Safeguarding Learning-based Control for Smart Energy Systems with Sampling Specifications
CoRR(2023)
摘要
We study challenges using reinforcement learning in controlling energy systems, where apart from performance requirements, one has additional safety requirements such as avoiding blackouts. We detail how these safety requirements in real-time temporal logic can be strengthened via discretization into linear temporal logic (LTL), such that the satisfaction of the LTL formulae implies the satisfaction of the original safety requirements. The discretization enables advanced engineering methods such as synthesizing shields for safe reinforcement learning as well as formal verification, where for statistical model checking, the probabilistic guarantee acquired by LTL model checking forms a lower bound for the satisfaction of the original real-time safety requirements.
更多查看译文
关键词
safety,reinforcement learning,energy grids
AI 理解论文
溯源树
样例
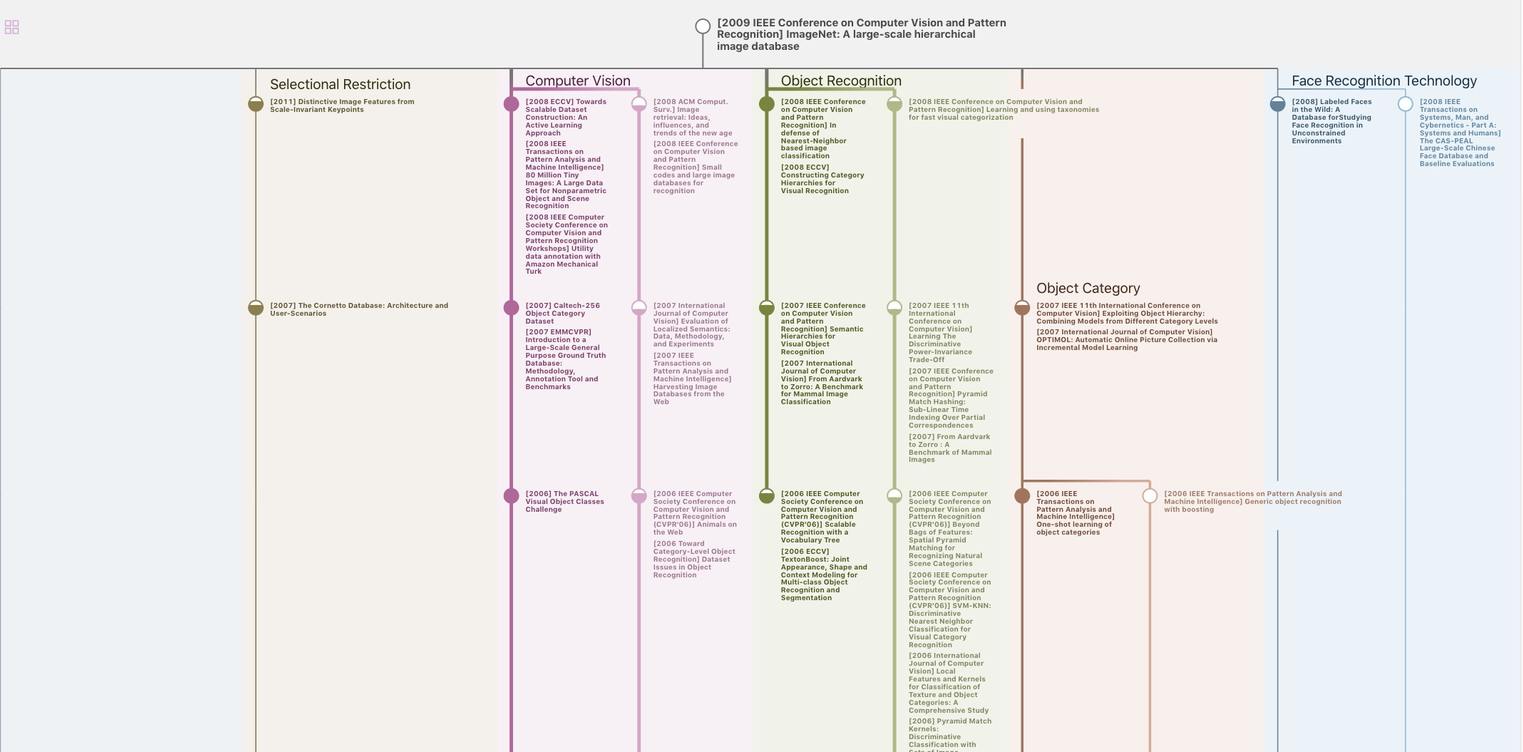
生成溯源树,研究论文发展脉络
Chat Paper
正在生成论文摘要