Progressive-Hint Prompting Improves Reasoning in Large Language Models
arXiv (Cornell University)(2023)
摘要
The performance of Large Language Models (LLMs) in reasoning tasks depends heavily on prompt design, with Chain-of-Thought (CoT) and self-consistency being critical methods that enhance this ability. However, these methods do not fully exploit the answers generated by the LLM to guide subsequent responses. This paper proposes a new prompting method, named Progressive-Hint Prompting (PHP), that enables automatic multiple interactions between users and LLMs by using previously generated answers as hints to progressively guide toward the correct answers. PHP is orthogonal to CoT and self-consistency, making it easy to combine with state-of-the-art techniques to further improve performance. We conducted extensive and comprehensive experiments on seven benchmarks. The results show that PHP significantly improves accuracy while remaining highly efficient. For instance, with text-davinci-003, we observed a 4.2% improvement on GSM8K with greedy decoding compared to Complex CoT, and a 46.17% reduction in sample paths with self-consistency. With GPT-4 and PHP, we achieve state-of-the-art performances on SVAMP (89.1% -> 91.9%), GSM8K (92% -> 95.5%), AQuA (76.4% -> 79.9%) and MATH (50.3% -> 53.9%).
更多查看译文
关键词
reasoning,language,models,progressive-hint
AI 理解论文
溯源树
样例
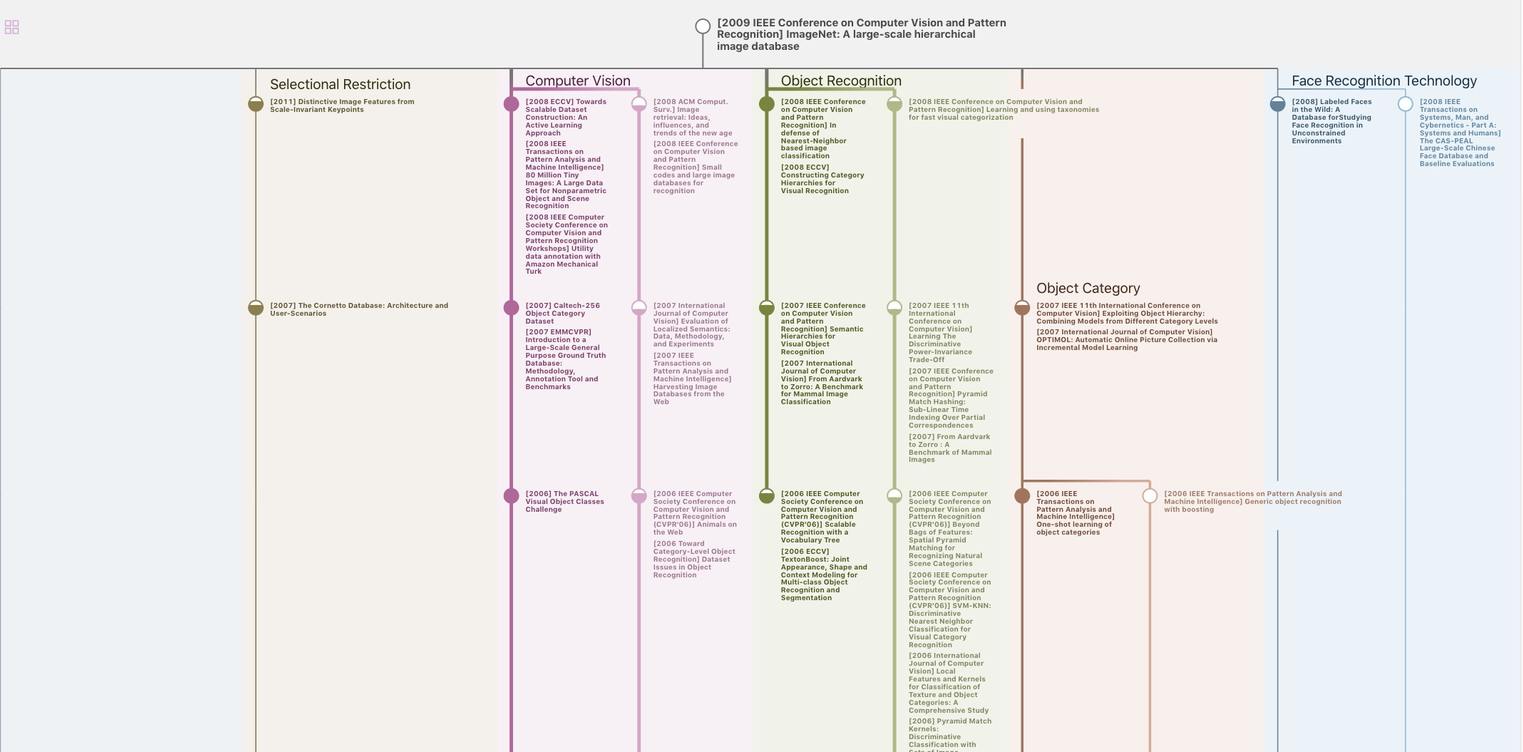
生成溯源树,研究论文发展脉络
Chat Paper
正在生成论文摘要