ATNAS: Automatic Termination for Neural Architecture Search.
Neural networks : the official journal of the International Neural Network Society(2023)
摘要
Neural architecture search (NAS) is a framework for automating the design process of a neural network structure. While the recent one-shot approaches have reduced the search cost, there still exists an inherent trade-off between cost and performance. It is important to appropriately stop the search and further reduce the high cost of NAS. Meanwhile, the differentiable architecture search (DARTS), a typical one-shot approach, is known to suffer from overfitting. Heuristic early-stopping strategies have been proposed to overcome such performance degradation. In this paper, we propose a more versatile and principled early-stopping criterion on the basis of the evaluation of a gap between expectation values of generalisation errors of the previous and current search steps with respect to the architecture parameters. The stopping threshold is automatically determined at each search epoch without cost. In numerical experiments, we demonstrate the effectiveness of the proposed method. We stop the one-shot NAS algorithms and evaluate the acquired architectures on the benchmark datasets: NAS-Bench-201 and NATS-Bench. Our algorithm is shown to reduce the cost of the search process while maintaining a high performance.
更多查看译文
关键词
Neural Architecture Search,Deep learning
AI 理解论文
溯源树
样例
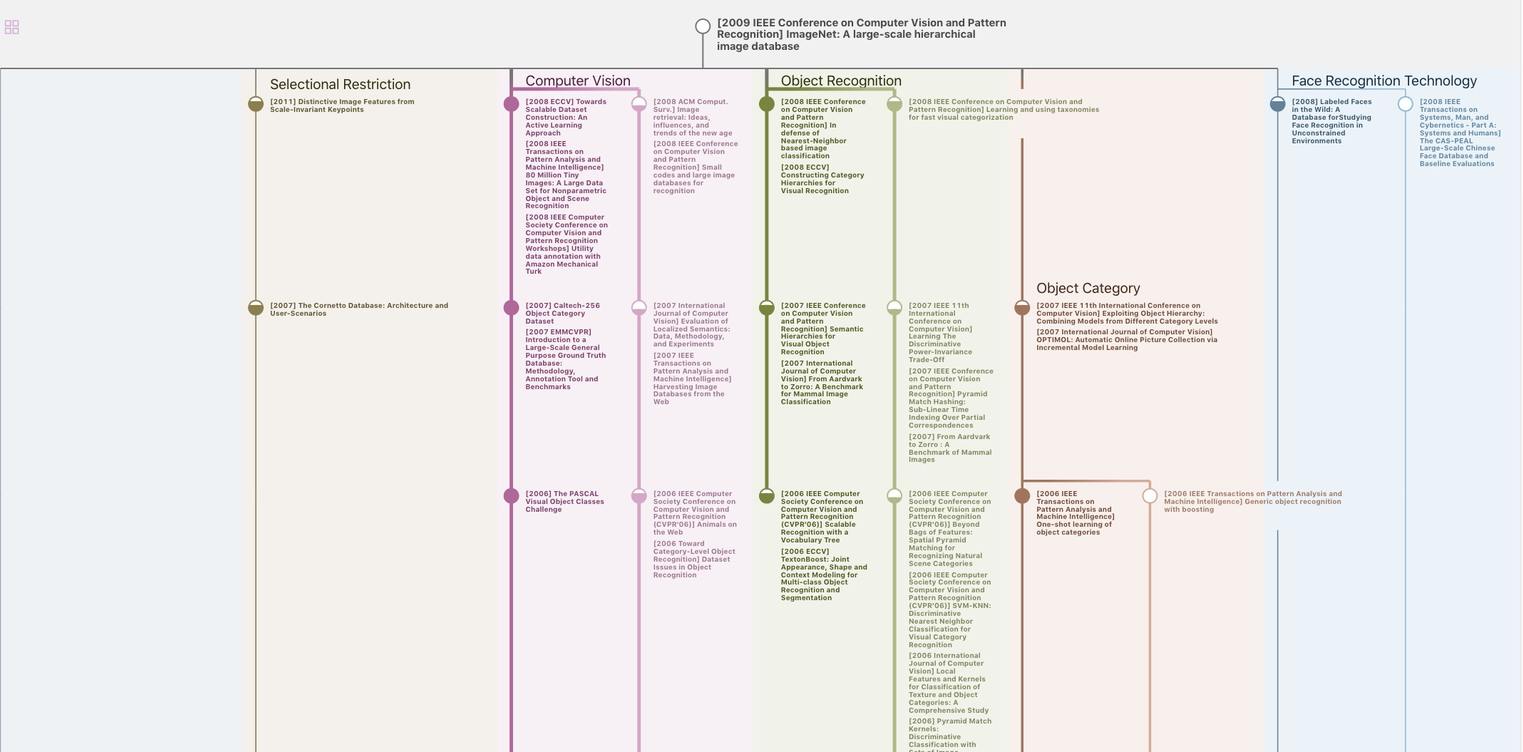
生成溯源树,研究论文发展脉络
Chat Paper
正在生成论文摘要