SecureBoost Hyperparameter Tuning via Multi-Objective Federated Learning
arXiv (Cornell University)(2023)
摘要
SecureBoost is a tree-boosting algorithm leveraging homomorphic encryption to protect data privacy in vertical federated learning setting. It is widely used in fields such as finance and healthcare due to its interpretability, effectiveness, and privacy-preserving capability. However, SecureBoost suffers from high computational complexity and risk of label leakage. To harness the full potential of SecureBoost, hyperparameters of SecureBoost should be carefully chosen to strike an optimal balance between utility, efficiency, and privacy. Existing methods either set hyperparameters empirically or heuristically, which are far from optimal. To fill this gap, we propose a Constrained Multi-Objective SecureBoost (CMOSB) algorithm to find Pareto optimal solutions that each solution is a set of hyperparameters achieving optimal tradeoff between utility loss, training cost, and privacy leakage. We design measurements of the three objectives. In particular, the privacy leakage is measured using our proposed instance clustering attack. Experimental results demonstrate that the CMOSB yields not only hyperparameters superior to the baseline but also optimal sets of hyperparameters that can support the flexible requirements of FL participants.
更多查看译文
关键词
learning,multi-objective
AI 理解论文
溯源树
样例
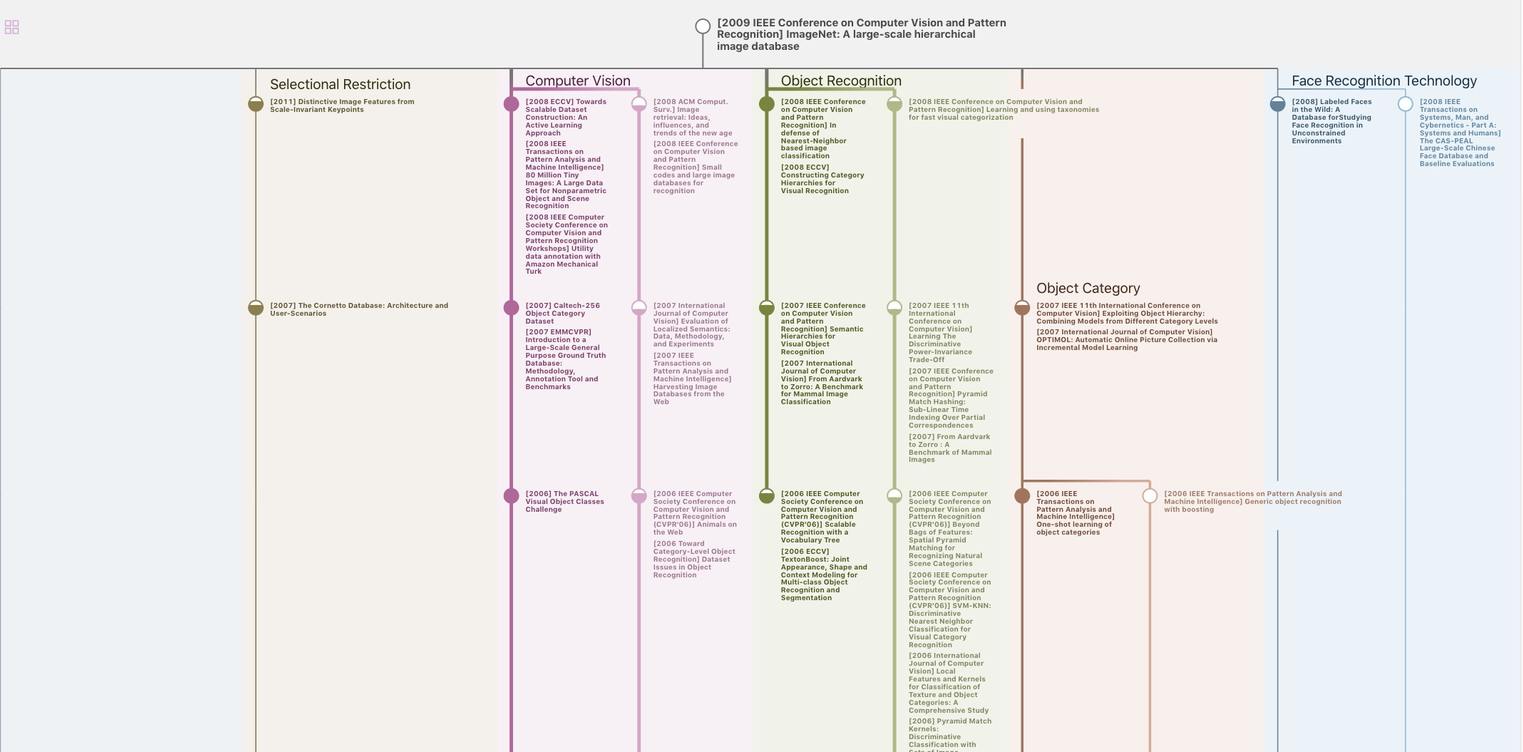
生成溯源树,研究论文发展脉络
Chat Paper
正在生成论文摘要