AI-enhanced integration of genetic and medical imaging data for risk assessment of Type 2 diabetes.
Nature communications(2024)
摘要
Type 2 diabetes (T2D) presents a formidable global health challenge, highlighted by its escalating prevalence, underscoring the critical need for precision health strategies and early detection initiatives. Leveraging artificial intelligence, particularly eXtreme Gradient Boosting (XGBoost), we devise robust risk assessment models for T2D. Drawing upon comprehensive genetic and medical imaging datasets from 68,911 individuals in the Taiwan Biobank, our models integrate Polygenic Risk Scores (PRS), Multi-image Risk Scores (MRS), and demographic variables, such as age, sex, and T2D family history. Here, we show that our model achieves an Area Under the Receiver Operating Curve (AUC) of 0.94, effectively identifying high-risk T2D subgroups. A streamlined model featuring eight key variables also maintains a high AUC of 0.939. This high accuracy for T2D risk assessment promises to catalyze early detection and preventive strategies. Moreover, we introduce an accessible online risk assessment tool for T2D, facilitating broader applicability and dissemination of our findings.
更多查看译文
关键词
diabetes,medical imaging data,genetic,risk assessment,ai-enhanced
AI 理解论文
溯源树
样例
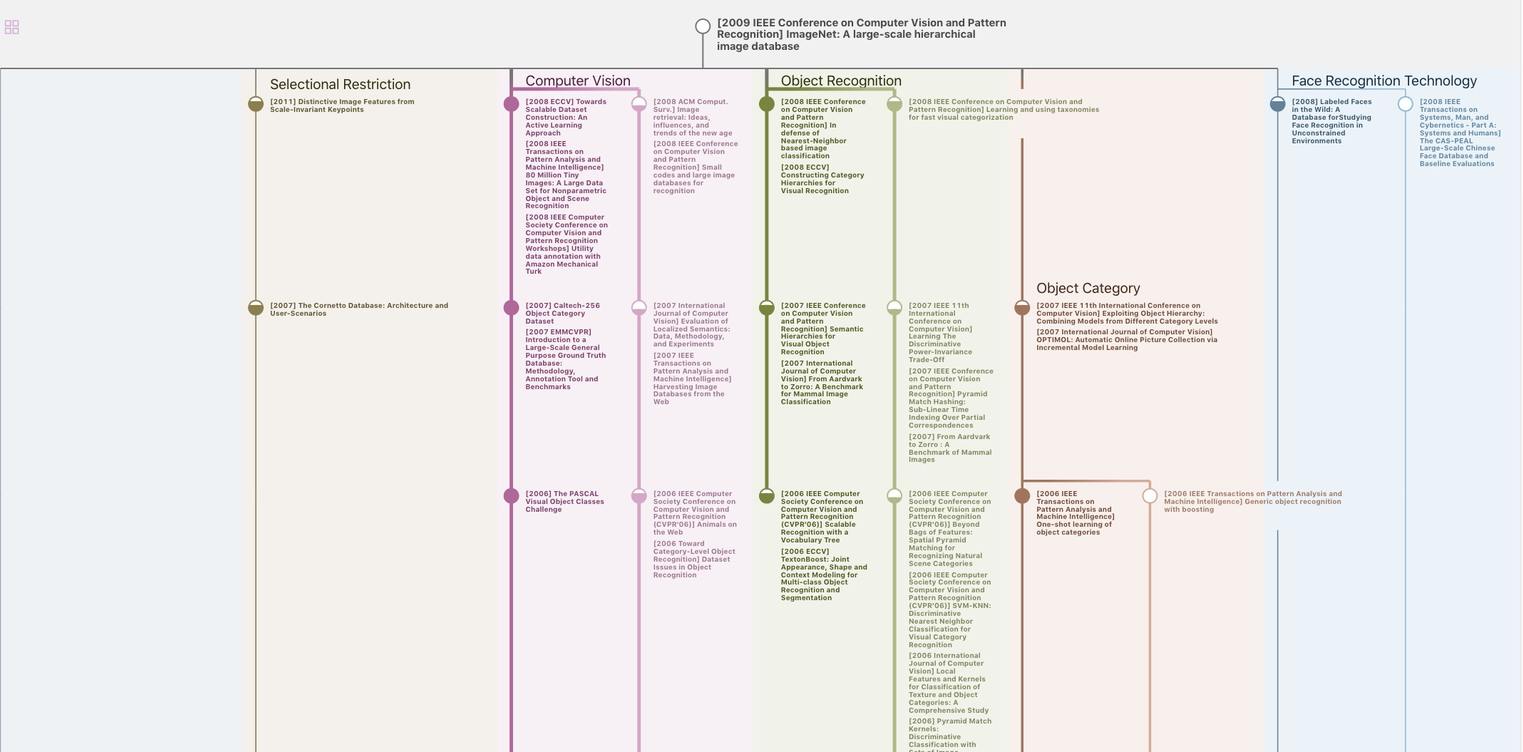
生成溯源树,研究论文发展脉络
Chat Paper
正在生成论文摘要