Efficient Neural PDE-Solvers using Quantization Aware Training
2023 IEEE/CVF INTERNATIONAL CONFERENCE ON COMPUTER VISION WORKSHOPS, ICCVW(2023)
摘要
In the past years, the application of neural networks as an alternative to classical numerical methods to solve Partial Differential Equations has emerged as a potential paradigm shift in this century-old mathematical field. However, in terms of practical applicability, computational cost remains a substantial bottleneck. Classical approaches try to mitigate this challenge by limiting the spatial resolution on which the PDEs are defined. For neural PDE solvers, we can do better: Here, we investigate the potential of state-of-the-art quantization methods on reducing computational costs. We show that quantizing the network weights and activations can successfully lower the computational cost of inference while maintaining performance. Our results on four standard PDE datasets and three network architectures show that quantization-aware training works across settings and three orders of FLOPs magnitudes. Finally, we empirically demonstrate that Pareto-optimality of computational cost vs performance is almost always achieved only by incorporating quantization.
更多查看译文
关键词
neural,pde-solvers
AI 理解论文
溯源树
样例
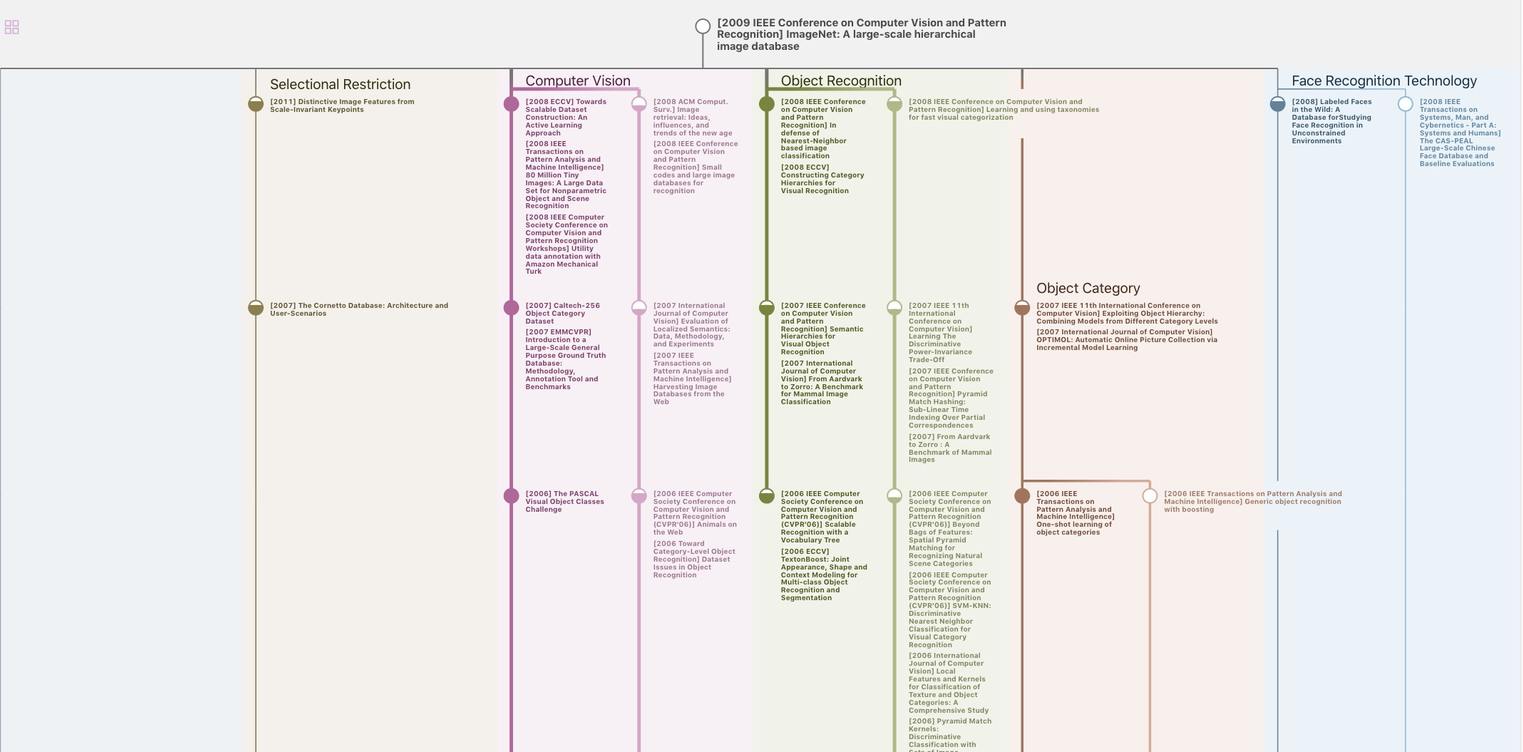
生成溯源树,研究论文发展脉络
Chat Paper
正在生成论文摘要