Quantile Optimization via Multiple-Timescale Local Search for Black-Box Functions
OPERATIONS RESEARCH(2024)
摘要
We consider quantile optimization of black-box functions that are estimated with noise. We propose two new iterative three-timescale local search algorithms. The first algorithm uses an appropriately modified finite-difference-based gradient estimator that requires 2d + 1 samples of the black-box function per iteration of the algorithm, where d is the number of decision variables (dimension of the input vector). For higher-dimensional problems, this algorithm may not be practical if the black-box function estimates are expensive. The second algorithm employs a simultaneous-perturbation-based gradient estimator that uses only three samples for each iteration regardless of problem dimension. Under appropriate conditions, we show the almost sure convergence of both algorithms. In addition, for the class of strongly convex functions, we further establish their (finite-time) convergence rate through a novel fixed-point argument. Simulation experiments indicate that the algorithms work well on a variety of test problems and compare well with recently proposed alternative methods.
更多查看译文
关键词
black-box optimization,quantile,local search,stochastic approximation,finite differences,simultaneous perturbation
AI 理解论文
溯源树
样例
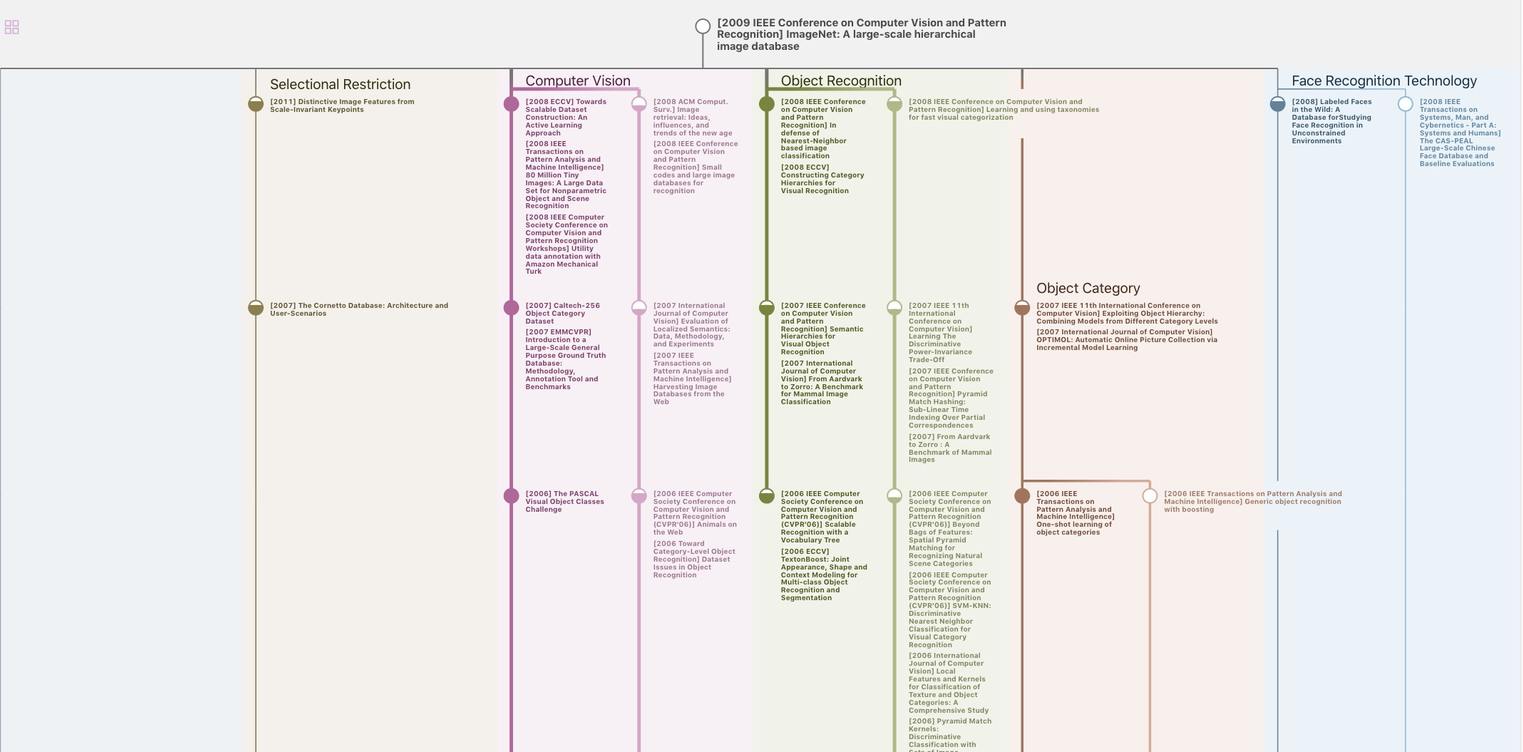
生成溯源树,研究论文发展脉络
Chat Paper
正在生成论文摘要