Leverage classifier: Another look at support vector machine
arXiv (Cornell University)(2023)
摘要
Support vector machine (SVM) is a popular classifier known for accuracy, flexibility, and robustness. However, its intensive computation has hindered its application to large-scale datasets. In this paper, we propose a new optimal leverage classifier based on linear SVM under a nonseparable setting. Our classifier aims to select an informative subset of the training sample to reduce data size, enabling efficient computation while maintaining high accuracy. We take a novel view of SVM under the general subsampling framework and rigorously investigate the statistical properties. We propose a two-step subsampling procedure consisting of a pilot estimation of the optimal subsampling probabilities and a subsampling step to construct the classifier. We develop a new Bahadur representation of the SVM coefficients and derive unconditional asymptotic distribution and optimal subsampling probabilities without giving the full sample. Numerical results demonstrate that our classifiers outperform the existing methods in terms of estimation, computation, and prediction.
更多查看译文
关键词
vector,machine
AI 理解论文
溯源树
样例
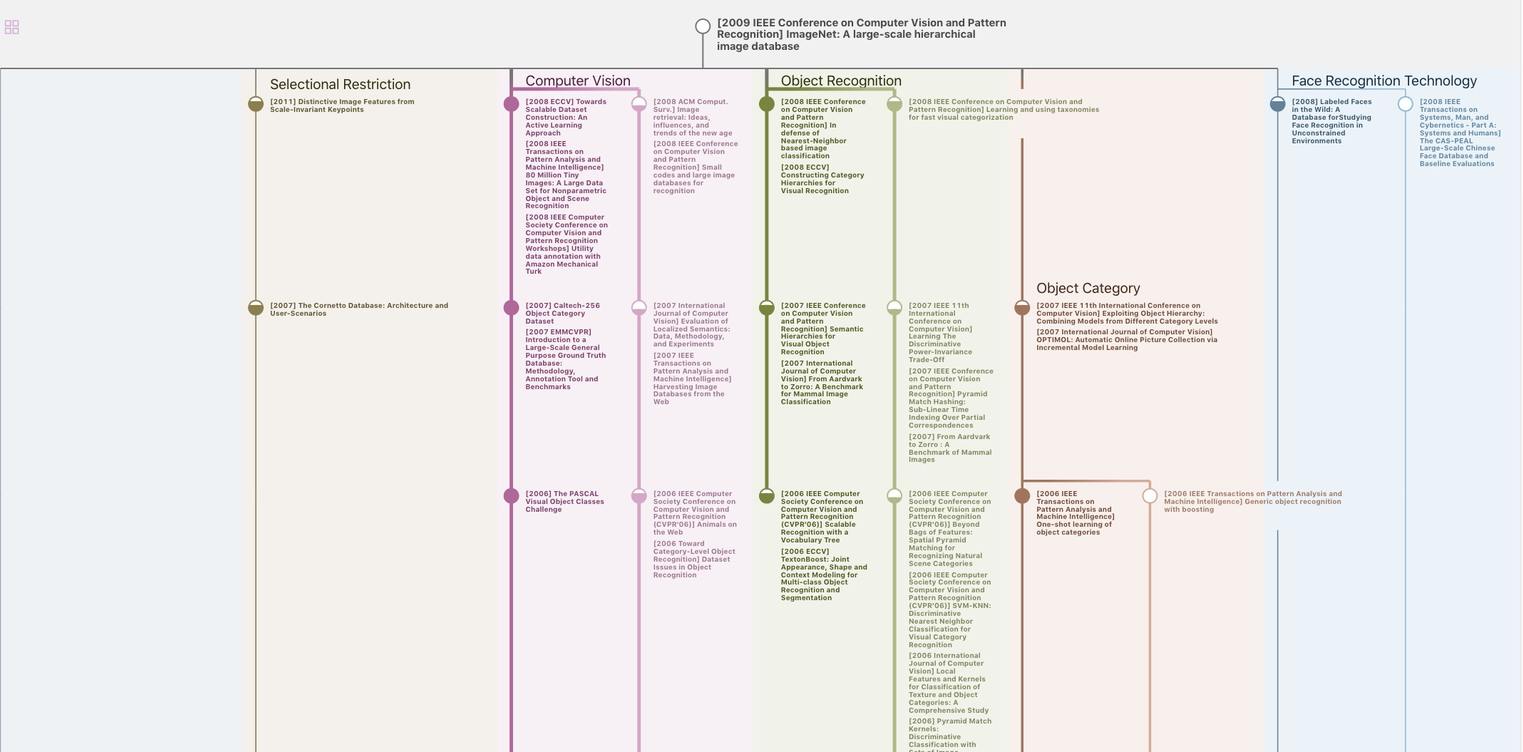
生成溯源树,研究论文发展脉络
Chat Paper
正在生成论文摘要