Trajectory Learning and Reproduction for Tracked Robot Based on Bagging-GMM/HSMM
Journal of Electrical Engineering & Technology(2023)
摘要
Learning and reproducing the tracked robot’s demonstration trajectory is a promising intelligent path planning solution that can summarize and extract the relevant characteristics of the tracked robot’s desired trajectory. However, the existing methods are difficult to ensure the robustness when there are deviations in the demonstration trajectory data or when the task constraints are added. In order to address the problem, this paper proposes a novel trajectory learning and reproduction method for tracked robots that is based on Bagging algorithm and GMM/HSMM. The Bagging algorithm is used to randomly resample the demonstration trajectory data and construct the sub trajectory data sets. Then, GMM/HSMM is used to train and learn these sub trajectory data sets, and the output probability density function of the hidden state and the Gaussian component with the largest average position probability are selected as the motion elements. According to the mean and variance of these motion elements, the least square method is used to reconstruct the tracked robot’s trajectory. On the basis of using the IPSO algorithm to optimize the position of constraint points in each sub trajectory data sets, combined with the weight method, the learning results are integrated to realize the trajectory reproduction of a tracked robot under task constraints. The final results and analysis show that the proposed method can successfully realize the trajectory learning and reproduction of tracked robot, as well as ensure that the reproduced trajectory can pass through the required task constraint points without increasing the algorithm’s complexity.
更多查看译文
关键词
Trajectory learning,GMM,HSMM,Bagging,Tracked robot
AI 理解论文
溯源树
样例
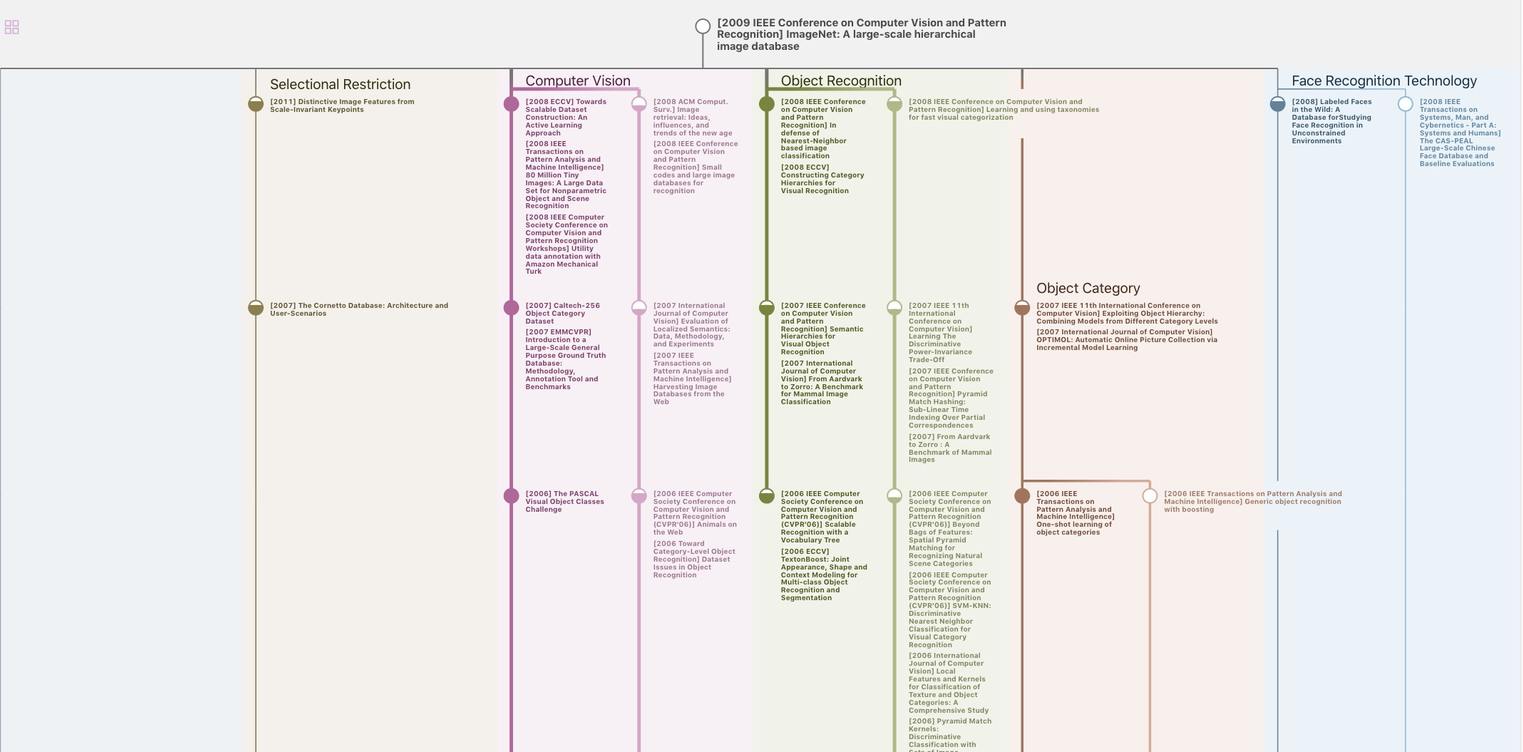
生成溯源树,研究论文发展脉络
Chat Paper
正在生成论文摘要