Unsupervised Prototype Adapter for Vision-Language Models
PATTERN RECOGNITION AND COMPUTER VISION, PRCV 2023, PT I(2024)
摘要
Recently, large-scale pre-trained vision-language models (e.g. CLIP and ALIGN) have demonstrated remarkable effectiveness in acquiring transferable visual representations. To leverage the valuable knowledge encoded within these models for downstream tasks, several fine-tuning approaches, including prompt tuning methods and adapter-based methods, have been developed to adapt vision-language models effectively with supervision. However, these methods rely on the availability of annotated samples, which can be labor-intensive and time-consuming to acquire, thus limiting scalability. To address this issue, in this work, we design an unsupervised fine-tuning approach for vision-language models called Unsupervised Prototype Adapter (UP-Adapter). Specifically, for the unannotated target datasets, we leverage the text-image aligning capability of CLIP to automatically select the most confident samples for each class. Utilizing these selected samples, we generate class prototypes, which serve as the initialization for the learnable prototype model. After fine-tuning, the prototype model prediction is combined with the original CLIP's prediction by a residual connection to perform downstream recognition tasks. Our extensive experimental results on image recognition and domain generalization show that the proposed unsupervised method outperforms 8-shot CoOp, 8-shot Tip-Adapter, and also the state-of-the-art UPL method by large margins.
更多查看译文
关键词
Vision-Language Models,Contrastive Language-Image Pre-training,Unsupervised Learning,Image Recognition
AI 理解论文
溯源树
样例
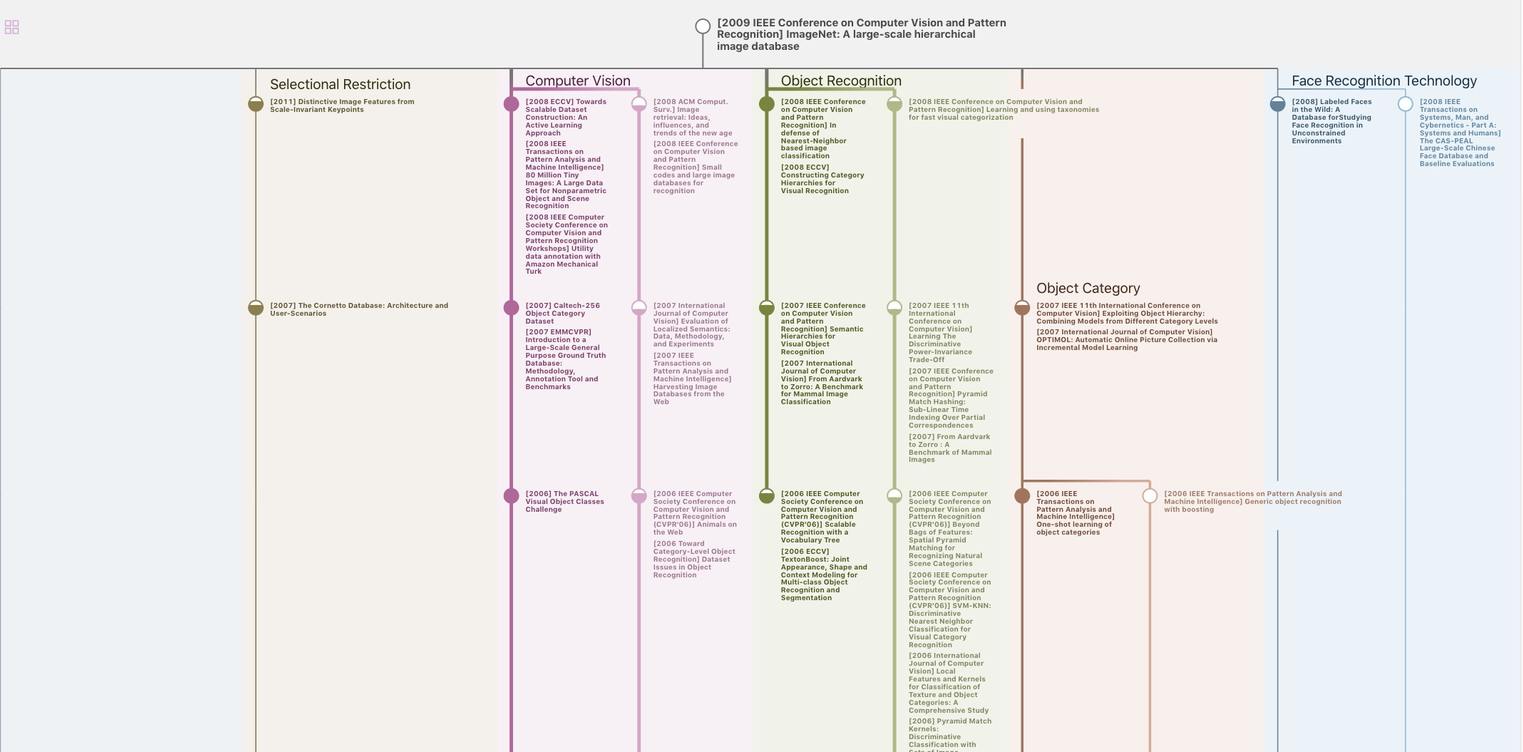
生成溯源树,研究论文发展脉络
Chat Paper
正在生成论文摘要