Machine learning models in predicting health care costs in patients with a recent acute coronary syndrome: A prospective pilot study
Cardiovascular digital health journal(2023)
摘要
BACKGROUND Health care budgets are limited, requiring the optimal use of resources. Machine learning (ML) methods may have an enormous potential for effective use of health care re-sources. OBJECTIVE We assessed the applicability of selected ML tools to evaluate the contribution of known risk markers for prognosis of coronary artery disease to predict health care costs for all reasons in patients with a recent acute coronary syndrome (n = 65, aged 65 & PLUSMN; 9 years) for 1-year follow-up.METHODS Risk markers were assessed at baseline, and health care costs were collected from electronic health registries. The Cross -decomposition algorithms were used to rank the considered risk markers based on their impacts on variances. Then regression anal-ysis was performed to predict costs by entering the first top-ranking risk marker and adding the next-best markers, one by one, to build up altogether 13 predictive models.RESULTS The average annual health care costs were euro2601 & PLUSMN; euro5378 per patient. The Depression Scale showed the highest pre-dictive value (r = 0.395), accounting for 16% of the costs (P = .001). When the next 2 ranked markers (LDL cholesterol, r = 0.230; and left ventricular ejection fraction, r = -0.227, respec-tively) were added to the model, the predictive value was 24% for the costs (P = .001).CONCLUSION Higher depression score is the primary variable fore-casting health care costs in 1-year follow-up among acute coronary syndrome patients. The ML tools may help decision-making when planning optimal utilization of treatment strategies.
更多查看译文
关键词
Coronary artery disease,Coronary heart disease,Artifi-cial intelligence,Health care costs,Economic evaluation
AI 理解论文
溯源树
样例
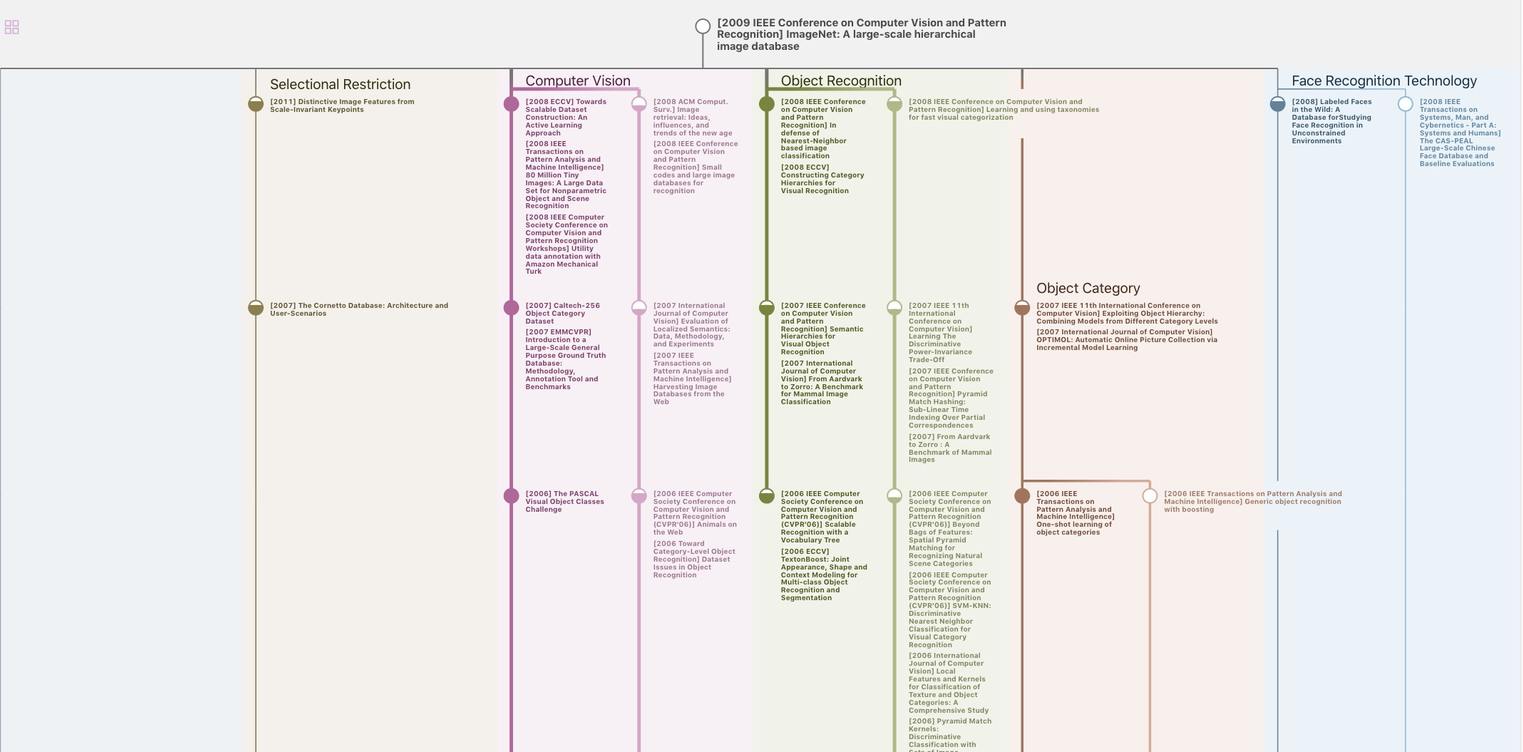
生成溯源树,研究论文发展脉络
Chat Paper
正在生成论文摘要