Masking Strategies for Background Bias Removal in Computer Vision Models
2023 IEEE/CVF INTERNATIONAL CONFERENCE ON COMPUTER VISION WORKSHOPS, ICCVW(2023)
摘要
Models for fine-grained image classification tasks, where the difference between some classes can be extremely subtle and the number of samples per class tends to be low, are particularly prone to picking up background-related biases and demand robust methods to handle potential examples with out-of-distribution (OOD) backgrounds. To gain deeper insights into this critical problem, our research investigates the impact of background-induced bias on finegrained image classification, evaluating standard backbone models such as Convolutional Neural Network (CNN) and Vision Transformers (ViT). We explore two masking strategies to mitigate background-induced bias: Early masking, which removes background information at the (input) image level, and late masking, which selectively masks high-level spatial features corresponding to the background. Extensive experiments assess the behavior of CNN and ViT models under different masking strategies, with a focus on their generalization to OOD backgrounds. The obtained findings demonstrate that both proposed strategies enhance OOD performance compared to the baseline models, with early masking consistently exhibiting the best OOD performance. Notably, a ViT variant employing GAP-Pooled Patch tokenbased classification combined with early masking achieves the highest OOD robustness. Our code and models are available at: GitHub
更多查看译文
关键词
background bias removal,masking,vision
AI 理解论文
溯源树
样例
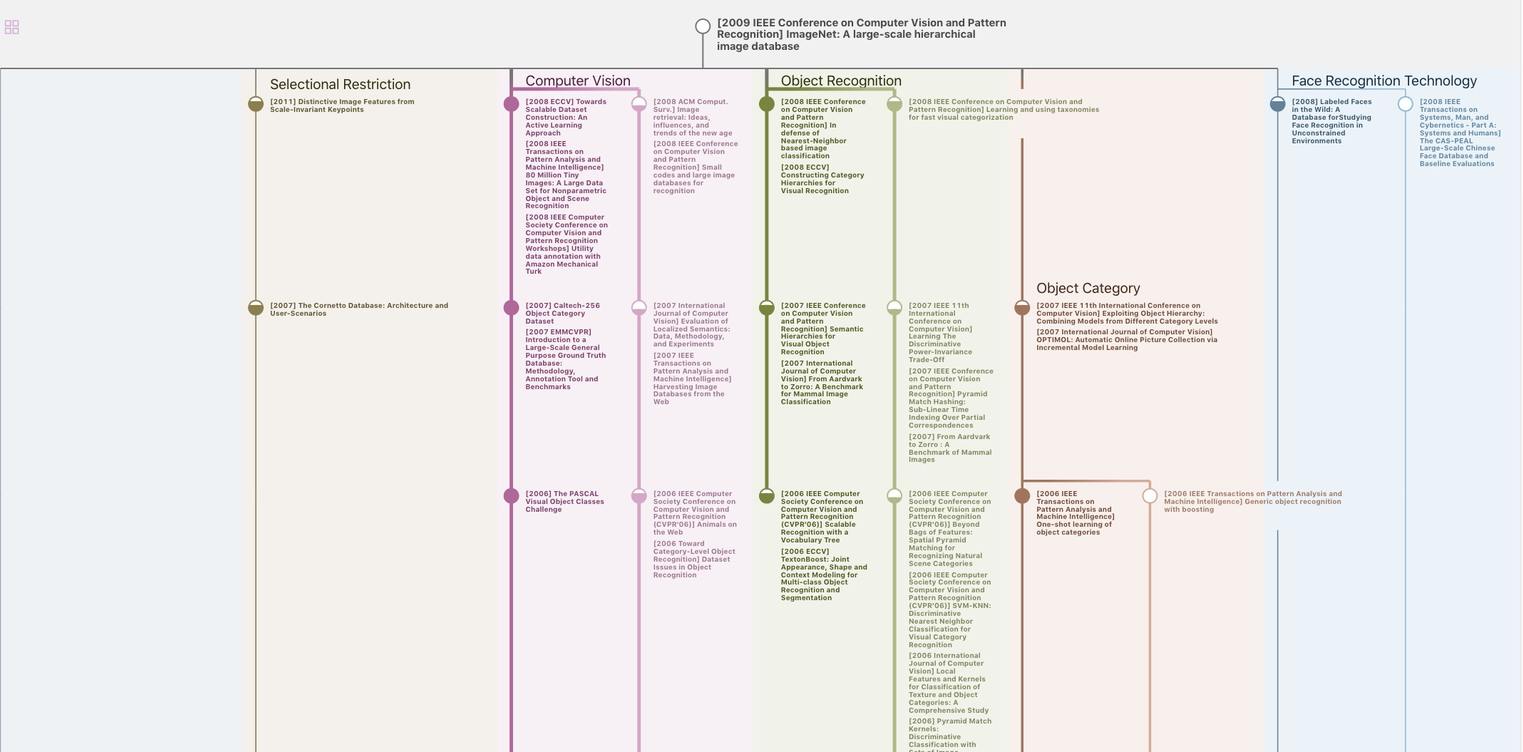
生成溯源树,研究论文发展脉络
Chat Paper
正在生成论文摘要