Spatial-information Guided Adaptive Context-aware Network for Efficient RGB-D Semantic Segmentation
CoRR(2023)
摘要
Efficient RGB-D semantic segmentation has received considerable attention in mobile robots, which plays a vital role in analyzing and recognizing environmental information. According to previous studies, depth information can provide corresponding geometric relationships for objects and scenes, but actual depth data usually exist as noise. To avoid unfavorable effects on segmentation accuracy and computation, it is necessary to design an efficient framework to leverage cross-modal correlations and complementary cues. In this paper, we propose an efficient lightweight encoder-decoder network that reduces the computational parameters and guarantees the robustness of the algorithm. Working with channel and spatial fusion attention modules, our network effectively captures multi-level RGB-D features. A globally guided local affinity context module is proposed to obtain sufficient high-level context information. The decoder utilizes a lightweight residual unit that combines short- and long-distance information with a few redundant computations. Experimental results on NYUv2, SUN RGB-D, and Cityscapes datasets show that our method achieves a better trade-off among segmentation accuracy, inference time, and parameters than the state-of-the-art methods. The source code will be at https://github.com/MVME-HBUT/SGACNet
更多查看译文
关键词
Efficient,encoder–decoder framework,RGB-D semantic segmentation,spatial and channel attention
AI 理解论文
溯源树
样例
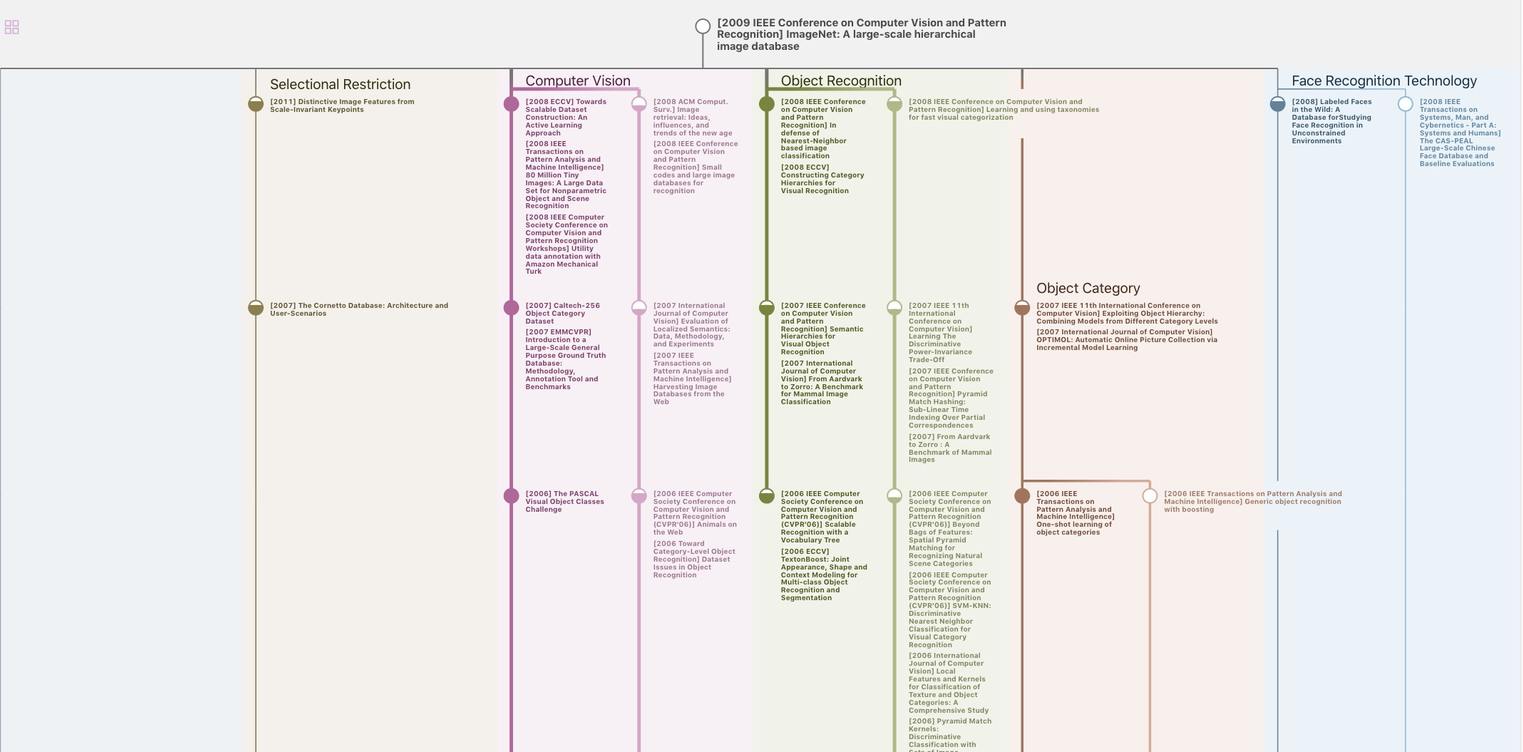
生成溯源树,研究论文发展脉络
Chat Paper
正在生成论文摘要