Machine learning to improve efficiency of non-empirical interaction parameter for dissipative particle dynamics (DPD) simulation
JAPANESE JOURNAL OF APPLIED PHYSICS(2023)
摘要
We have attempted to use machine learning to streamline the calculation of non-empirical parameters for use in dissipative particle dynamics simulations. We replaced the calculation of molecular interaction energies by the non-empirical MO method, which requires a lot of computational resources, with machine learning predictions. We developed two methods for prediction replacement, which are a 1-step method and a 2-step method. The prediction accuracy of the results obtained with these methods was investigated. A reduction of about half of the computational cost was expected.
更多查看译文
关键词
DPD,FMO,machine learning,interaction energy,Chi parameter
AI 理解论文
溯源树
样例
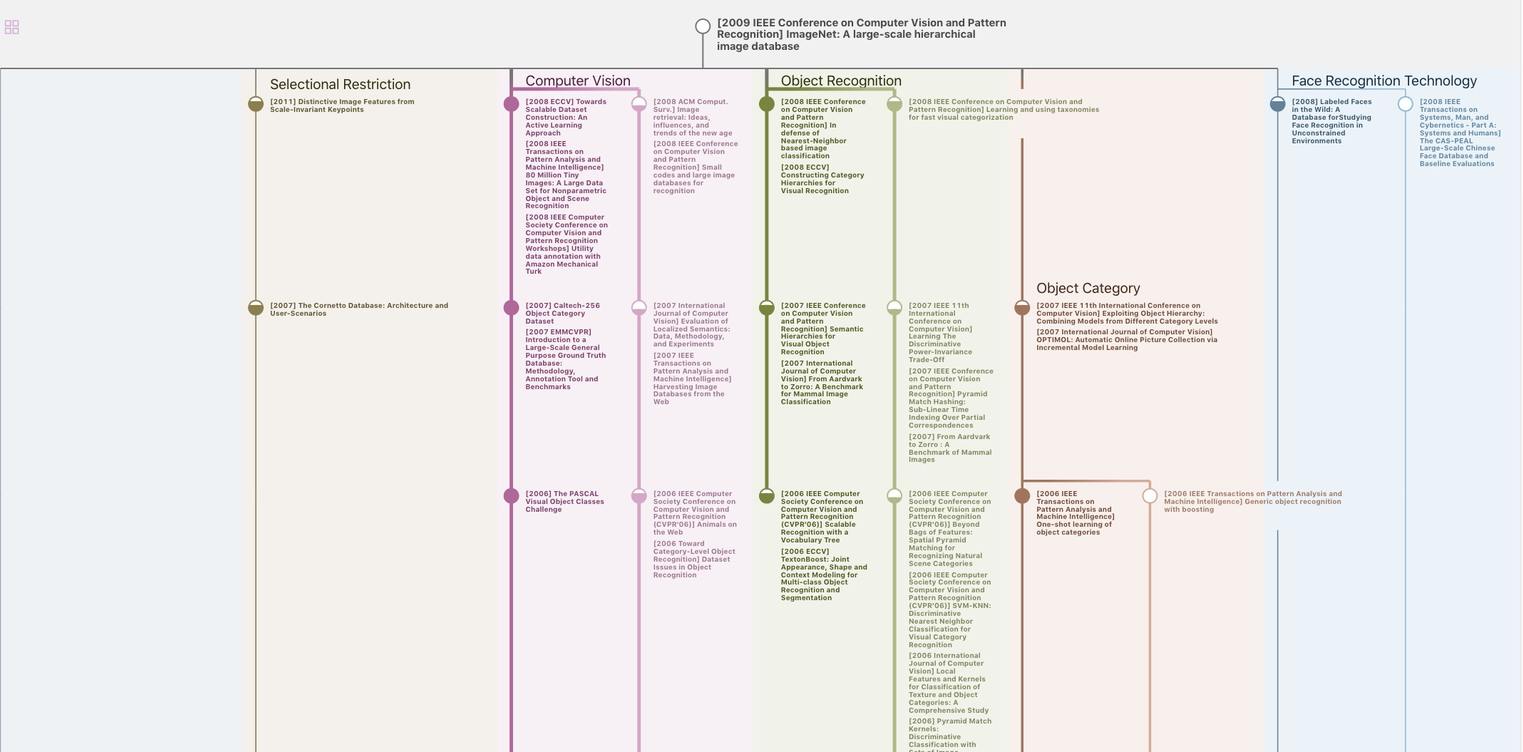
生成溯源树,研究论文发展脉络
Chat Paper
正在生成论文摘要