Human Comprehensible Active Learning of Genome-Scale Metabolic Networks
CoRR(2023)
摘要
An important application of Synthetic Biology is the engineering of the host cell system to yield useful products. However, an increase in the scale of the host system leads to huge design space and requires a large number of validation trials with high experimental costs. A comprehensible machine learning approach that efficiently explores the hypothesis space and guides experimental design is urgently needed for the Design-Build-Test-Learn (DBTL) cycle of the host cell system. We introduce a novel machine learning framework ILP-iML1515 based on Inductive Logic Programming (ILP) that performs abductive logical reasoning and actively learns from training examples. In contrast to numerical models, ILP-iML1515 is built on comprehensible logical representations of a genome-scale metabolic model and can update the model by learning new logical structures from auxotrophic mutant trials. The ILP-iML1515 framework 1) allows high-throughput simulations and 2) actively selects experiments that reduce the experimental cost of learning gene functions in comparison to randomly selected experiments.
更多查看译文
关键词
metabolic,networks,learning,genome-scale
AI 理解论文
溯源树
样例
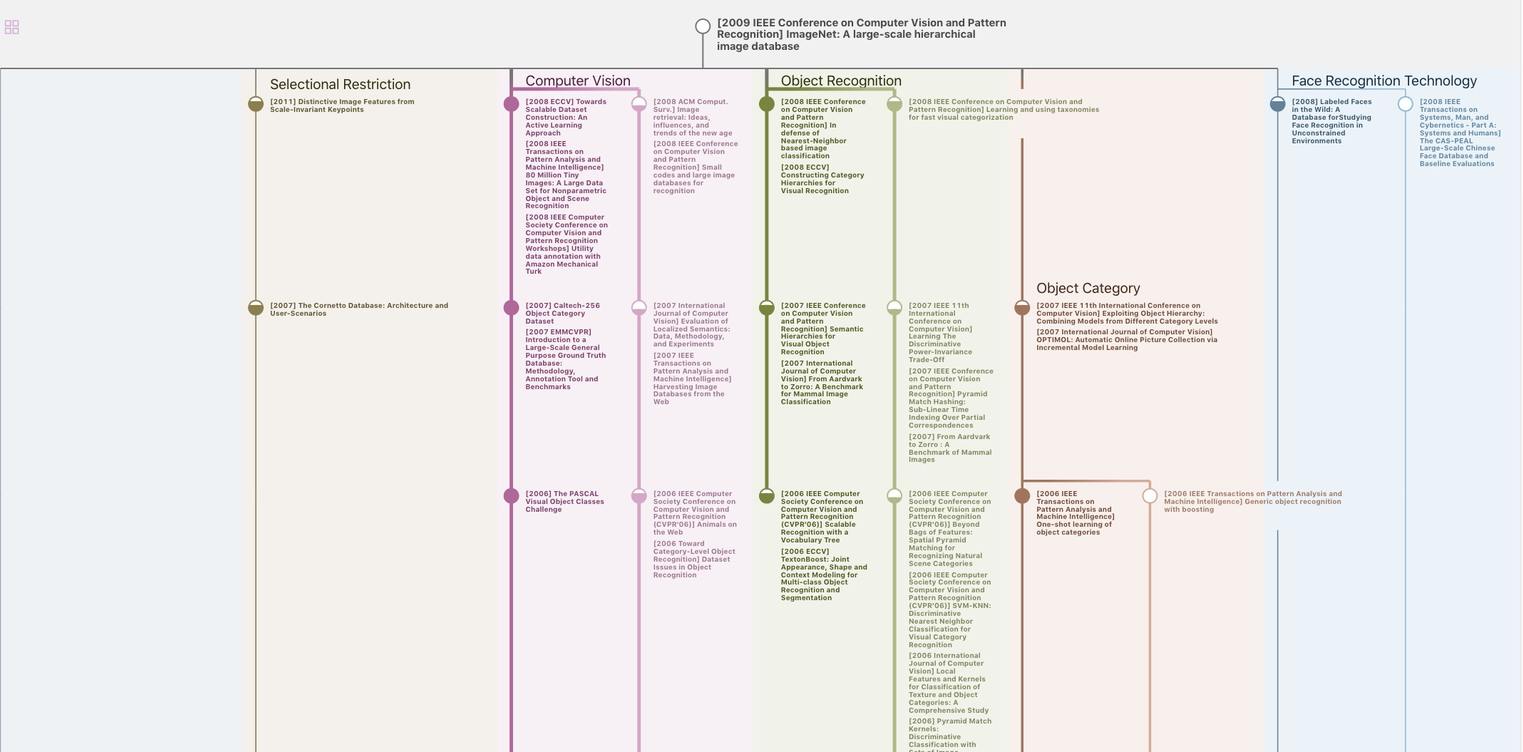
生成溯源树,研究论文发展脉络
Chat Paper
正在生成论文摘要