TDG: Text-guided Domain Generalization
CoRR(2023)
摘要
Domain generalization (DG) attempts to generalize a model trained on single or multiple source domains to the unseen target domain. Benefiting from the success of Visual-and-Language Pre-trained models in recent years, we argue that it is crucial for domain generalization by introducing extra text information. In this paper, we develop a novel Text-guided Domain Generalization (TDG) paradigm for domain generalization, which includes three following aspects. Specifically, we first devise an automatic words generation method to extend the description of current domains with novel domain-relevant words. Then, we embed the generated domain information into the text feature space, by the proposed prompt learning-based text feature generation method, which shares a common representation space with the image feature. Finally, we utilize both input image features and generated text features to train a specially designed classifier that generalizes well on unseen target domains, while the image encoder is also updated under the supervision of gradients back propagated from the classifier. Our experimental results show that the techniques incorporated by TDG contribute to the performance in an easy implementation manner. Experimental results on several domain generalization benchmarks show that our proposed framework achieves superior performance by effectively leveraging generated text information in domain generalization.
更多查看译文
AI 理解论文
溯源树
样例
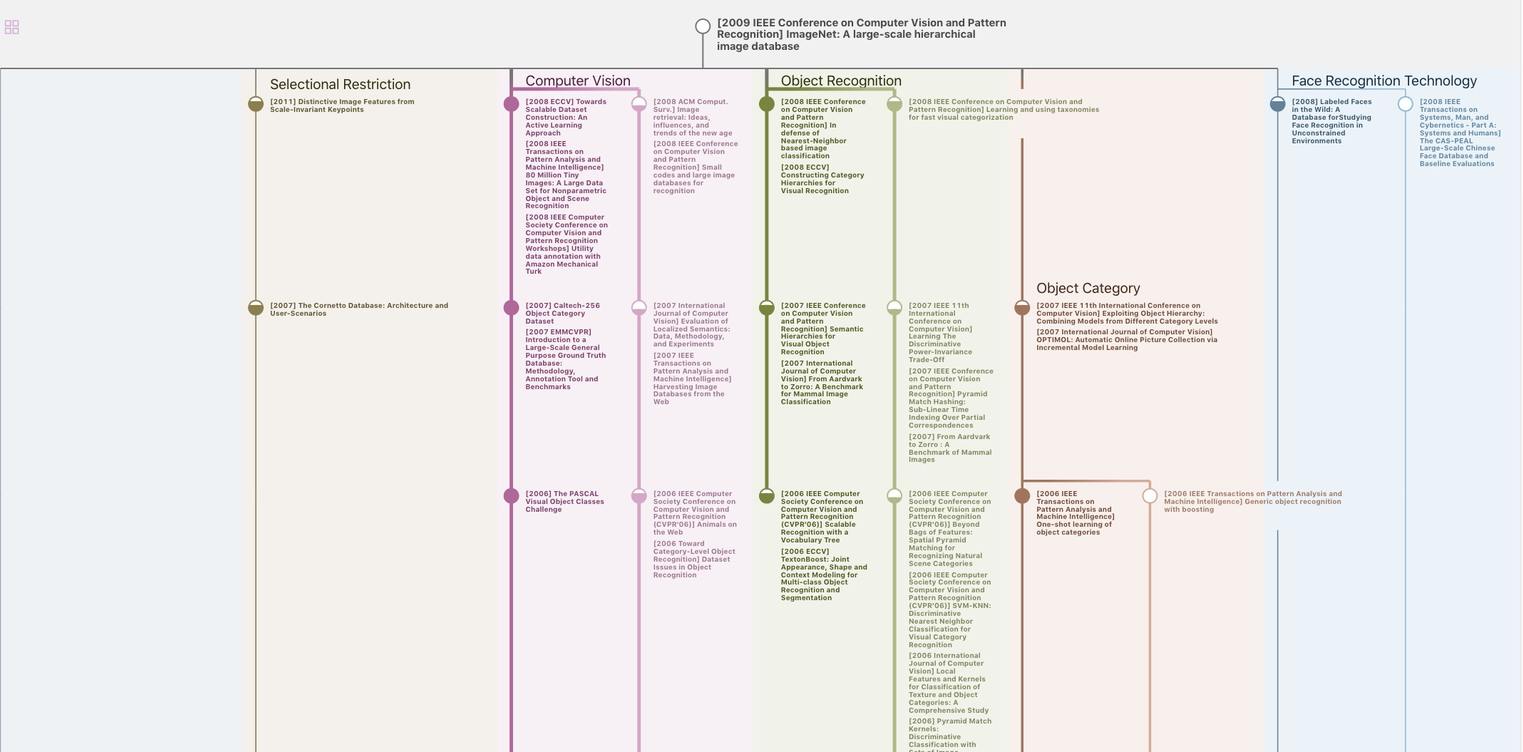
生成溯源树,研究论文发展脉络
Chat Paper
正在生成论文摘要