Applicability Area: A novel utility-based approach for evaluating predictive models, beyond discrimination.
medRxiv (Cold Spring Harbor Laboratory)(2024)
摘要
Translating prediction models into practice and supporting clinicians' decision-making demand demonstration of clinical value. Existing approaches to evaluating machine learning models emphasize discriminatory power, which is only a part of the medical decision problem. We propose the Applicability Area (ApAr), a decision-analytic utility-based approach to evaluating predictive models that communicate the range of prior probability and test cutoffs for which the model has positive utility; larger ApArs suggest a broader potential use of the model. We assess ApAr with simulated datasets and with three published medical datasets. ApAr adds value beyond the typical area under the receiver operating characteristic curve (AUROC) metric analysis. As an example, in the diabetes dataset, the top model by ApAr was ranked as the 23rd best model by AUROC. Decision makers looking to adopt and implement models can leverage ApArs to assess if the local range of priors and utilities is within the respective ApArs.
更多查看译文
关键词
predictive models,applicability,utility-based
AI 理解论文
溯源树
样例
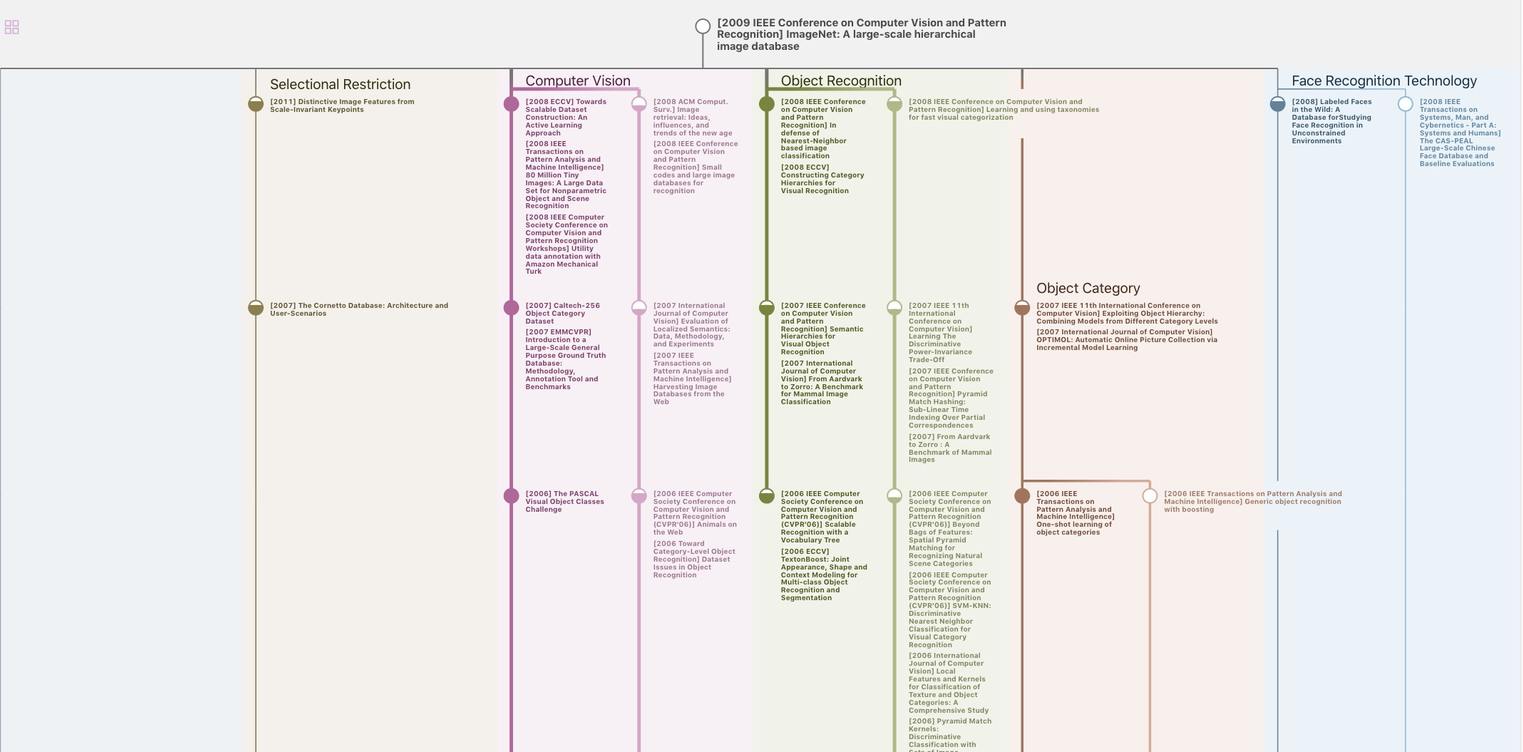
生成溯源树,研究论文发展脉络
Chat Paper
正在生成论文摘要