Improving the speed and quality of cancer segmentation using lower resolution pathology images
Multimedia Tools and Applications(2024)
摘要
In this paper, we propose a pipeline to investigate the performance of semantic segmentation model that employs an encoder-decoder architecture with atrous separable convolution and spatial pyramid pooling, trained on multi-resolution whole slide breast pathological images with different patch sizes. Our segmentation model obtains the best performance on zoom level 2 (10 × magnification) with AUC score 0.974 in terms of slide-level classification. This outperforms both the performance of the pathologist and other semantic segmentation models on the Camelyon16 dataset. By offering a larger field of view and reducing noise and detail, training a semantic segmentation model on the properly selected lower resolution pathology images can further improve the precision of pixel-wise cancer region segmentation. By contrast, the corresponding inference time is 14 times shorter than the inference time trained on the highest resolution patches, and it is also shorter than the time required by a pathologist with time constraints. Moreover, we prove that the model trained on lower resolution patches can still generate refined external polygons of cancer region on the highest resolution image. This study provides new insights into efficient gigapixel histopathology analysis that will make clinical adoption more likely.
更多查看译文
关键词
Semantic segmentation,Breast cancer,Whole-slide imaging,Gigapixel histopathology,Pixel-based convolutional neural networks
AI 理解论文
溯源树
样例
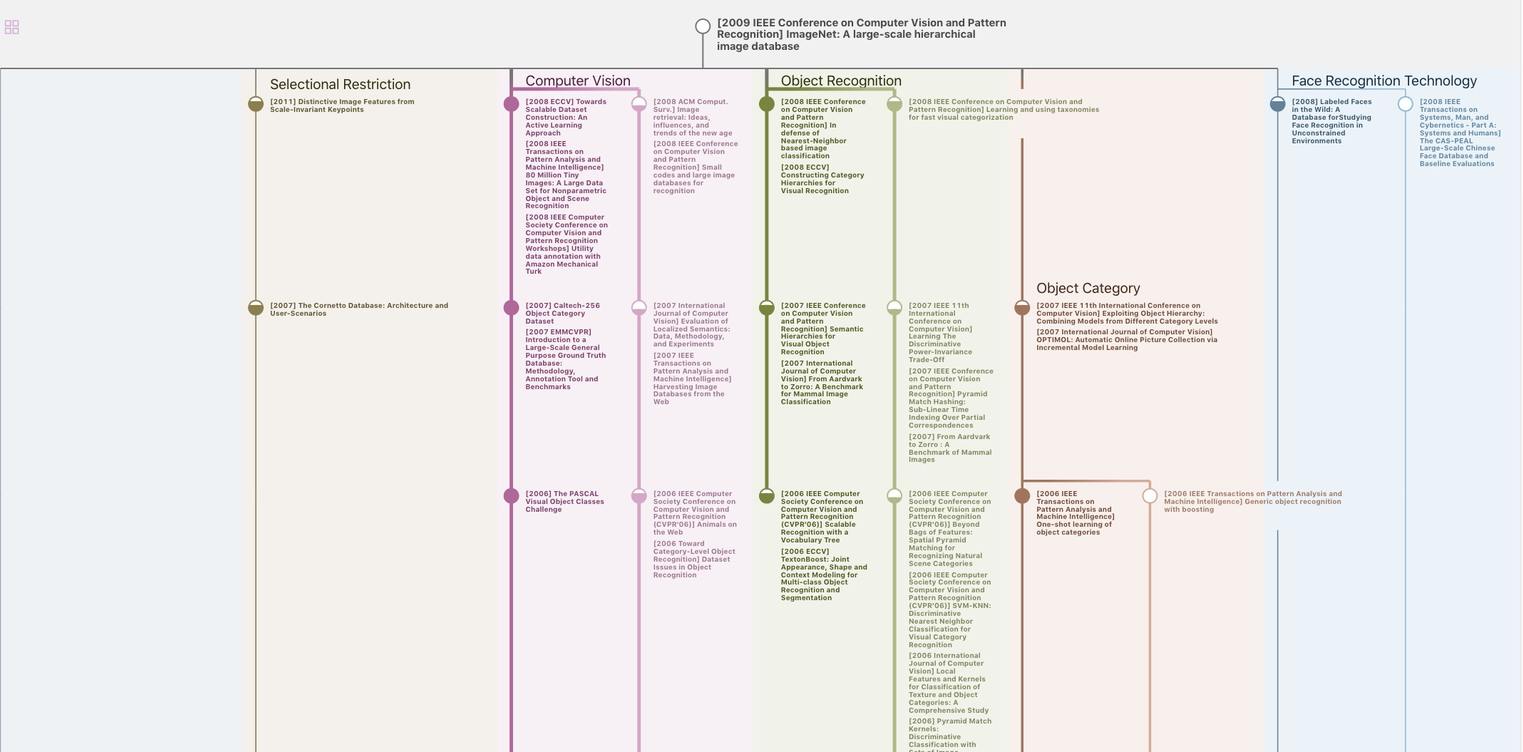
生成溯源树,研究论文发展脉络
Chat Paper
正在生成论文摘要