Defect graph neural networks for materials discovery in high-temperature clean-energy applications
Nature Computational Science(2023)
摘要
We present a graph neural network approach that fully automates the prediction of defect formation enthalpies for any crystallographic site from the ideal crystal structure, without the need to create defected atomic structure models as input. Here we used density functional theory reference data for vacancy defects in oxides, to train a defect graph neural network (dGNN) model that replaces the density functional theory supercell relaxations otherwise required for each symmetrically unique crystal site. Interfaced with thermodynamic calculations of reduction entropies and associated free energies, the dGNN model is applied to the screening of oxides in the Materials Project database, connecting the zero-kelvin defect enthalpies to high-temperature process conditions relevant for solar thermochemical hydrogen production and other energy applications. The dGNN approach is applicable to arbitrary structures with an accuracy limited principally by the amount and diversity of the training data, and it is generalizable to other defect types and advanced graph convolution architectures. It will help to tackle future materials discovery problems in clean energy and beyond.
更多查看译文
关键词
materials discovery,neural networks,graph,high-temperature,clean-energy
AI 理解论文
溯源树
样例
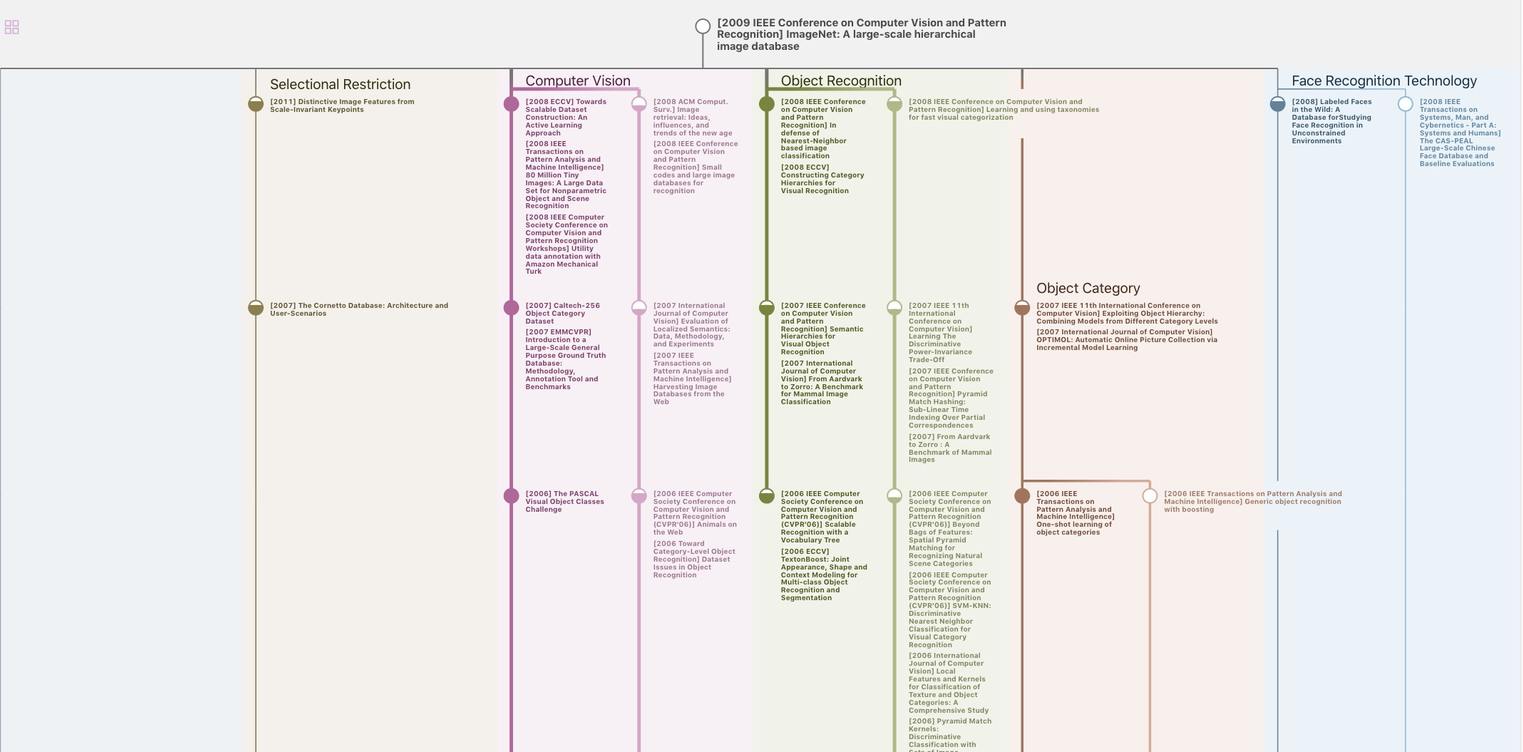
生成溯源树,研究论文发展脉络
Chat Paper
正在生成论文摘要