FFEINR: flow feature-enhanced implicit neural representation for spatiotemporal super-resolution
CoRR(2024)
摘要
Large-scale numerical simulations are capable of generating data up to terabytes or even petabytes. As a promising method of data reduction, super-resolution (SR) has been widely studied in the scientific visualization community. However, most of them are based on deep convolutional neural networks or generative adversarial networks and the scale factor needs to be determined before constructing the network. As a result, a single training session only supports a fixed factor and has poor generalization ability. To address these problems, this paper proposes a flow feature-enhanced implicit neural representation (FFEINR) for spatiotemporal super-resolution of flow field data. It can take full advantage of the implicit neural representation in terms of model structure and sampling resolution. The neural representation is based on a fully connected network with periodic activation functions, which enables us to obtain lightweight models. The learned continuous representation can decode the low-resolution flow field input data to arbitrary spatial and temporal resolutions, allowing for flexible upsampling. The training process of FFEINR is facilitated by introducing feature enhancements for the input layer, which complements the contextual information of the flow field. To demonstrate the effectiveness of the proposed method, a series of experiments are conducted on different datasets by setting different hyperparameters. The results show that FFEINR achieves significantly better results than the trilinear interpolation method. Graphical abstract
更多查看译文
关键词
Implicit neural representation,Spatiotemporal super-resolution,Flow field data
AI 理解论文
溯源树
样例
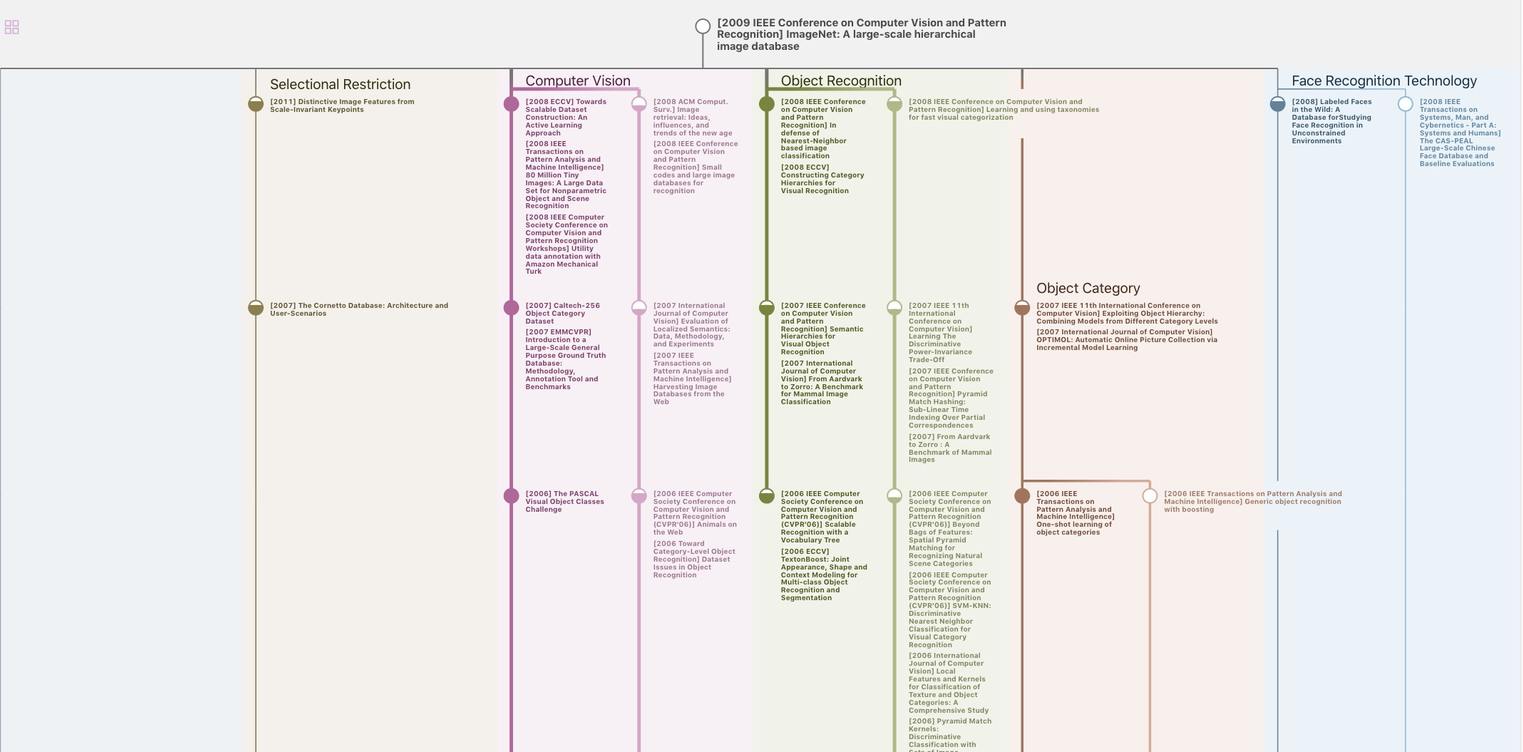
生成溯源树,研究论文发展脉络
Chat Paper
正在生成论文摘要