Semantic Graph Representation Learning for Handwritten Mathematical Expression Recognition
ICDAR (1)(2023)
摘要
Handwritten mathematical expression recognition (HMER) has attracted extensive attention recently. However, current methods cannot explicitly study the interactions between different symbols, which may fail when faced similar symbols. To alleviate this issue, we propose a simple but efficient method to enhance semantic interaction learning (SIL). Specifically, we firstly construct a semantic graph based on the statistical symbol co-occurrence probabilities. Then we design a semantic aware module (SAM), which projects the visual and classification feature into semantic space. The cosine distance between different projected vectors indicates the correlation between symbols. And jointly optimizing HMER and SIL can explicitly enhances the model's understanding of symbol relationships. In addition, SAM can be easily plugged into existing attention-based models for HMER and consistently bring improvement. Extensive experiments on public benchmark datasets demonstrate that our proposed module can effectively enhance the recognition performance. Our method achieves better recognition performance than prior arts on both CROHME and HME100K datasets.
更多查看译文
关键词
handwritten mathematical,graph,representation,recognition,learning
AI 理解论文
溯源树
样例
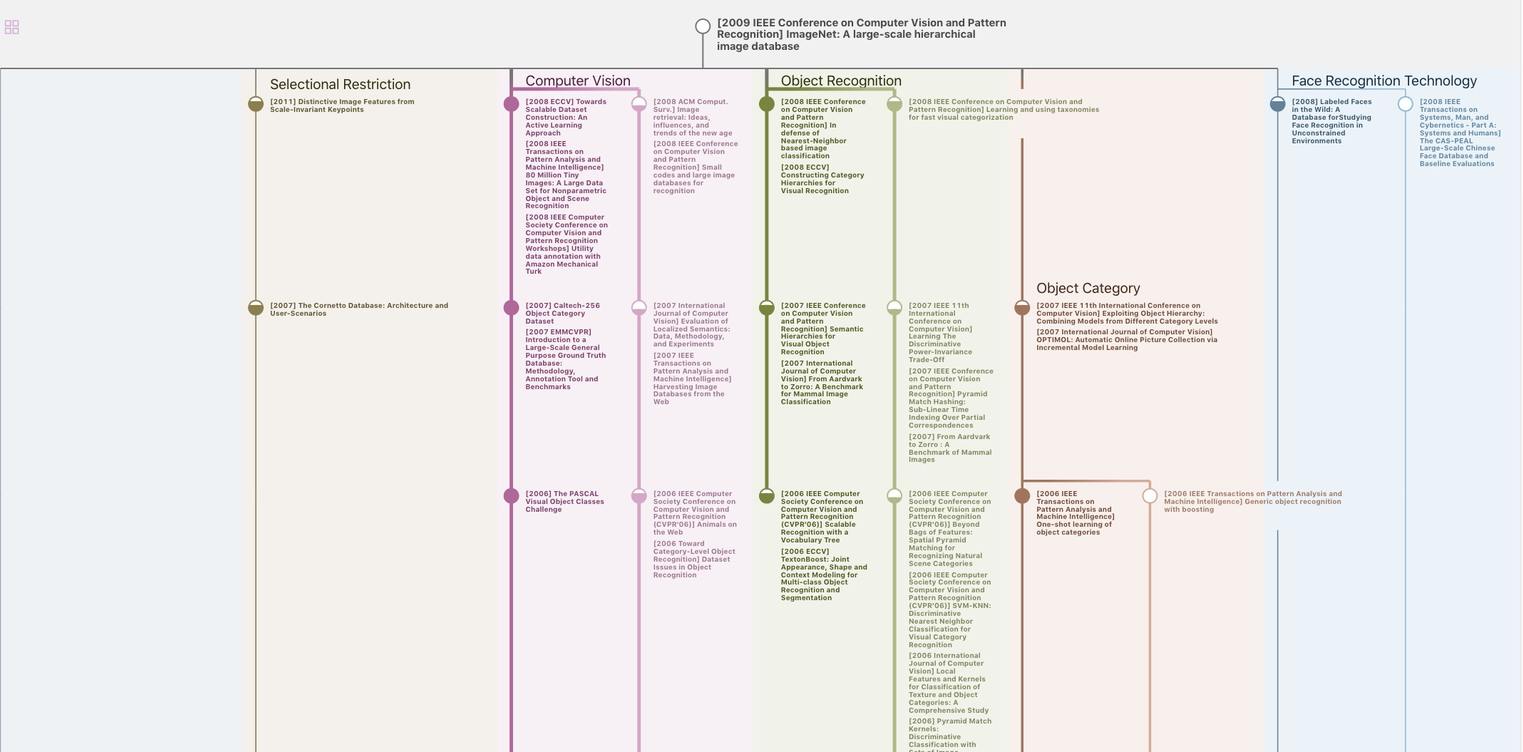
生成溯源树,研究论文发展脉络
Chat Paper
正在生成论文摘要