Audio Generation with Multiple Conditional Diffusion Model
AAAI 2024(2024)
摘要
Text-based audio generation models have limitations as they cannot encompass all the information in audio, leading to restricted controllability when relying solely on text. To address this issue, we propose a novel model that enhances the controllability of existing pre-trained text-to-audio models by incorporating additional conditions including content (timestamp) and style (pitch contour and energy contour) as supplements to the text. This approach achieves fine-grained control over the temporal order, pitch, and energy of generated audio. To preserve the diversity of generation, we employ a trainable control condition encoder that is enhanced by a large language model and a trainable Fusion-Net to encode and fuse the additional conditions while keeping the weights of the pre-trained text-to-audio model frozen. Due to the lack of suitable datasets and evaluation metrics, we consolidate existing datasets into a new dataset comprising the audio and corresponding conditions and use a series of evaluation metrics to evaluate the controllability performance. Experimental results demonstrate that our model successfully achieves fine-grained control to accomplish controllable audio generation.
更多查看译文
关键词
NLP: Language Grounding & Multi-modal NLP,NLP: Generation
AI 理解论文
溯源树
样例
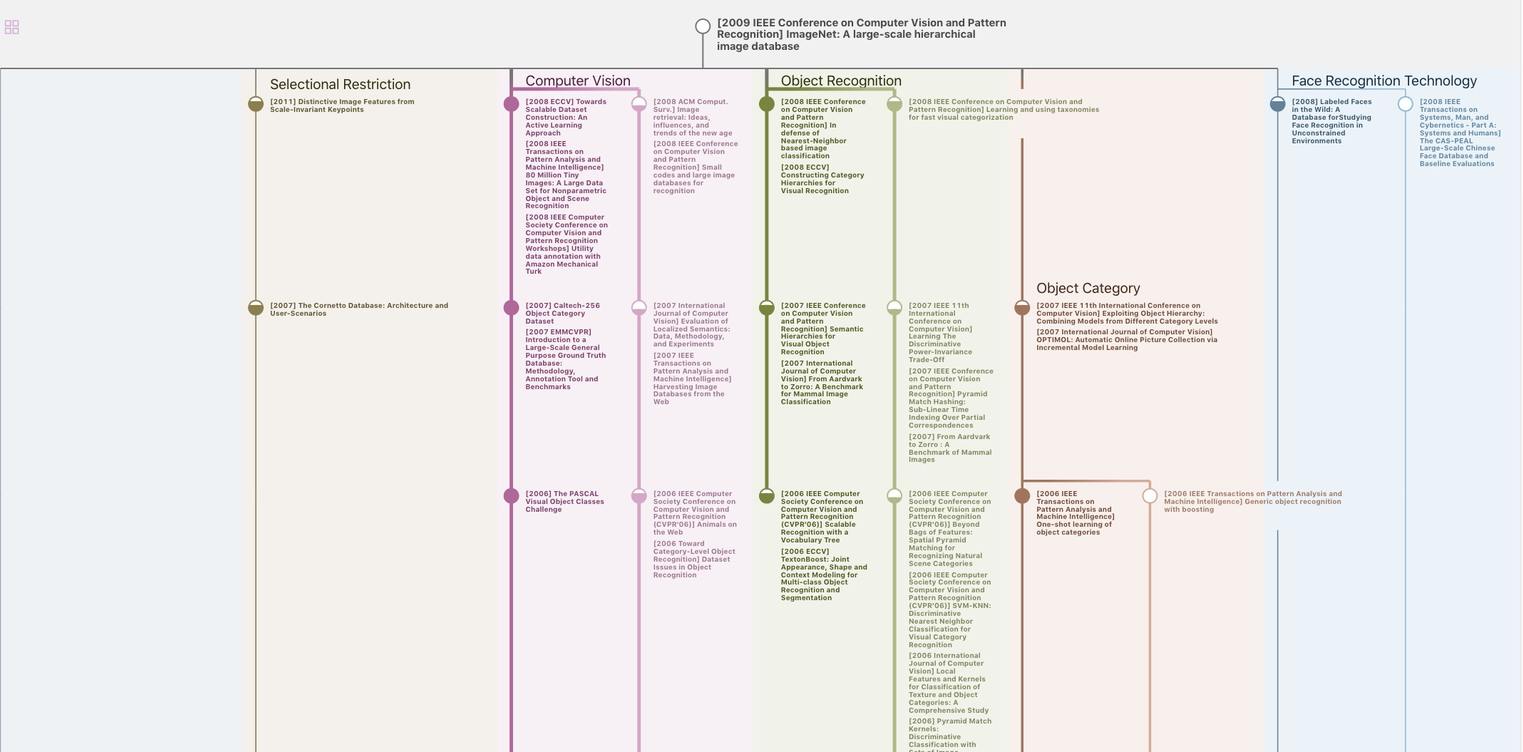
生成溯源树,研究论文发展脉络
Chat Paper
正在生成论文摘要