Machine Learning-based Gesture Recognition UsingWearable Devices
2022 INTERNATIONAL CONFERENCE ON CYBER-ENABLED DISTRIBUTED COMPUTING AND KNOWLEDGE DISCOVERY, CYBERC(2022)
摘要
Traditional gesture recognition solutions are based on touch screens or vision, limited by environmental conditions and not portable. The accelerometer-based gesture recognition technology can be integrated into small wearable smart devices, such as smart bracelets, smartwatches or smart rings. The portability and reliability of this technology make it a broad market and application space. This project is based on a smartwatch accelerometer dataset from TensorFlow Datasets. By experimenting with two different pre-processing algorithms: Kalman Filter and Savitzky-Golay Filter, feature extraction algorithms and machine learning algorithms (random forests, k-nearest neighbours, support vector machine), the relatively optimal algorithm for each part to combine to obtain a good accelerometer-based gesture recognition model were filtered out, including gravity reduction, Fourier transforms, a normal exception elimination algorithm, Savitzky-Golay Filter and Support Vector Machine (SVM). The best accuracy rate of this model is over 97%, with a similar degree of precision, recall rate and f1 score.
更多查看译文
关键词
Machine Learning, Gesture Recognition, Signal Processing, Feature Engineering
AI 理解论文
溯源树
样例
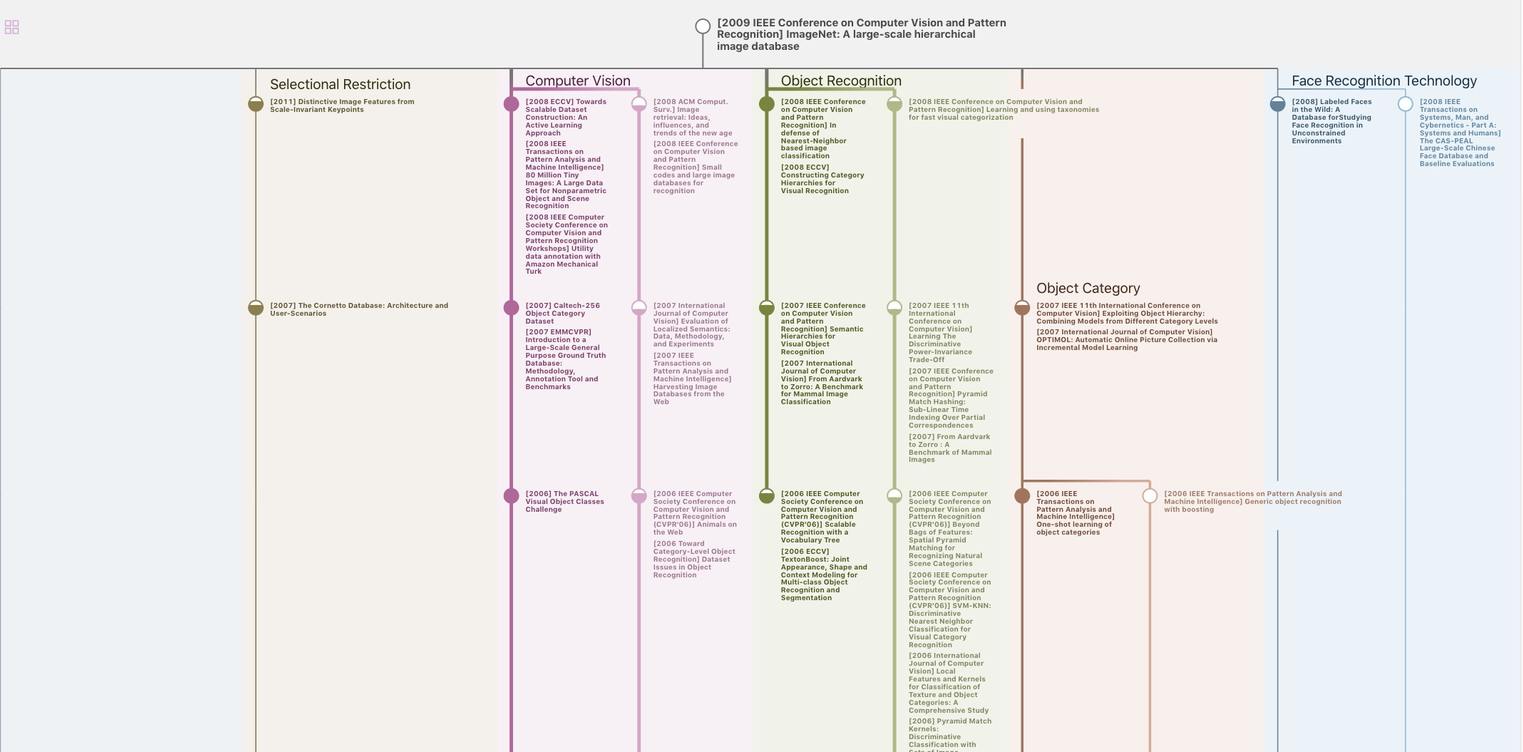
生成溯源树,研究论文发展脉络
Chat Paper
正在生成论文摘要