Almost Tight Bounds for Differentially Private Densest Subgraph
arxiv(2023)
摘要
We study the Densest Subgraph (DSG) problem under the additional constraint
of differential privacy. DSG is a fundamental theoretical question which plays
a central role in graph analytics, and so privacy is a natural requirement. But
all known private algorithms for Densest Subgraph lose constant multiplicative
factors as well as relative large (at least log^2 n) additive factors,
despite the existence of non-private exact algorithms. We show that, perhaps
surprisingly, these losses are not necessary: in both the classic differential
privacy model and the LEDP model (local edge differential privacy, introduced
recently by Dhulipala et al. [FOCS 2022]), we give (ϵ,
δ)-differentially private algorithms with no multiplicative loss
whatsoever. In other words, the loss is purely additive. Moreover, our additive
losses improve the best-known previous additive loss (in any version of
differential privacy) when 1/δ is at least polynomial in n, and are
almost tight: in the centralized setting, our additive loss is O(log n
/ϵ) while there is a known lower bound of Ω(√(log n /
ϵ)). Additionally, we give a different algorithm that is
ϵ-differentially private in the LEDP model which achieves a
multiplicative ratio arbitrarily close to 2, along with an additional
additive factor. This improves over the previous multiplicative
4-approximation in the LEDP model. Finally, we conclude with extensions of
our techniques to both the node-weighted and the directed versions of the
problem.
更多查看译文
关键词
private densest subgraph
AI 理解论文
溯源树
样例
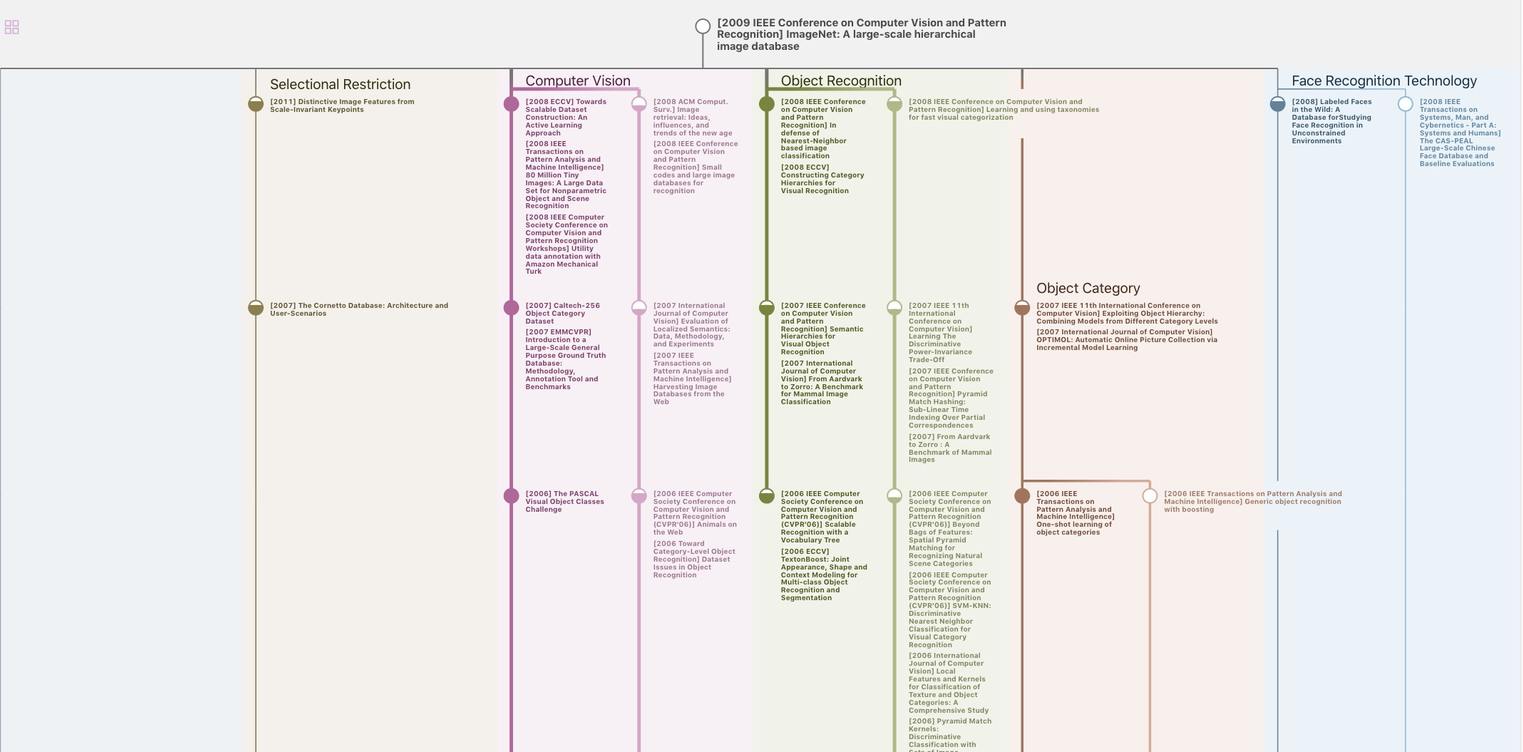
生成溯源树,研究论文发展脉络
Chat Paper
正在生成论文摘要