Synthetic computed tomography generation from multi-sequence magnetic resonance images for nasopharyngeal carcinoma treatment planning via cycle-consistent generative adversarial network
Research Square (Research Square)(2023)
摘要
Abstract Background and purpose: The reliability of deep-learning-based methods for synthetic CT (SCT) generation depends on magnetic resonance (MR)-CT registration errors and the different anatomical information and contract divergence of various tissues in different sequences MRI. This study aimed at establishing CycleGAN models of different single-sequence MRI images and multi-sequence MRI images and investigating the effect of different MRI sequences in the patients with complex nasopharyngealcarcinoma. Materials and methods: Three sequences of MR and planning CT (PCT) images of 151 patients with nasopharyngeal carcinoma were acquired in the radiation treatment position. The number of training, verification, and test sets was 108, 16, and 27, respectively. Four unsupervised CycleGAN-based models were trained using different sequences (single channels) and combinations (multiple channels) as inputs. PCT and MRI pairs were used for network training and testing, respectively. The variables were evaluated between the PCTs and SCTs generated from the different models. Results: T2WI CycleGAN model achieved better results than those of T1WIC and T1WI for a single-sequence model. The CycleGAN model with multiple sequences (T1WI+T2WI+T1WIC) as input to generate the SCT achieved a higher accuracy than that of any single MR sequence model. Meanwhile, the average (3%, 3 mm) gamma passing rates were over 99.06%, and the absolute dose discrepancies within the regions of interest were 0.73%±0.73% for the multi-sequence MR model. Conclusion: CycleGAN with multiple MR sequences as the model input showed the best accuracy. The T2WI images are suitable for SCT prediction in clinical scenarios with limited acquisition sequences or times.
更多查看译文
关键词
synthetic computed tomography generation,nasopharyngeal carcinoma treatment planning,computed tomography,generative,multi-sequence,cycle-consistent
AI 理解论文
溯源树
样例
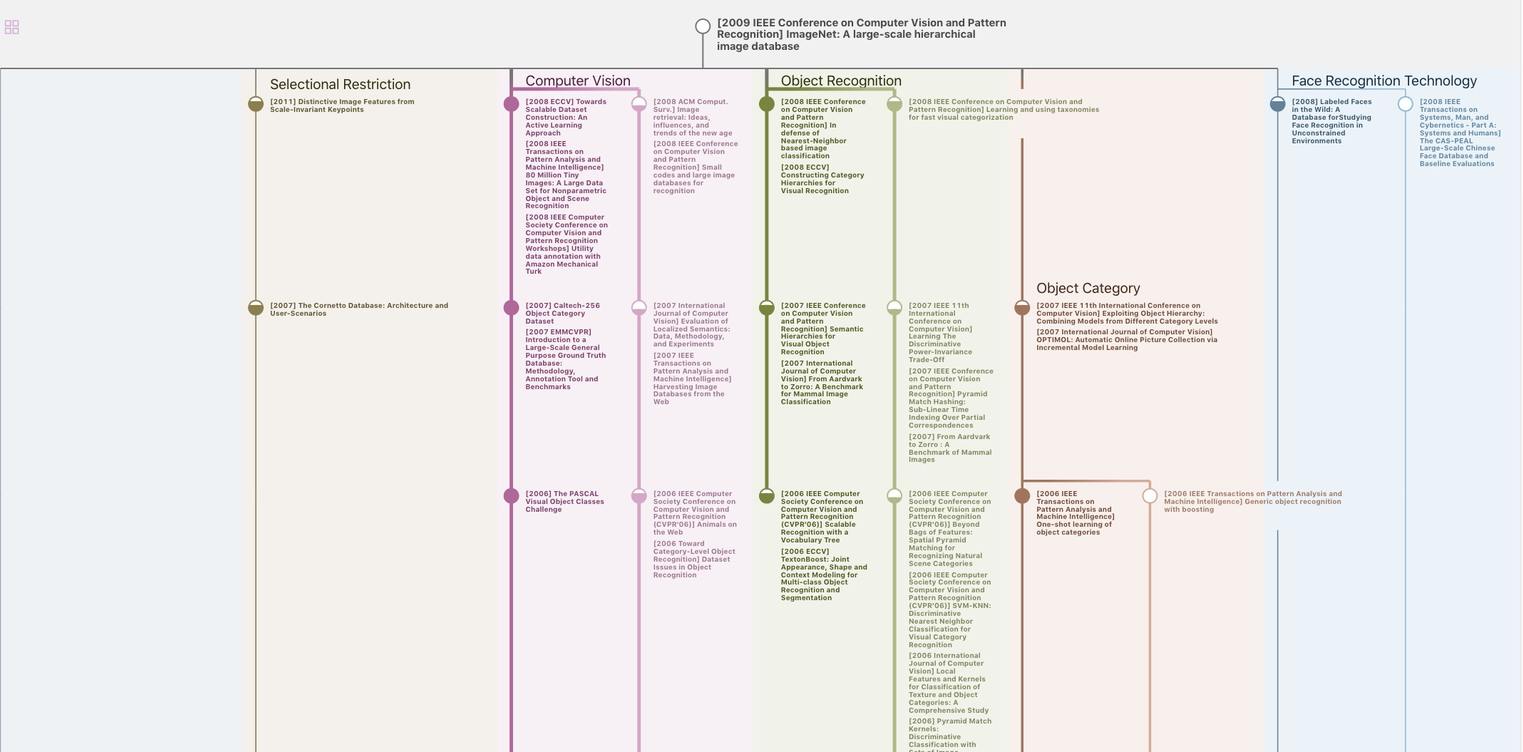
生成溯源树,研究论文发展脉络
Chat Paper
正在生成论文摘要