SeqGPT: An Out-of-the-Box Large Language Model for Open Domain Sequence Understanding
AAAI 2024(2024)
摘要
Large language models (LLMs) have shown impressive abilities for open-domain NLP tasks. However, LLMs are sometimes too footloose for natural language understanding (NLU) tasks which always have restricted output and input format. Their performances on NLU tasks are highly related to prompts or demonstrations and are shown to be poor at performing several representative NLU tasks, such as event extraction and entity typing. To this end, we present SeqGPT, a bilingual (i.e., English and Chinese) open-source autoregressive model specially enhanced for open-domain natural language understanding. We express all NLU tasks with two atomic tasks, which define fixed instructions to restrict the input and output format but still ``open'' for arbitrarily varied label sets. The model is first instruction-tuned with extremely fine-grained labeled data synthesized by ChatGPT and then further fine-tuned by 233 different atomic tasks from 152 datasets across various domains. The experimental results show that SeqGPT has decent classification and extraction ability, and is capable of performing language understanding tasks on unseen domains. We also conduct empirical studies on the scaling of data and model size as well as on the transfer across tasks. Our models are accessible at https://github.com/Alibaba-NLP/SeqGPT.
更多查看译文
关键词
NLP: (Large) Language Models,NLP: Information Extraction,NLP: Sentence-level Semantics, Textual Inference, etc.,NLP: Sentiment Analysis, Stylistic Analysis, and Argument Mining,NLP: Text Classification
AI 理解论文
溯源树
样例
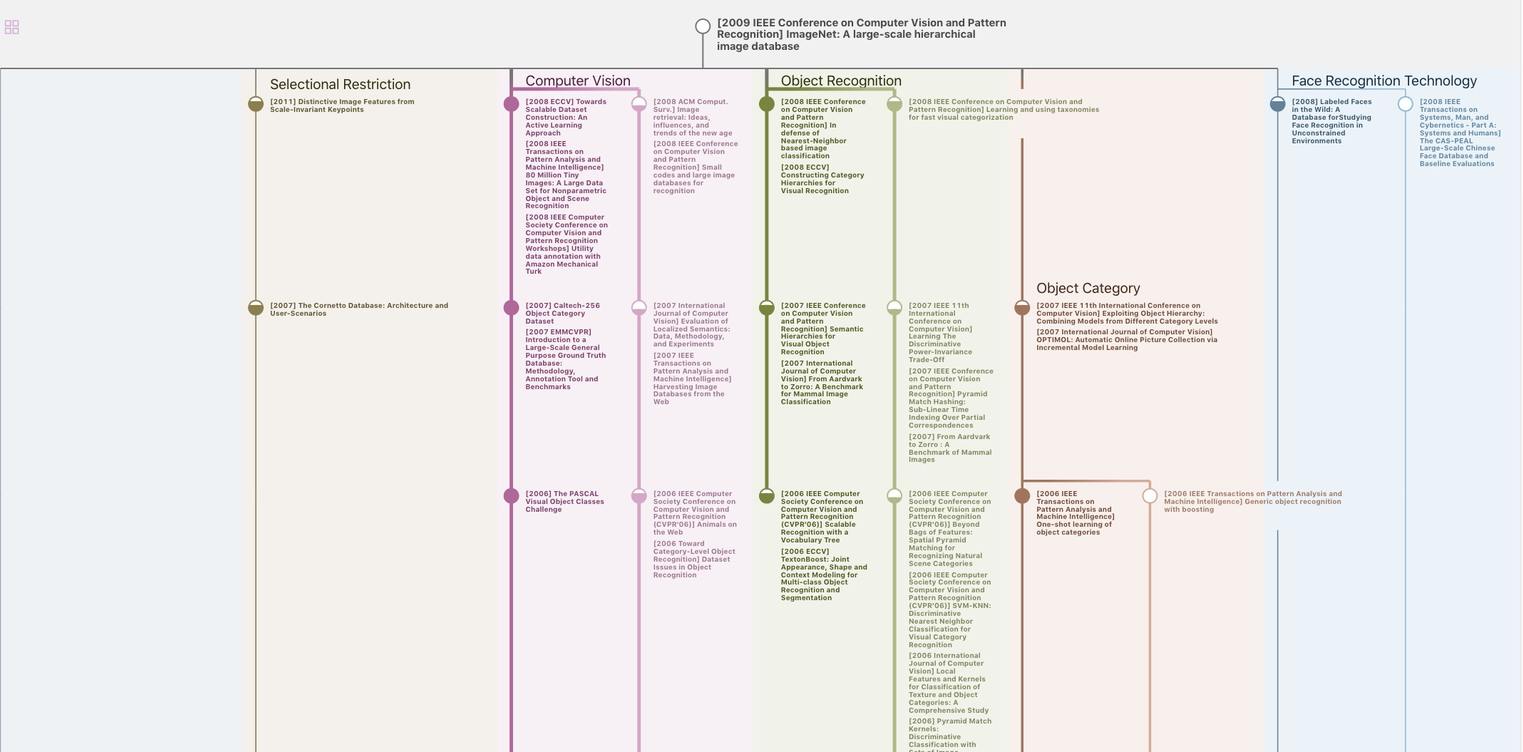
生成溯源树,研究论文发展脉络
Chat Paper
正在生成论文摘要