EW-YOLOv7: A Lightweight and Effective Detection Model for Small Defects in Electrowetting Display
Processes(2023)
摘要
In order to overcome the shortcomings of existing electrowetting display defect detection models in terms of computational complexity, structural complexity, detection speed, and detection accuracy, this article proposes an improved YOLOv7-based electrowetting display defect detection model. The model effectively optimizes the detection performance of display defects, especially small target defects, by integrating GhostNetV2 modules, Acmix attention mechanisms, and NGWD (Normalized Gaussian Wasserstein Distance) Loss. At the same time, it reduces the parameter size of the network model and improves the inference efficiency of the network. This article evaluates the performance of an improved model using a self-constructed electrowetting display defect dataset. The experimental results show that the proposed improved model achieves an average detection rate (mAP) of 89.5% and an average inference time of 35.9 ms. Compared to the original network, the number of parameters and computational costs are reduced by 19.2% and 64.3%, respectively. Compared with current state-of-the-art detection network models, the proposed EW-YOLOv7 exhibits superior performance in detecting electrowetting display defects. This model helps to solve the problem of defect detection in industrial production of electrowetting display and assists the research team in quickly identifying the causes and locations of defects.
更多查看译文
关键词
electrowetting display,small defects,detection,ew-yolov
AI 理解论文
溯源树
样例
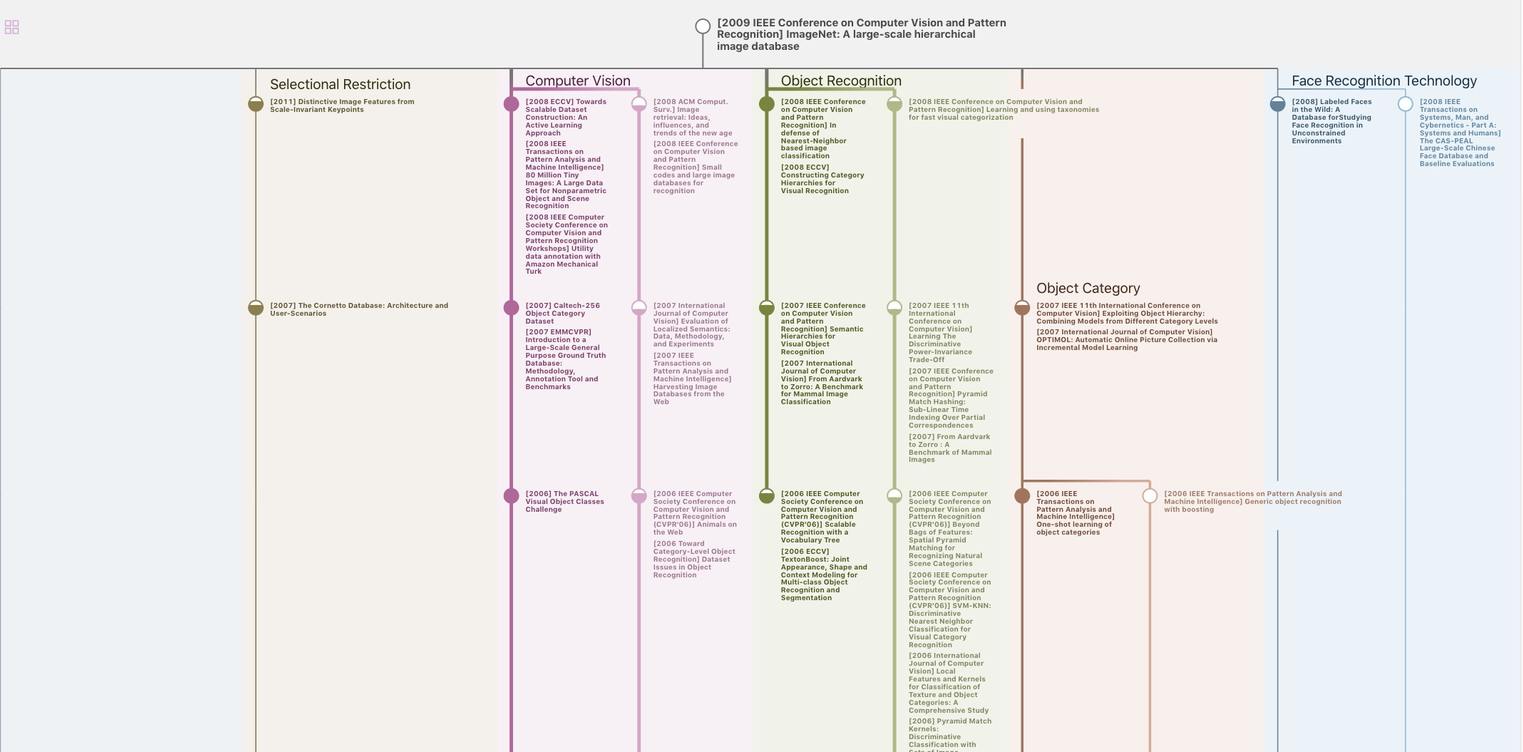
生成溯源树,研究论文发展脉络
Chat Paper
正在生成论文摘要