Causal Adversarial Perturbations for Individual Fairness and Robustness in Heterogeneous Data Spaces
AAAI 2024(2024)
摘要
As responsible AI gains importance in machine learning algorithms, properties like fairness, adversarial robustness, and causality have received considerable attention in recent years. However, despite their individual significance, there remains a critical gap in simultaneously exploring and integrating these properties. In this paper, we propose a novel approach that examines the relationship between individual fairness, adversarial robustness, and structural causal models (SCMs) in heterogeneous data spaces, particularly when dealing with discrete sensitive attributes. We use SCMs and sensitive attributes to create a fair metric and apply it to measure semantic similarity among individuals. By introducing a novel causal adversarial perturbation (CAP) and applying adversarial training, we create a new regularizer that combines individual fairness, causality, and robustness in the classifier. Our method is evaluated on both real-world and synthetic datasets, demonstrating its effectiveness in achieving an accurate classifier that simultaneously exhibits fairness, adversarial robustness, and causal awareness.
更多查看译文
关键词
ML: Ethics, Bias, and Fairness,ML: Adversarial Learning & Robustness
AI 理解论文
溯源树
样例
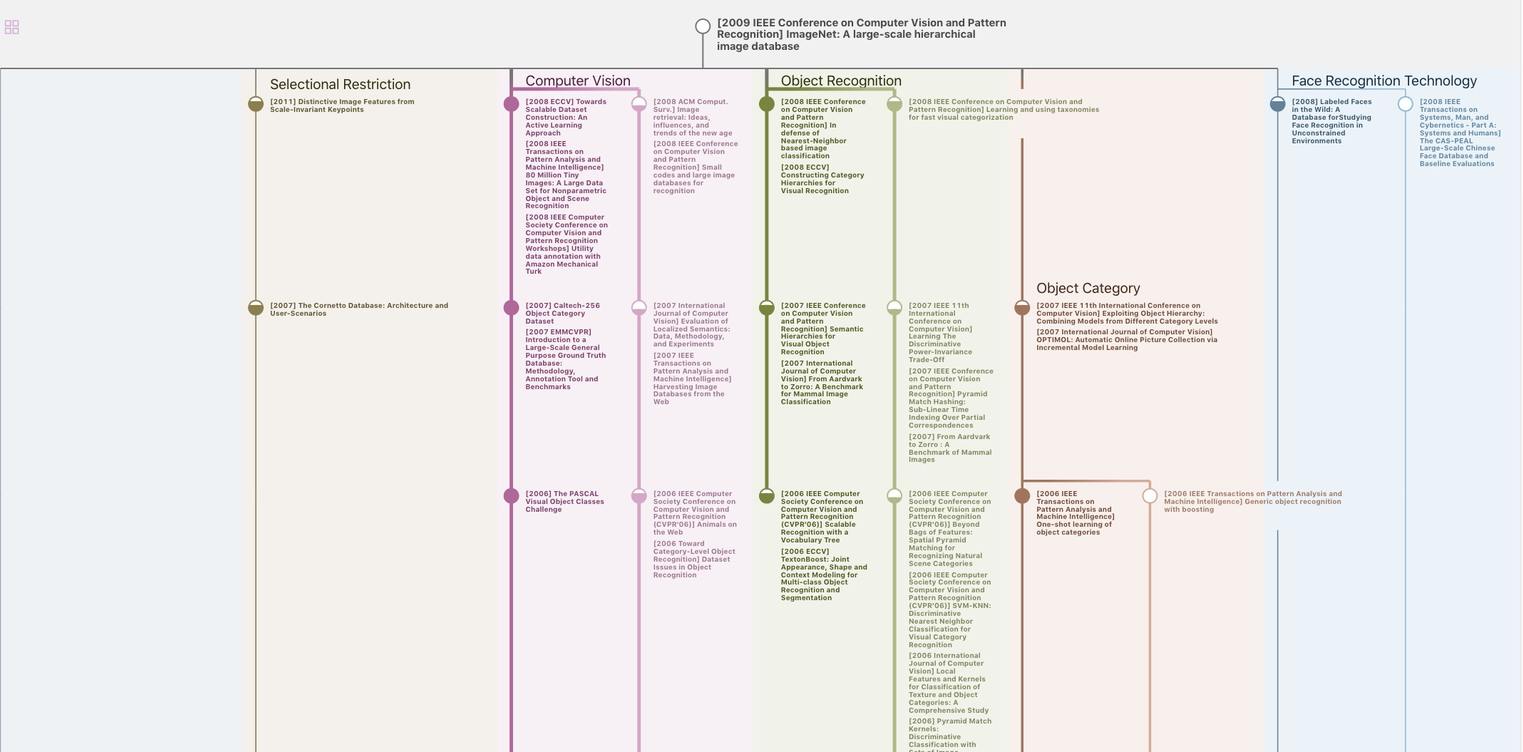
生成溯源树,研究论文发展脉络
Chat Paper
正在生成论文摘要