Approximating Score-based Explanation Techniques Using Conformal Regression
CoRR(2023)
摘要
Score-based explainable machine-learning techniques are often used to understand the logic behind black-box models. However, such explanation techniques are often computationally expensive, which limits their application in time-critical contexts. Therefore, we propose and investigate the use of computationally less costly regression models for approximating the output of score-based explanation techniques, such as SHAP. Moreover, validity guarantees for the approximated values are provided by the employed inductive conformal prediction framework. We propose several non-conformity measures designed to take the difficulty of approximating the explanations into account while keeping the computational cost low. We present results from a large-scale empirical investigation, in which the approximate explanations generated by our proposed models are evaluated with respect to efficiency (interval size). The results indicate that the proposed method can significantly improve execution time compared to the fast version of SHAP, TreeSHAP. The results also suggest that the proposed method can produce tight intervals, while providing validity guarantees. Moreover, the proposed approach allows for comparing explanations of different approximation methods and selecting a method based on how informative (tight) are the predicted intervals.
更多查看译文
关键词
explanation techniques,regression,conformal
AI 理解论文
溯源树
样例
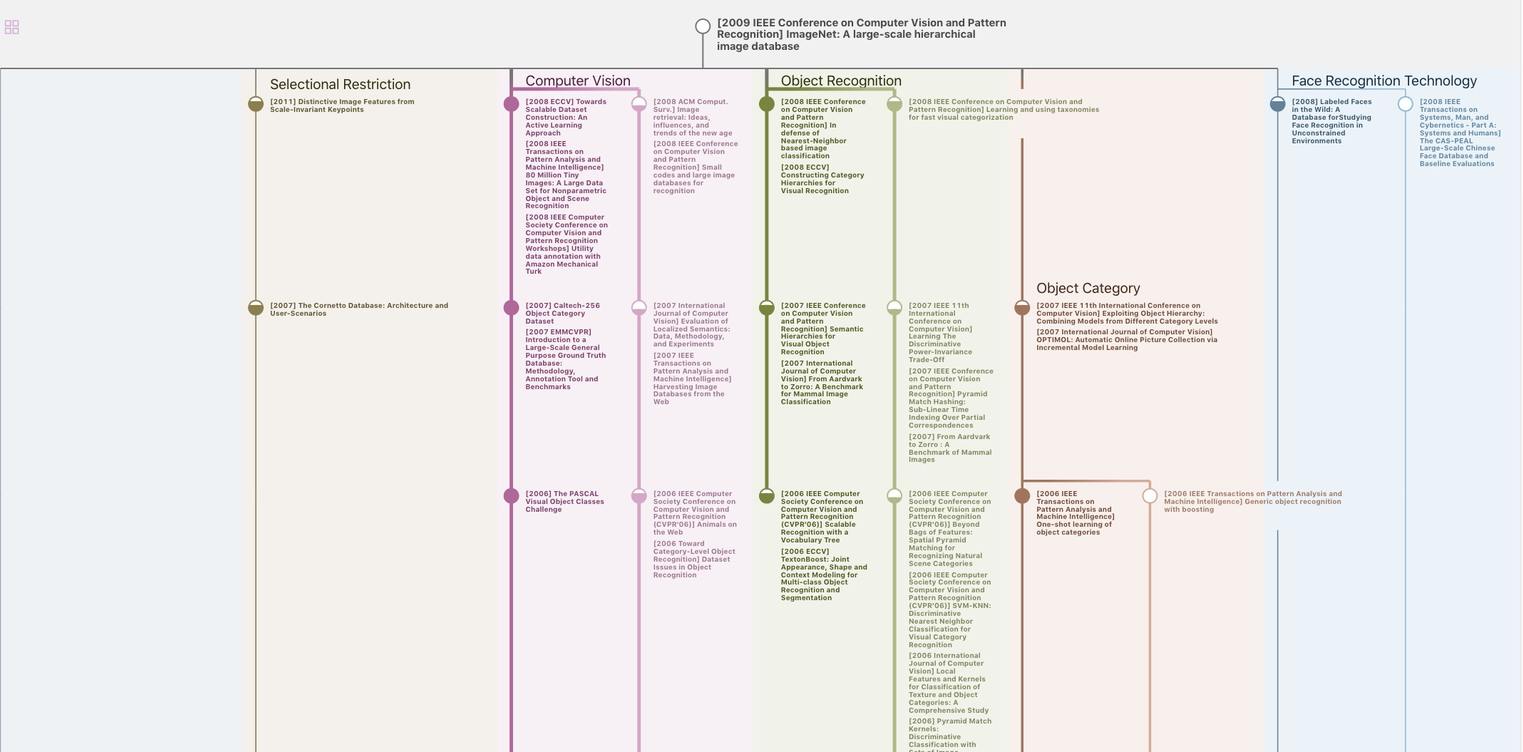
生成溯源树,研究论文发展脉络
Chat Paper
正在生成论文摘要