Machine Learning-Assisted Computationally Efficient Target Detection and Tracking in Massive Fully Digital Phased Arrays
IEEE Transactions on Radar Systems(2023)
摘要
We consider the problem of 3D target detection and tracking using large fully digital radar arrays such as phased array radars or MIMO radars, in the presence of interference or jammers and under realistic array impairments. Accurate target detection and tracking in such case demands a large amount of computation due to beamforming and subsequent detection operations, which can create a bottleneck in the rate at which IQ samples are processed. In addition, the beamforming approach needs to provide suppression of strong jammers under practical array imperfections such as calibration errors. To ensure robust interference suppression and enable real time target tracking, we propose several methods to reduce the complexity of beamforming, detection and tracking. We develop a reinforcement learning-based framework to adaptively control the angle resolution of beamforming and detection based on the location estimates and uncertainties of currently tracked targets. We comprehensively investigate robust and computationally efficient adaptive beamformer design through a closed loop analysis of several covariance based algorithms with reduced complexity of matrix inversion or iterative beamforming coefficient adaptation. We demonstrate and evaluate our methods in both a simulated environment and on a real radar array for long range target tracking with a software-defined GPU-accelerated digital radar processing pipeline incorporating our proposed algorithms.
更多查看译文
关键词
MIMO radar,digital phased array radar,massive array,beamformer design,detection,computational efficiency,reinforcement learning
AI 理解论文
溯源树
样例
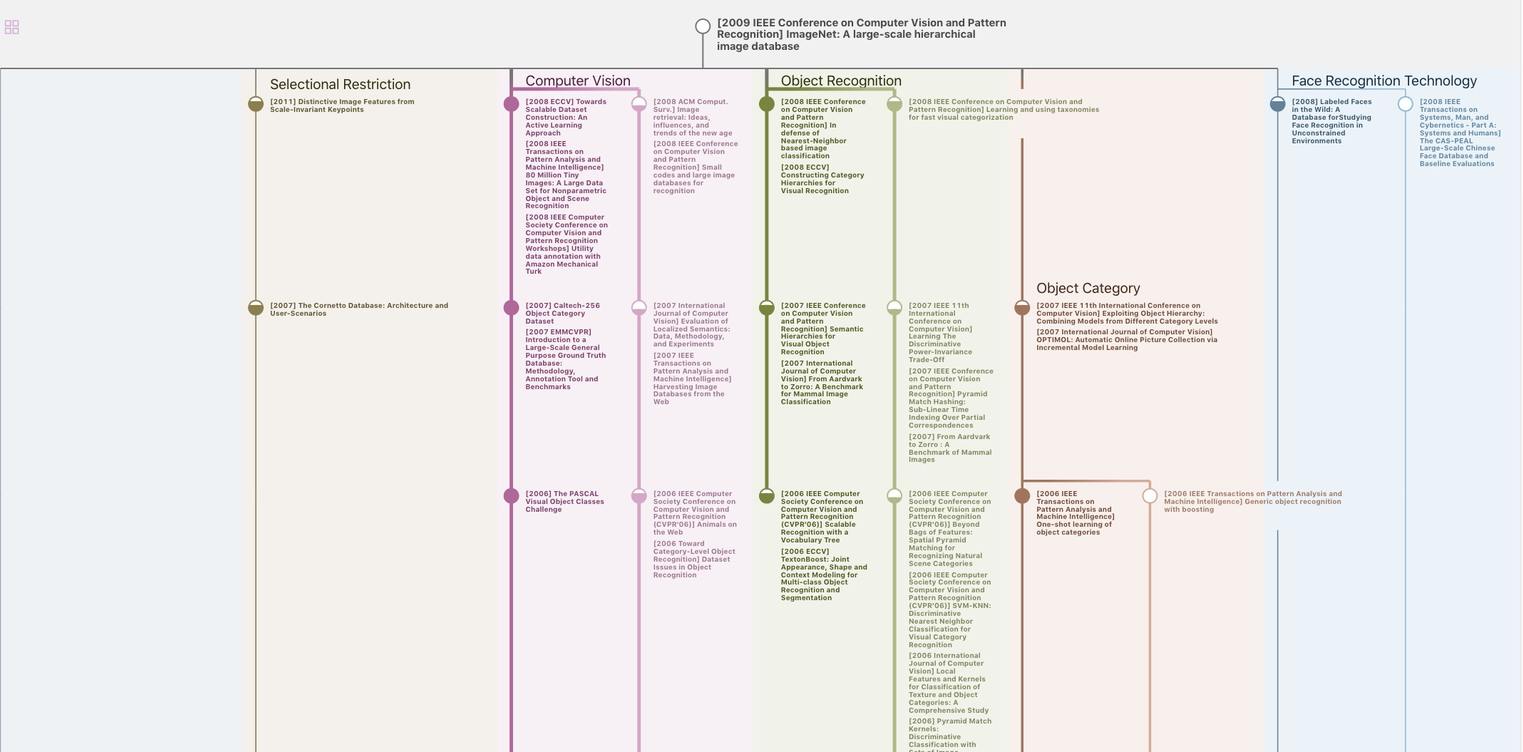
生成溯源树,研究论文发展脉络
Chat Paper
正在生成论文摘要