Evota: an enhanced visual object tracking network with attention mechanism
Multimedia Tools and Applications(2024)
摘要
Transformer architecture has made breakthrough in various downstream computer vision tasks and has shown its great potential in visual object tracking. However, existing transformer-based approaches adopt pixel-to-pixel attention strategy to integrate the domain knowledge, but fail to explore the channel and location information from object features, which limits the expressivity of the tracker. To address the above problems, we propose a novel tracking framework, where we propose 2 attention blocks that fuses with Transformer (dubbed EVOTA). It has 4 modules: the feature extraction module, the enhanced attention module, a transformer module and a model predictor. Specifically, a channel-wise attention module re-calibrates the channel-wise feature responses in an adaptive way by modelling interdependencies explicitly between channels. A local cross-channel interaction scheme learns strong channel context information. Meanwhile, an energy function is developed to analyze the importance of each neuron and infers their 3D weights. Extensive experiments have been carried out on 5 prevalent tracking benchmarks to testify the effectiveness of our model, in which EVOTA outperforms several state-of-the-art methods.
更多查看译文
关键词
Attention mechanism,Visual tracking,Transformer
AI 理解论文
溯源树
样例
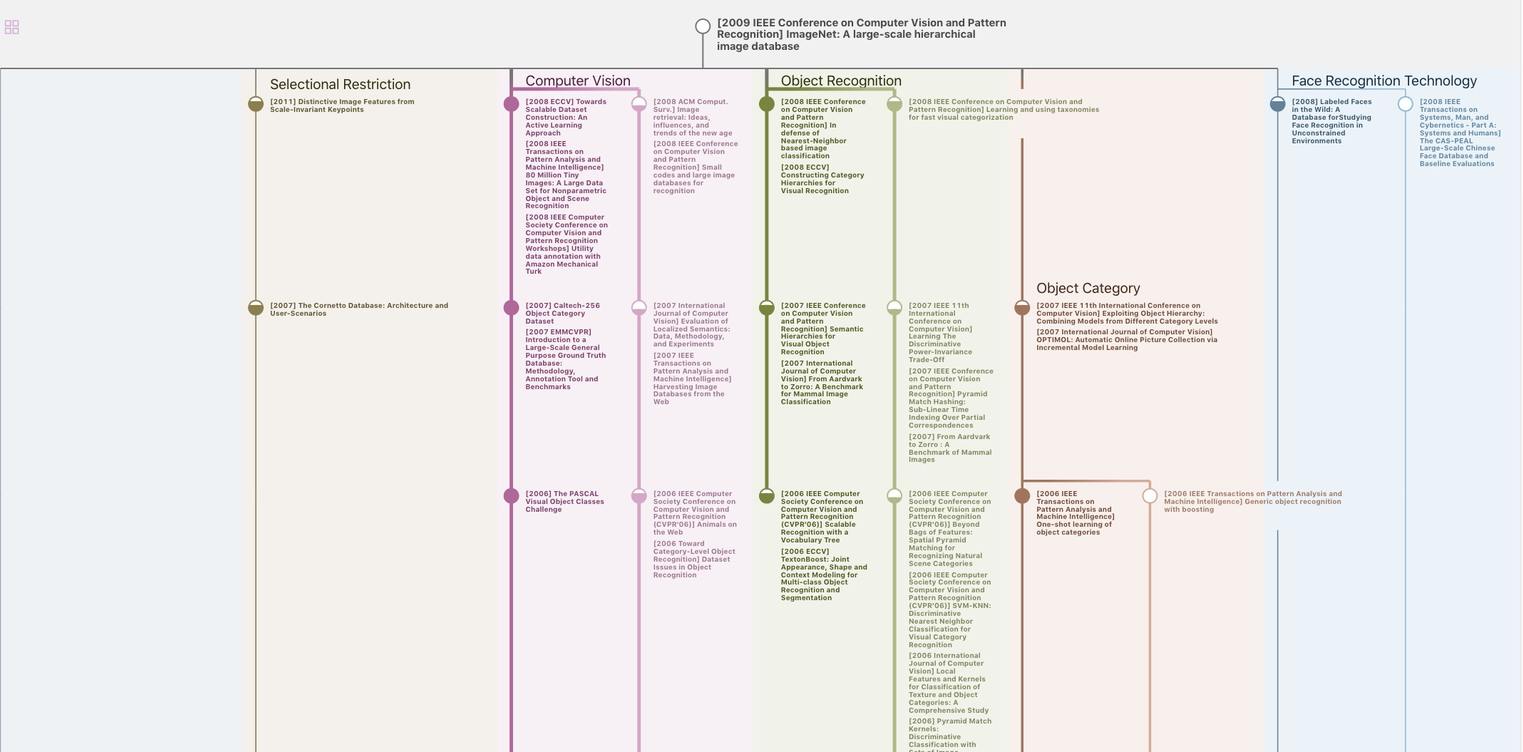
生成溯源树,研究论文发展脉络
Chat Paper
正在生成论文摘要