SPM: Structured Pretraining and Matching Architectures for Relevance Modeling in Meituan Search
PROCEEDINGS OF THE 32ND ACM INTERNATIONAL CONFERENCE ON INFORMATION AND KNOWLEDGE MANAGEMENT, CIKM 2023(2023)
摘要
In e-commerce search, relevance between query and documents is an essential requirement for satisfying user experience. Different from traditional e-commerce platforms that offer products, users search on life service platforms such as Meituan mainly for product providers, which usually have abundant structured information, e.g. name, address, category, thousands of products. Modeling search relevance with these rich structured contents is challenging due to the following issues: (1) there is language distribution discrepancy among different fields of structured document, making it difficult to directly adopt off-the-shelf pretrained language model based methods like BERT. (2) different fields usually have different importance and their length vary greatly, making it difficult to extract document information helpful for relevance matching. To tackle these issues, in this paper we propose a novel two-stage pretraining and matching architecture for relevance matching with rich structured documents. At pretraining stage, we propose an effective pretraining method that employs both query and multiple fields of document as inputs, including an effective information compression method for lengthy fields. At relevance matching stage, a novel matching method is proposed by leveraging domain knowledge in search query to generate more effective document representations for relevance scoring. Extensive offline experiments and online A/B tests on millions of users verify that the proposed architectures effectively improve the performance of relevance modeling. The model has already been deployed online, serving the search traffic of Meituan for over a year.
更多查看译文
关键词
search relevance,pretraining language model
AI 理解论文
溯源树
样例
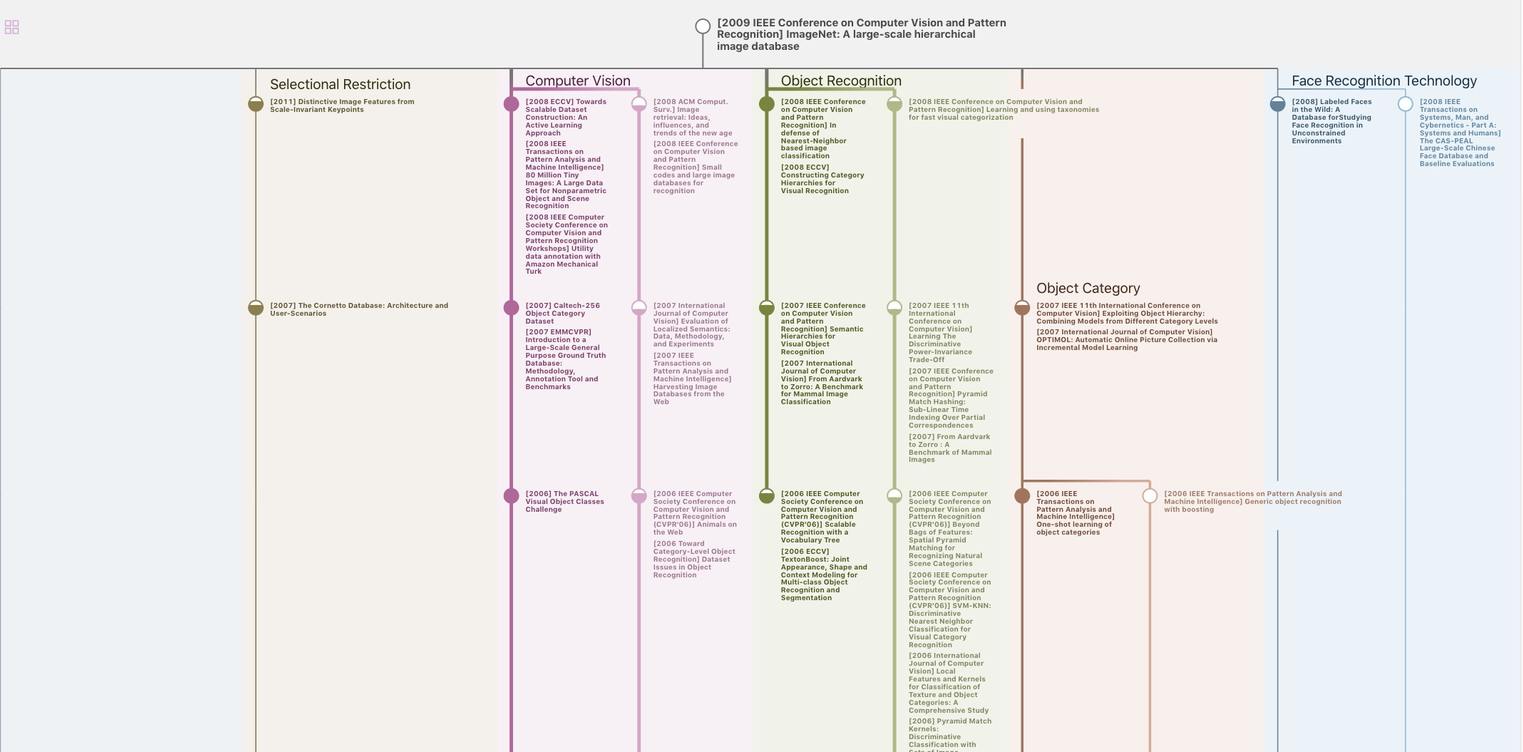
生成溯源树,研究论文发展脉络
Chat Paper
正在生成论文摘要