Invasion depth estimation of carcinoma cells using adaptive stain normalization to improve epidermis segmentation accuracy.
Comput. Medical Imaging Graph.(2023)
摘要
Submucosal invasion depth is a significant prognostic factor when assessing lymph node metastasis and cancer itself to plan proper treatment for the patient. Conventionally, oncologists measure the invasion depth by hand which is a laborious, subjective, and time-consuming process. The manual pathological examination by measuring accurate carcinoma cell invasion with considerable inter-observer and intra-observer variations is still challenging. The increasing use of medical imaging and artificial intelligence reveals a significant role in clinical medicine and pathology. In this paper, we propose an approach to study invasive behavior and measure the invasion depth of carcinoma from stained histopathology images. Specifically, our model includes adaptive stain normalization, color decomposition, and morphological reconstruction with adaptive thresholding to separate the epithelium with blue ratio image. Our method splits the image into multiple non-overlapping meaningful segments and successfully finds the homogeneous segments to measure accurate invasion depth. The invasion depths are measured from the inner epithelium edge to outermost pixels of the deepest part of particles in image. We conduct our experiments on skin melanoma tissue samples as well as on organotypic invasion model utilizing myoma tissue and oral squamous cell carcinoma. The performance is experimentally compared to three closely related reference methods and our method provides a superior result in measuring invasion depth. This computational technique will be beneficial for the segmentation of epithelium and other particles for the development of novel computer-aided diagnostic tools in biobank applications.
更多查看译文
关键词
adaptive stain normalization,invasion depth estimation,carcinoma cells,segmentation
AI 理解论文
溯源树
样例
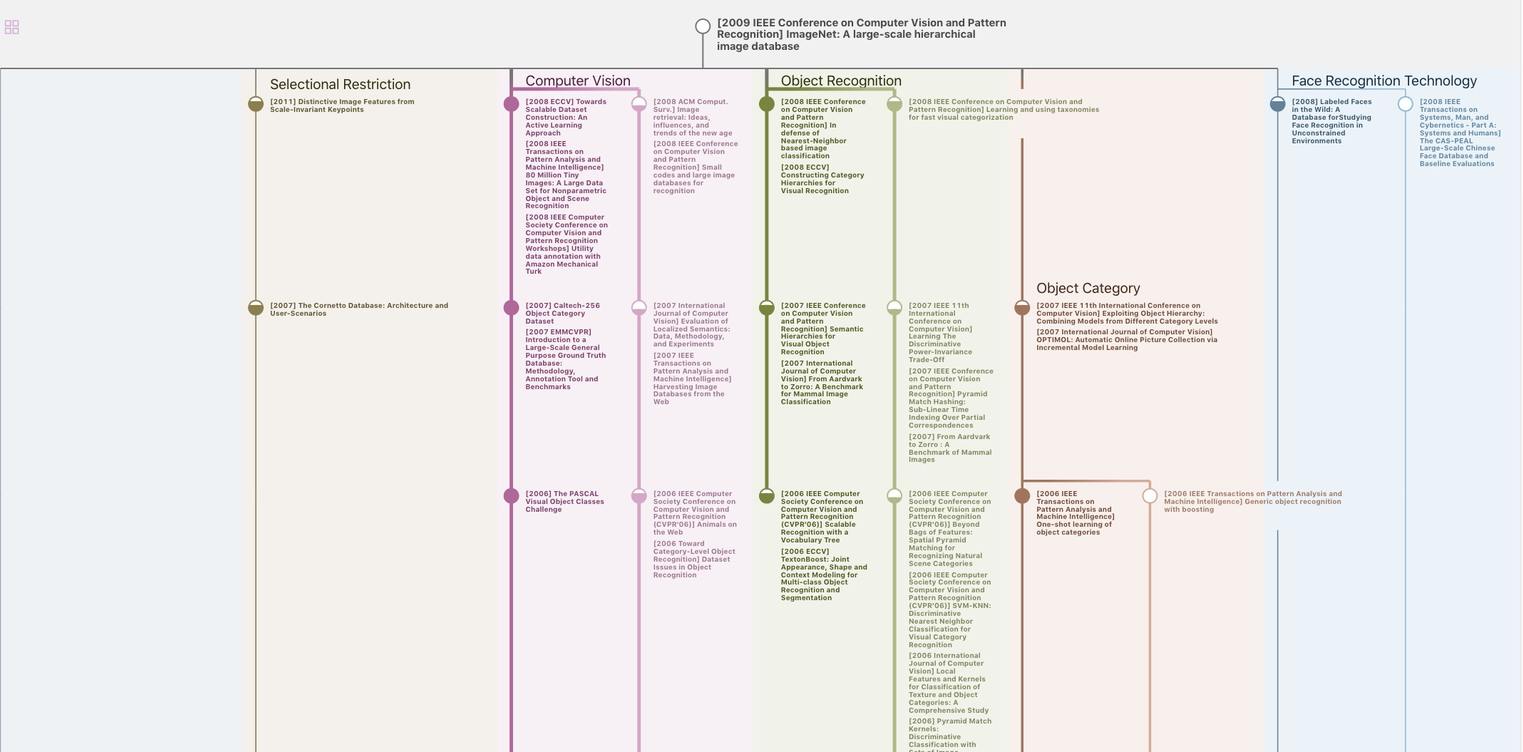
生成溯源树,研究论文发展脉络
Chat Paper
正在生成论文摘要