Mitigating Semantic Confusion from Hostile Neighborhood for Graph Active Learning
PROCEEDINGS OF THE 32ND ACM INTERNATIONAL CONFERENCE ON INFORMATION AND KNOWLEDGE MANAGEMENT, CIKM 2023(2023)
摘要
Graph Active Learning (GAL), which aims to find the most informative nodes in graphs for annotation to maximize the Graph Neural Networks (GNNs) performance, has attracted many research efforts but remains non-trivial challenges. One major challenge is that existing GAL strategies may introduce semantic confusion to the selected training set, particularly when graphs are noisy. Specifically, most existing methods assume all aggregating features to be helpful, ignoring the semantically negative effect between inter-class edges under the message-passing mechanism. In this work, we present Semantic-aware Active learning framework for Graphs (SAG) to mitigate the semantic confusion problem. Pairwise similarities and dissimilarities of nodes with semantic features are introduced to jointly evaluate the node influence. A new prototype-based criterion and query policy are also designed to maintain diversity and class balance of the selected nodes, respectively. Extensive experiments on the public benchmark graphs and a real-world financial dataset demonstrate that SAG significantly improves node classification performances and consistently outperforms previous methods. Moreover, comprehensive analysis and ablation study also verify the effectiveness of the proposed framework.
更多查看译文
关键词
Graph Neural Networks,Active Learning,Semantic Confusion
AI 理解论文
溯源树
样例
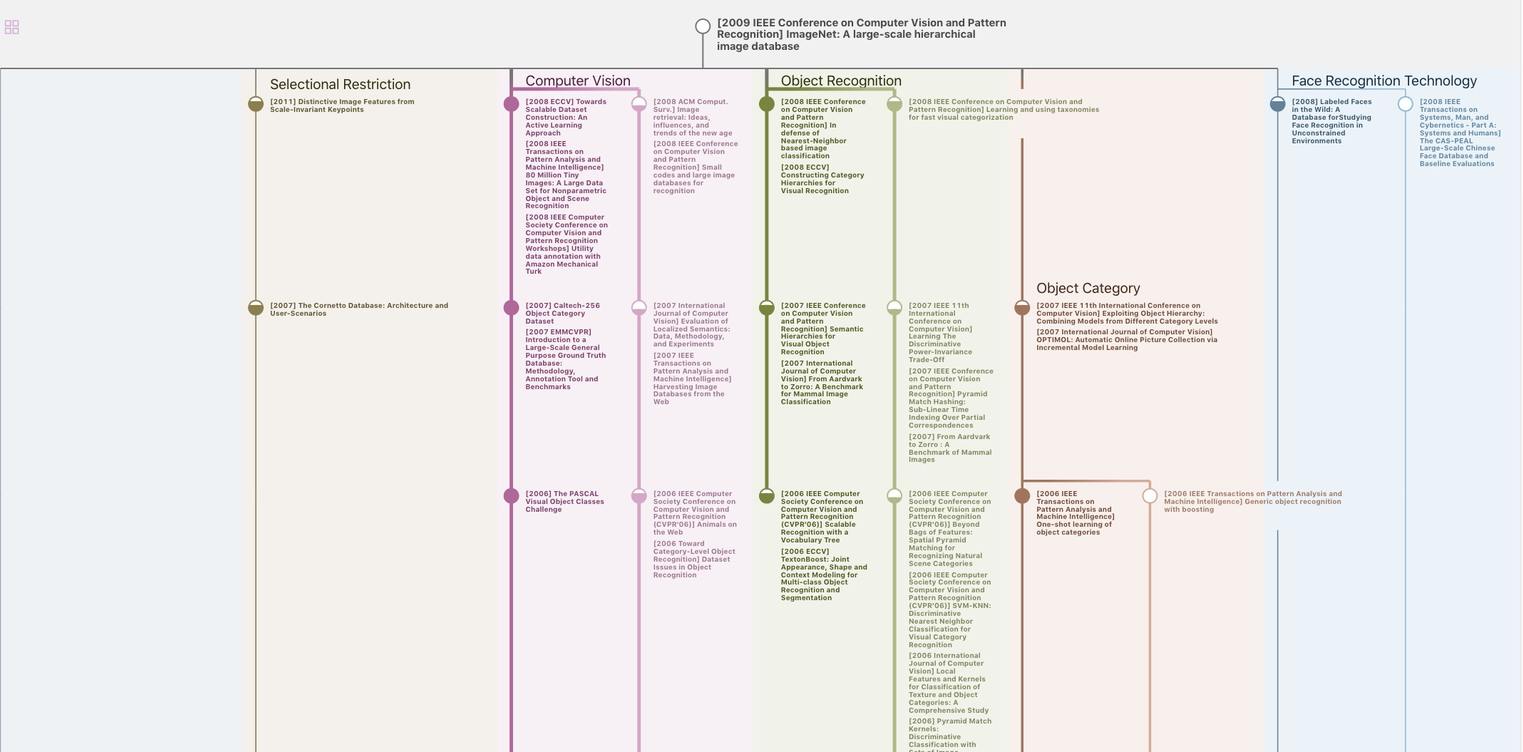
生成溯源树,研究论文发展脉络
Chat Paper
正在生成论文摘要