriguing behavior of performing well. To this end, we analyze the backbone learned by different algorithms and the influence of adaptation from different perspectives. We uncover that ProtoNet learns better, and MetaLSTM++ learns the worst backbone, but the generalizability to unseen data for MetaLSTM++ is compensated by powerful adaptation to the meta-test support sets, due to its learned data-driven optimizer.
更多查看译文
关键词
Adaptation,Initialization,Optimizer,Task-complexity
AI 理解论文
溯源树
样例
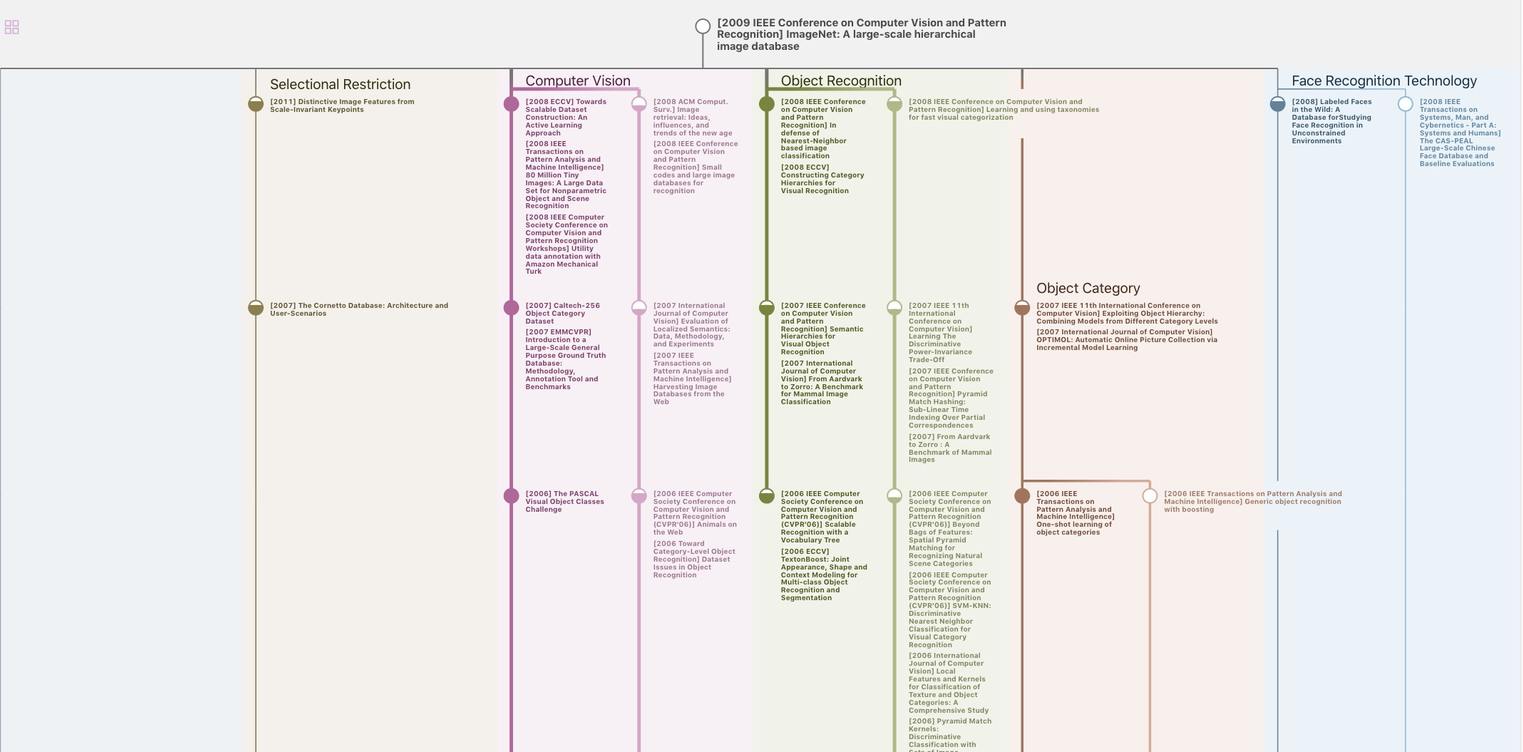
生成溯源树,研究论文发展脉络
Chat Paper
正在生成论文摘要