Assessing Perceived Trust and Satisfaction with Multiple Explanation Techniques in XAI-Enhanced Learning Analytics
ELECTRONICS(2023)
摘要
This study aimed to observe the impact of eight explainable AI (XAI) explanation techniques on user trust and satisfaction in the context of XAI-enhanced learning analytics while comparing two groups of STEM college students based on their Bologna study level, using various established feature relevance techniques, certainty, and comparison explanations. Overall, the students reported the highest trust in local feature explanation in the form of a bar graph. Additionally, master's students presented with global feature explanations also reported high trust in this form of explanation. The highest measured explanation satisfaction was observed with the local feature explanation technique in the group of bachelor's and master's students, with master's students additionally expressing high satisfaction with the global feature importance explanation. A detailed overview shows that the two observed groups of students displayed consensus in favored explanation techniques when evaluating trust and explanation satisfaction. Certainty explanation techniques were perceived with lower trust and satisfaction than were local feature relevance explanation techniques. The correlation between itemized results was documented and measured with the Trust in Automation questionnaire and Explanation Satisfaction Scale questionnaire. Master's-level students self-reported an overall higher understanding of the explanations and higher overall satisfaction with explanations and perceived the explanations as less harmful.
更多查看译文
关键词
Explainable Artificial Intelligence,learning analytics,XAI,XAI techniques,trust,explanation satisfaction
AI 理解论文
溯源树
样例
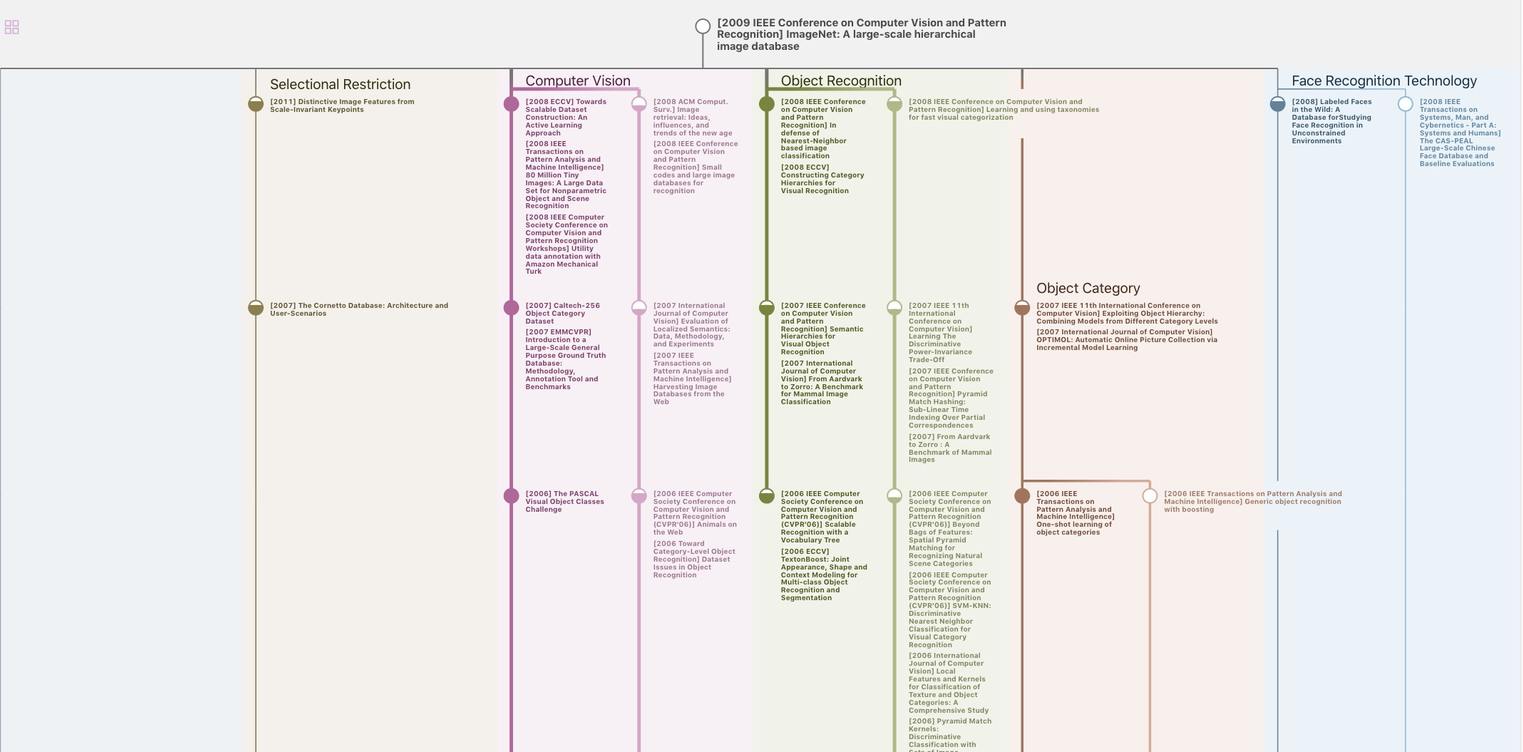
生成溯源树,研究论文发展脉络
Chat Paper
正在生成论文摘要