Learning Control Policies for Variable Objectives from Offline Data
CoRR(2023)
摘要
Offline reinforcement learning provides a viable approach to obtain advanced control strategies for dynamical systems, in particular when direct interaction with the environment is not available. In this paper, we introduce a conceptual extension for model-based policy search methods, called variable objective policy (VOP). With this approach, policies are trained to generalize efficiently over a variety of objectives, which parameterize the reward function. We demonstrate that by altering the objectives passed as input to the policy, users gain the freedom to adjust its behavior or re-balance optimization targets at runtime, without need for collecting additional observation batches or re-training.
更多查看译文
关键词
learning control policies,variable objectives
AI 理解论文
溯源树
样例
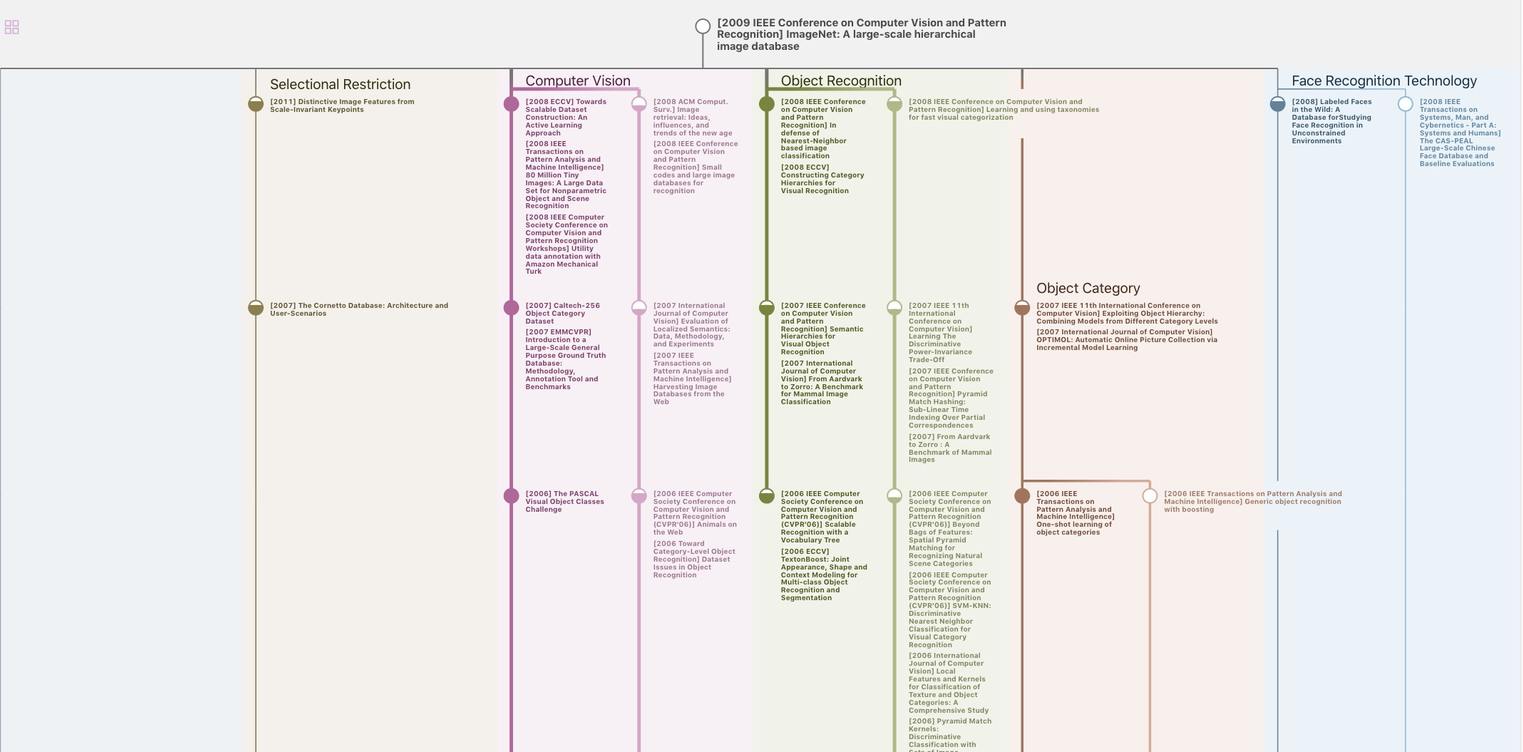
生成溯源树,研究论文发展脉络
Chat Paper
正在生成论文摘要