DoCRL: Double Critic Deep Reinforcement Learning for Mapless Navigation of a Hybrid Aerial Underwater Vehicle with Medium Transition
2023 LATIN AMERICAN ROBOTICS SYMPOSIUM, LARS, 2023 BRAZILIAN SYMPOSIUM ON ROBOTICS, SBR, AND 2023 WORKSHOP ON ROBOTICS IN EDUCATION, WRE(2023)
摘要
Deep Reinforcement Learning (Deep-RL) techniques for motion control have been continuously used to deal with decision-making problems for a wide variety of robots. Previous works showed that Deep-RL can be applied to perform mapless navigation, including the medium transition of Hybrid Unmanned Aerial Underwater Vehicles (HUAUVs). These are robots that can operate in both air and water media, with future potential for rescue tasks in robotics. This paper presents new approaches based on the state-of-the-art Double Critic Actor-Critic algorithms to address the navigation and medium transition problems for a HUAUV. We show that double-critic Deep-RL with Recurrent Neural Networks using range data and relative localization solely improves the navigation performance of HUAUVs. Our DoCRL approaches achieved better navigation and transitioning capability, outperforming previous approaches.
更多查看译文
关键词
hybrid aerial underwater vehicle,mapless navigation,critic
AI 理解论文
溯源树
样例
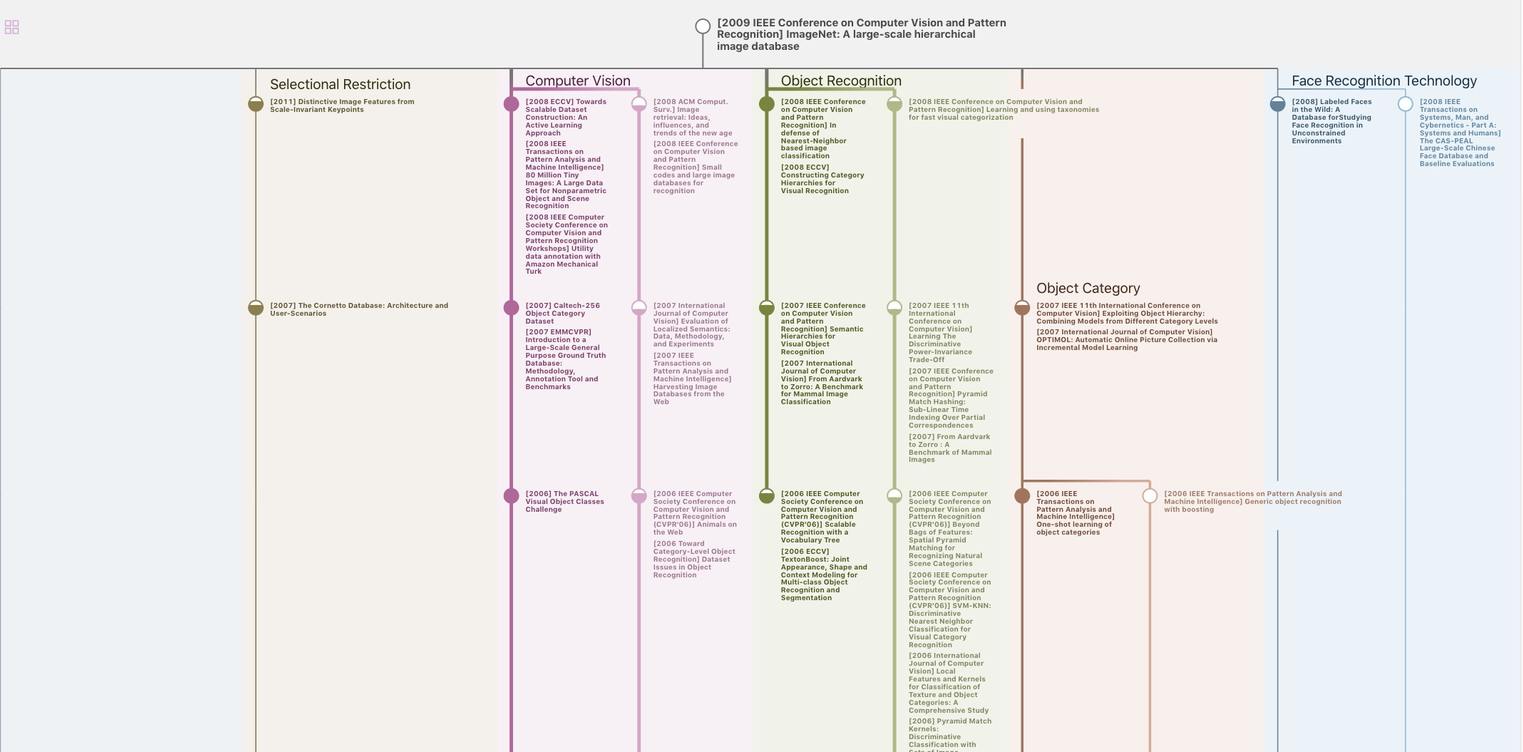
生成溯源树,研究论文发展脉络
Chat Paper
正在生成论文摘要