O^2-Recon: Completing 3D Reconstruction of Occluded Objects in the Scene with a Pre-trained 2D Diffusion Model
Proceedings of the AAAI Conference on Artificial Intelligence(2024)
摘要
Occlusion is a common issue in 3D reconstruction from RGB-D videos, oftenblocking the complete reconstruction of objects and presenting an ongoingproblem. In this paper, we propose a novel framework, empowered by a 2Ddiffusion-based in-painting model, to reconstruct complete surfaces for thehidden parts of objects. Specifically, we utilize a pre-trained diffusion modelto fill in the hidden areas of 2D images. Then we use these in-painted imagesto optimize a neural implicit surface representation for each instance for 3Dreconstruction. Since creating the in-painting masks needed for this process istricky, we adopt a human-in-the-loop strategy that involves very little humanengagement to generate high-quality masks. Moreover, some parts of objects canbe totally hidden because the videos are usually shot from limitedperspectives. To ensure recovering these invisible areas, we develop a cascadednetwork architecture for predicting signed distance field, making use ofdifferent frequency bands of positional encoding and maintaining overallsmoothness. Besides the commonly used rendering loss, Eikonal loss, andsilhouette loss, we adopt a CLIP-based semantic consistency loss to guide thesurface from unseen camera angles. Experiments on ScanNet scenes show that ourproposed framework achieves state-of-the-art accuracy and completeness inobject-level reconstruction from scene-level RGB-D videos. Code:https://github.com/THU-LYJ-Lab/O2-Recon.
更多查看译文
AI 理解论文
溯源树
样例
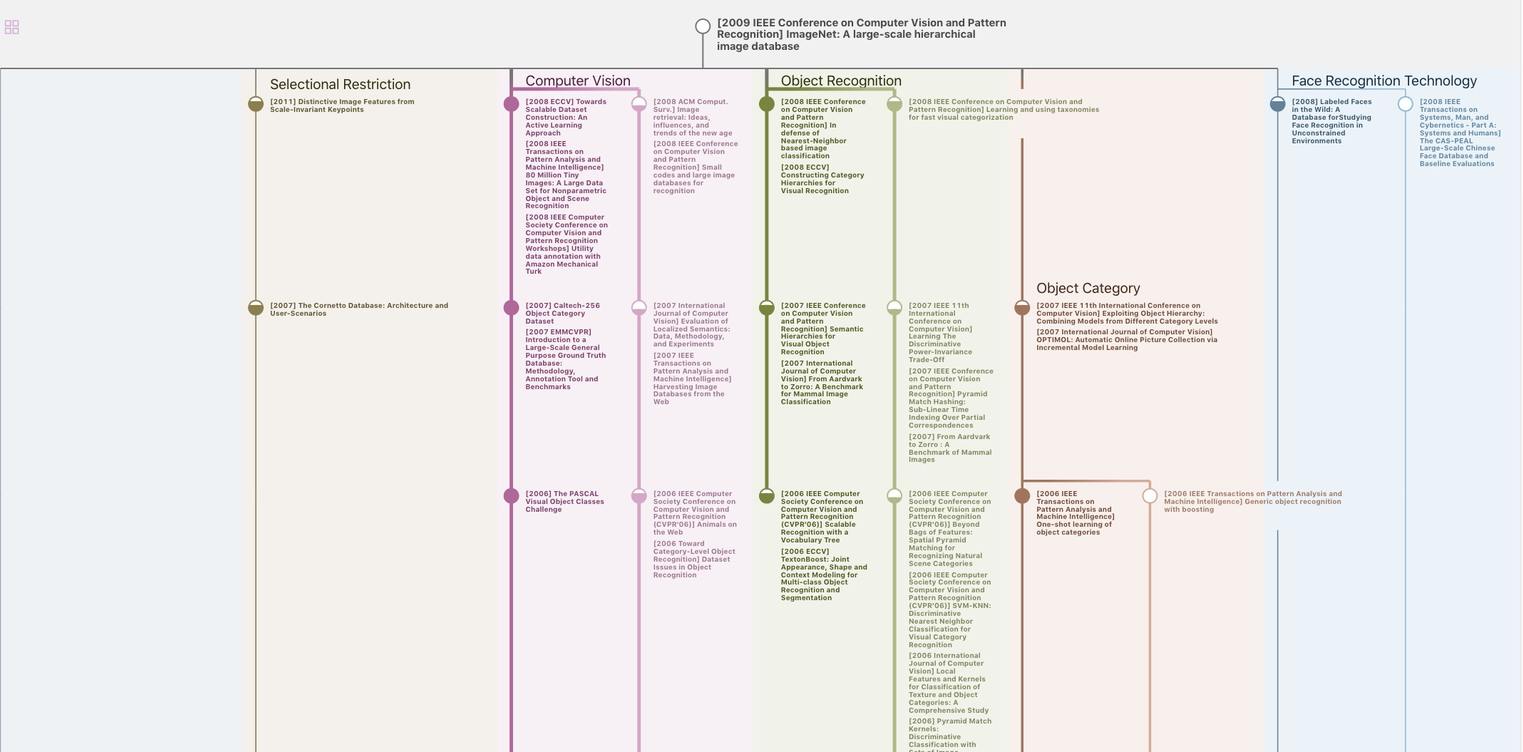
生成溯源树,研究论文发展脉络
Chat Paper
正在生成论文摘要