Multiscale Feature Aggregation Network for Salient Object Detection in Optical Remote Sensing Images
IEEE Sensors Journal(2023)
摘要
Optical remote sensing images (ORSIs) have various applications in different fields, and salient target detection (ORSI-SOD) of ORSI has become an important research topic in recent years. However, ORSI-SOD is a challenging problem due to the variable and complex backgrounds, large differences in levels, mixed backgrounds, and diverse topological shapes of ORSI. In this article, we propose a novel model called a multiscale feature refinement aggregation network (MFANet), which consists of a multiscale feature refinement (MFR) module and a context feature aggregation (CFA) module. The MFR module extracts semantic information of ORSI across different dimensions in the multiscale feature extraction stage. In the feature refinement stage, we use the proposed self-refinement module under the guidance of attention and reverse attention to progressively refine the prediction results. The CFA module introduces the hybrid attention module to gradually aggregate and extract salient regions from the context extraction module. To adapt to dense scenes, we develop a hybrid loss function that enables network optimization of multiscale objectives in a self-adaptive manner. Our method outperforms most state-of-the-art salient object detection (SOD) methods proposed in recent years in terms of accuracy.
更多查看译文
关键词
Index Terms- Feature refinement,hybrid loss,multiscale,optical remote sensing image salient object detection (ORSI-SOD),pyramid feature
AI 理解论文
溯源树
样例
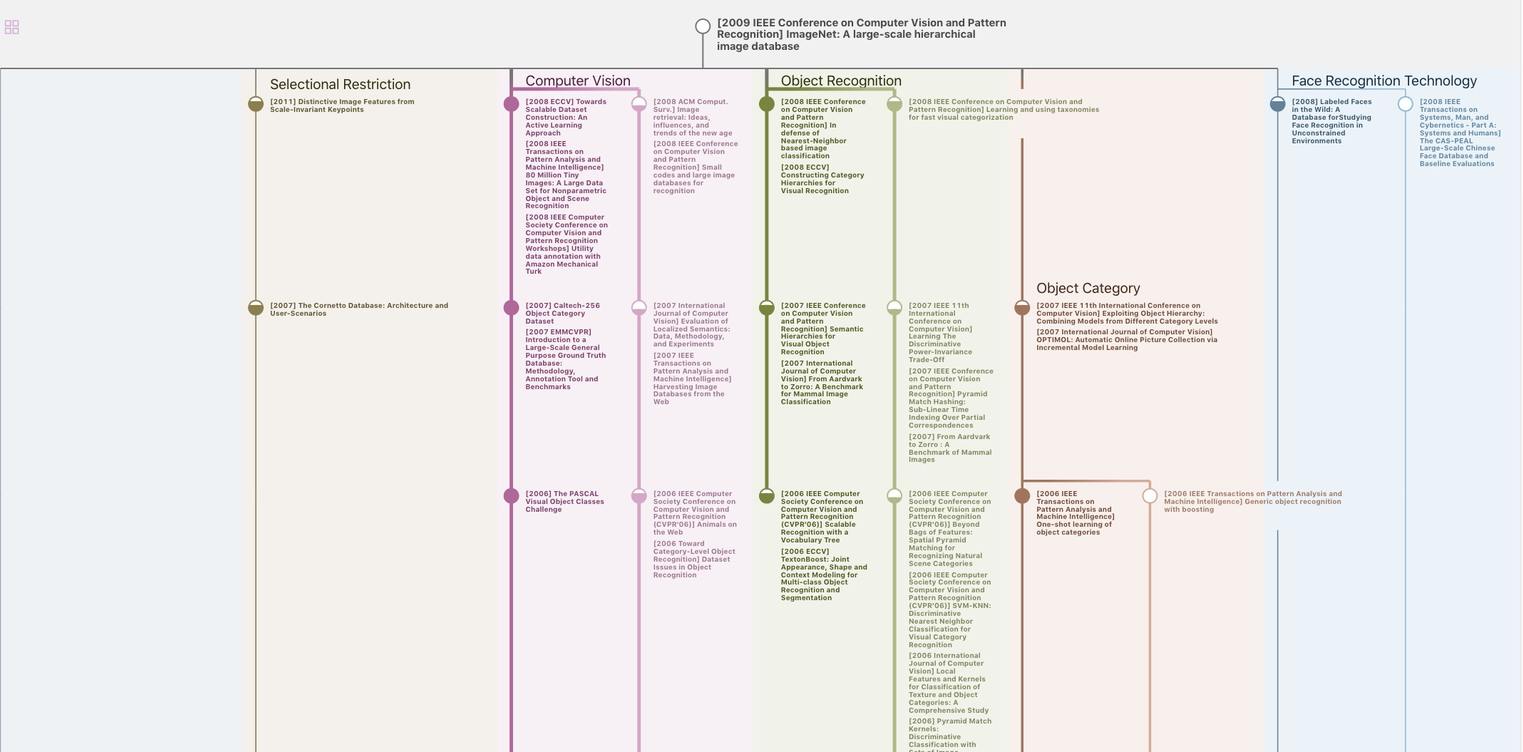
生成溯源树,研究论文发展脉络
Chat Paper
正在生成论文摘要