Joint Gradient Sparsification and Device Scheduling for Federated Learning
IEEE Transactions on Green Communications and Networking(2023)
摘要
Federated learning is an attractive distributed learning framework where edge server coordinates edge devices to collaboratively train an artificial intelligence (AI) model while training data stays in edge devices. However, implementing federated learning in an energy-efficient way still faces some problems in practice: i) uploading high-dimensional parameters of edge devices is energy-costly and causes communication congestion; ii) The heterogeneity of edge devices, i.e., non-i.i.d. data, different software/hardware configurations, and time-varying channel conditions, affects training accuracy and convergence rate of federated learning. To address these issues, in this paper, we aim at minimizing energy use of the heterogeneous edge devices while maintaining the learning performance. Note that the effects of sparsification and the number of participating devices are highly coupled, and they have opposite effects on both learning performance and energy consumption. Therefore, the gradient sparsification and device scheduling should be jointly optimized for federated learning. We consider the case where a fraction of heterogeneous devices are scheduled to upload their sparsified local gradients for global aggregation at the server. First, a tradeoff between the sparsification and the number of participating devices is revealed through the convergence rate analysis. Then, a joint optimization problem is formulated to minimize energy consumption under the convergence guarantee. An efficient algorithm is proposed to find the globally optimal solution of the considered non-convex problem. Simulations verify the efficiency of the proposed scheme.
更多查看译文
关键词
Edge AI,federated learning,gradient sparsification,heterogeneous devices,device scheduling
AI 理解论文
溯源树
样例
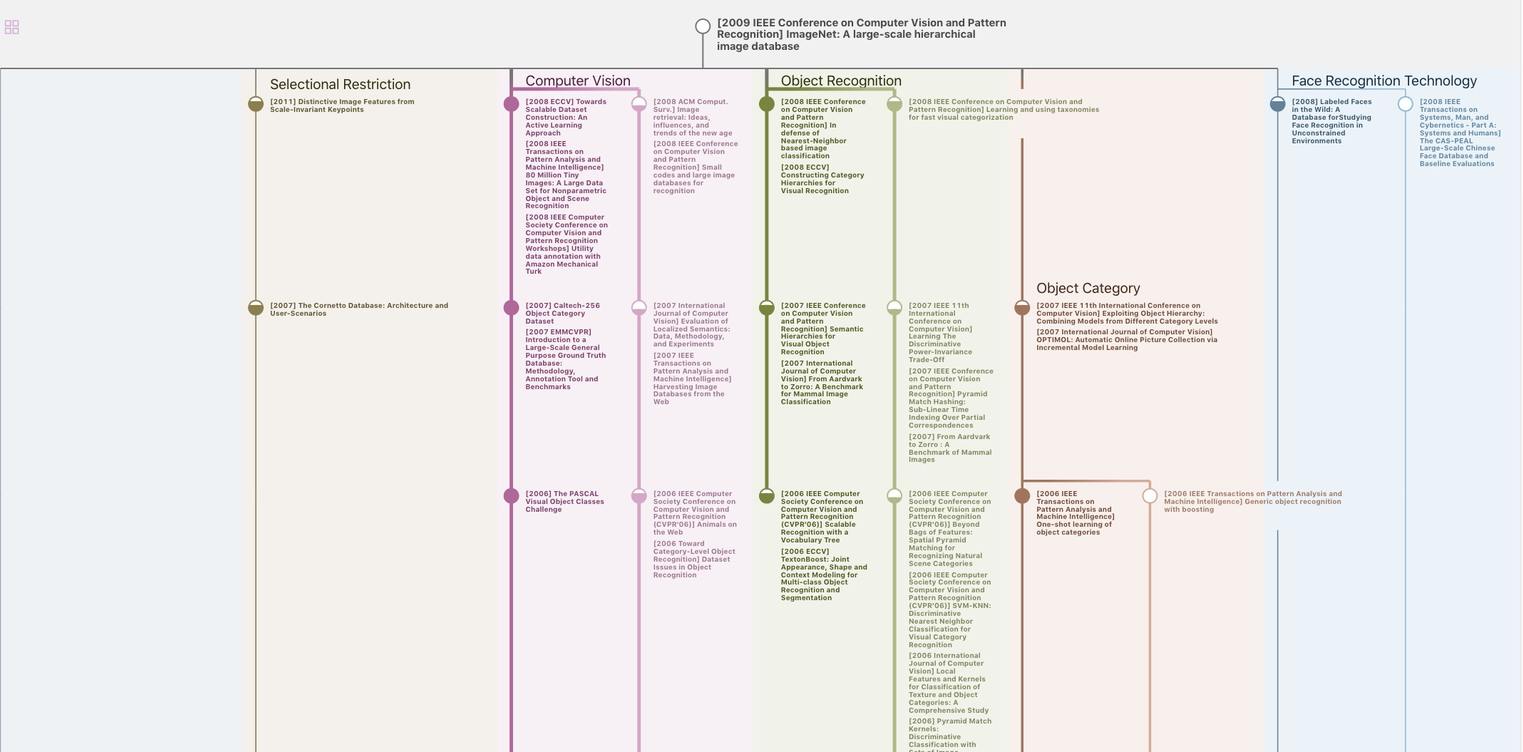
生成溯源树,研究论文发展脉络
Chat Paper
正在生成论文摘要