Genre Recognition from Symbolic Music with CNNs: Performance and Explainability
SN Computer Science(2022)
摘要
In this work, we study the use of convolutional neural networks for genre recognition in symbolically represented music. Specifically, we explore the effects of changing network depth, width and kernel sizes while keeping the number of trainable parameters and each block’s receptive field constant. We propose an architecture for handling MIDI data that makes use of multiple resolutions of the input, called Multiple Sequence Resolution Network (MuSeReNet). These networks accept multiple inputs, each at half the original sequence length, representing information at a lower resolution. Through our experiments, we outperform the state-of-the-art for MIDI genre recognition on the topMAGD and MASD datasets. Finally, we adapt various post hoc explainability methods to the domain of symbolic music and attempt to explain the predictions of our best performing network.
更多查看译文
关键词
Artificial intelligence,Machine learning,Music information retrieval,Genre classification,Explainability,Genetic programming,XAI
AI 理解论文
溯源树
样例
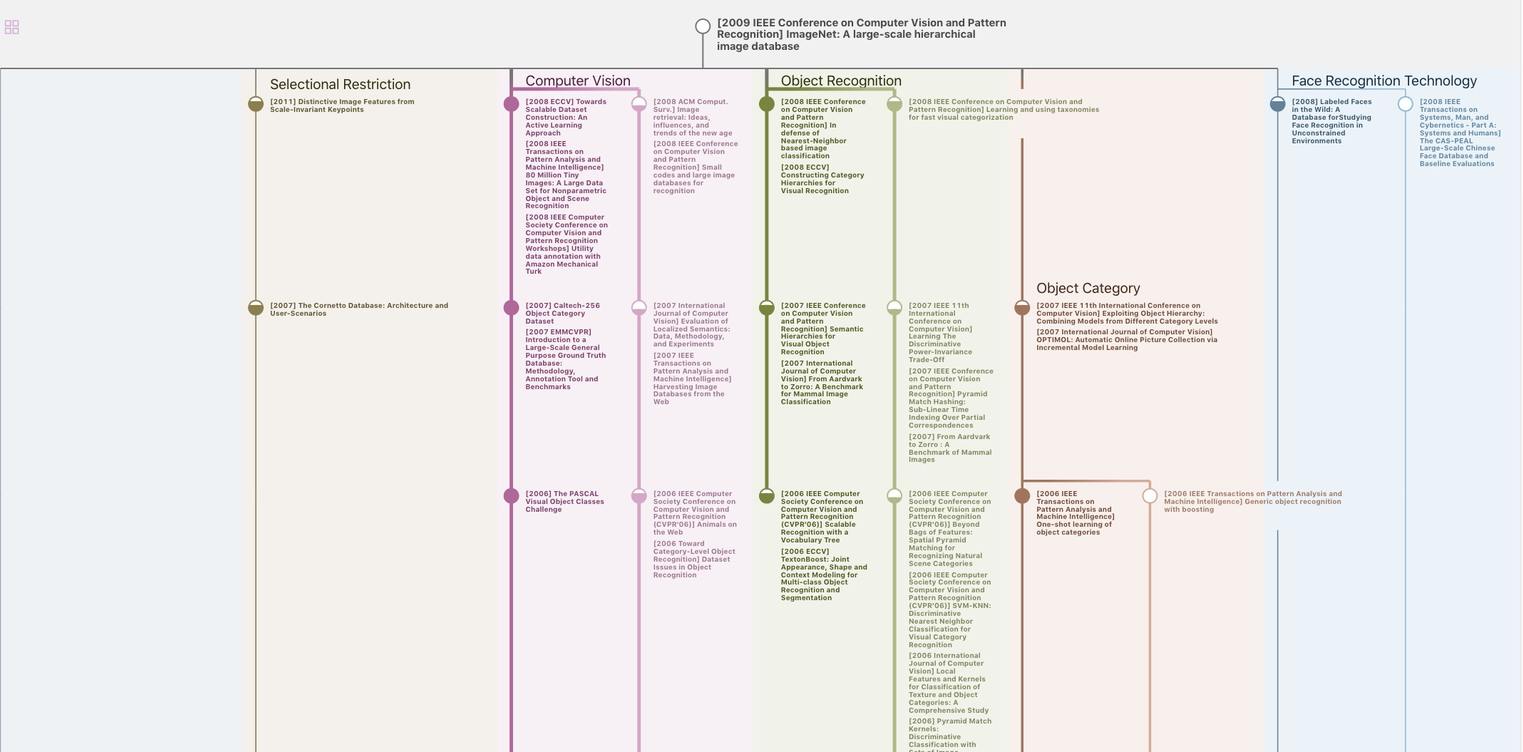
生成溯源树,研究论文发展脉络
Chat Paper
正在生成论文摘要