BLPSeg: Balance the Label Preference in Scribble-Supervised Semantic Segmentation.
IEEE transactions on image processing : a publication of the IEEE Signal Processing Society(2023)
摘要
Scribble-supervised semantic segmentation is an appealing weakly supervised technique with low labeling cost. Existing approaches mainly consider diffusing the labeled region of scribble by low-level feature similarity to narrow the supervision gap between scribble labels and mask labels. In this study, we observe an annotation bias between scribble and object mask, i.e., label workers tend to scribble on the spacious region instead of corners. This label preference makes the model learn well on those frequently labeled regions but poor on rarely labeled pixels. Therefore, we propose BLPSeg to balance the label preference for complete segmentation. Specifically, the BLPSeg first predicts an annotation probability map to evaluate the rarity of labels on each image, then utilizes a novel BLP loss to balance the model training by up-weighting those rare annotations. Additionally, to further alleviate the impact of label preference, we design a local aggregation module (LAM) to propagate supervision from labeled to unlabeled regions in gradient backpropagation. We conduct extensive experiments to illustrate the effectiveness of our BLPSeg. Our single-stage method even outperforms other advanced multi-stage methods and achieves state-of-the-art performance.
更多查看译文
关键词
Annotations, Semantic segmentation, Training, Task analysis, Head, Semantics, Costs, Scribble-supervised, weakly supervised, semantic segmentation
AI 理解论文
溯源树
样例
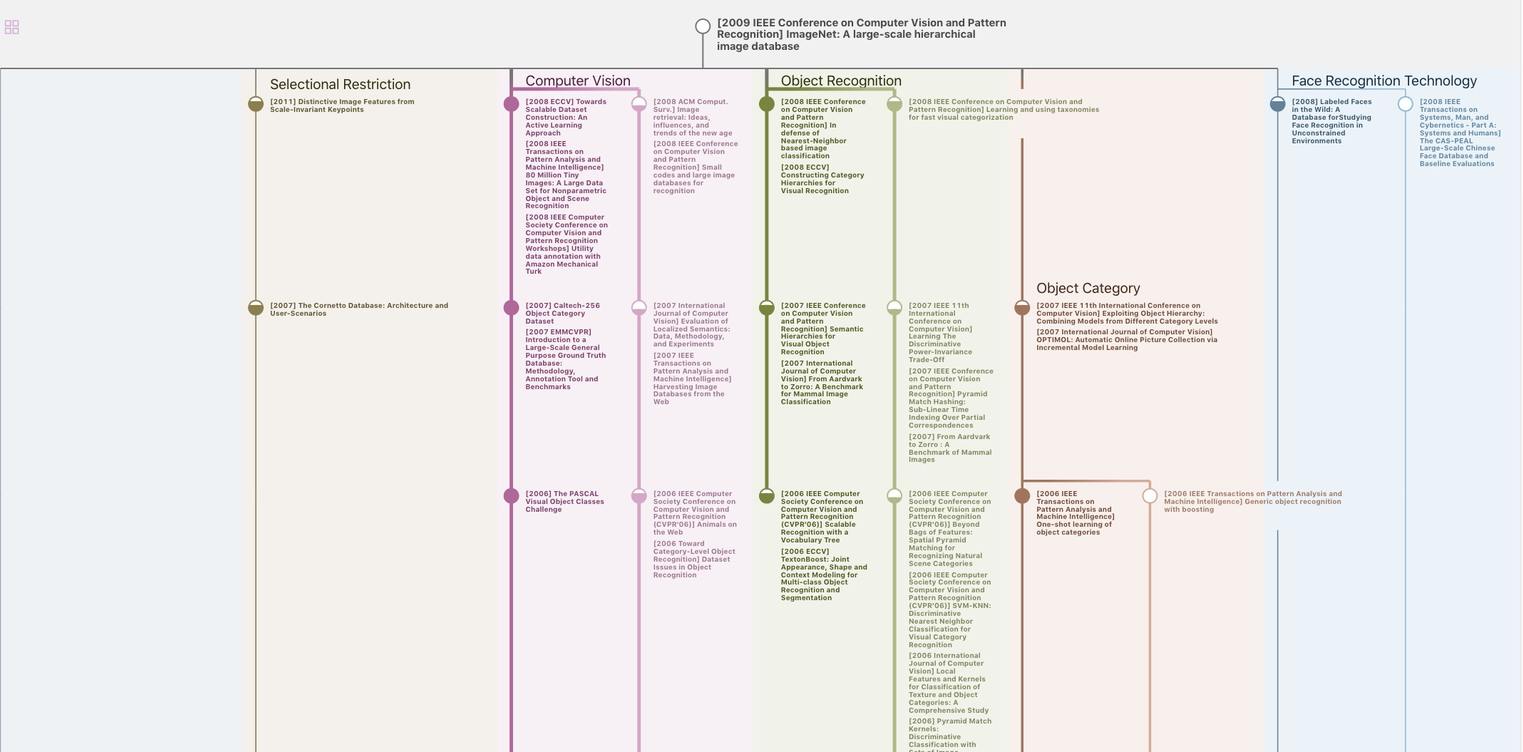
生成溯源树,研究论文发展脉络
Chat Paper
正在生成论文摘要