Automatic Machine Learning Combined with High-Throughput Computational Screening of Hydrophobic Metal-Organic Frameworks for Capture of Methanol and Ethanol from the Air
ACS ES&T ENGINEERING(2023)
摘要
The capture of low concentration alcohol VOCs (methanoland ethanol)from the air has also attracted more and more attention. In this work,high-throughput computational screening (HTCS) and machine learning(ML) methods based on molecular simulations were used to investigatethe adsorption properties of methanol and ethanol in 31 399hydrophobic metal-organic frameworks (MOFs). First, the structure-performancerelationship of MOFs was successfully established through univariateanalysis, and the key descriptors identified were LCD and Q (0) (st). Five ML methods, Decision Tree(DT), Random Forest (RF), Back Propagation Neural Network (BPNN),Support Vector Machines (SVM), and Tree-based Pipeline OptimizationTool (TPOT), were used to predict the adsorption performance of MOFs.The automatic machine learning (Auto-ML) algorithm TPOT has the bestprediction effect on the TSN of methanol and ethanol, with R (2) values of 0.852 and 0.945, respectively. Theaccuracy of the ML model was further improved using the random searchmethod. Analysis of the algorithms has revealed that GBR and RFR havethe highest prediction accuracy and frequency, respectively, for theMOF-methanol and MOF-ethanol systems. Ten MOF materialswith excellent adsorption properties (0.002 mol/kg & GE; N (CH3OH) & GE; 0.001 mol/kg, 0.068 mol/kg & GE; N (C2H5OH) & GE; 0.016 mol/kg; 420.67 & GE; S (CH3OH) & GE; 214.29, 3.2 x 10(6) & GE; S (C2H5OH) & GE; 8.5 x10(3)) were selected successfully. After analysis of theiradsorption sites, it was found that the primary adsorption sites formethanol and ethanol are located near the amino and halogen groups,and the different metal centers showed great influence on the adsorptioncapacity of MOFs for two kinds of alcohol molecules through the analysisof their structural commonness. This work can serve as a roadmap forexperimental synthesis, innovative design of MOFs, and the developmentof new ML algorithms.
更多查看译文
关键词
metal–organic frameworks,hydrophobic metal–organic,methanol,methanol,automatic machine learning combined,high-throughput
AI 理解论文
溯源树
样例
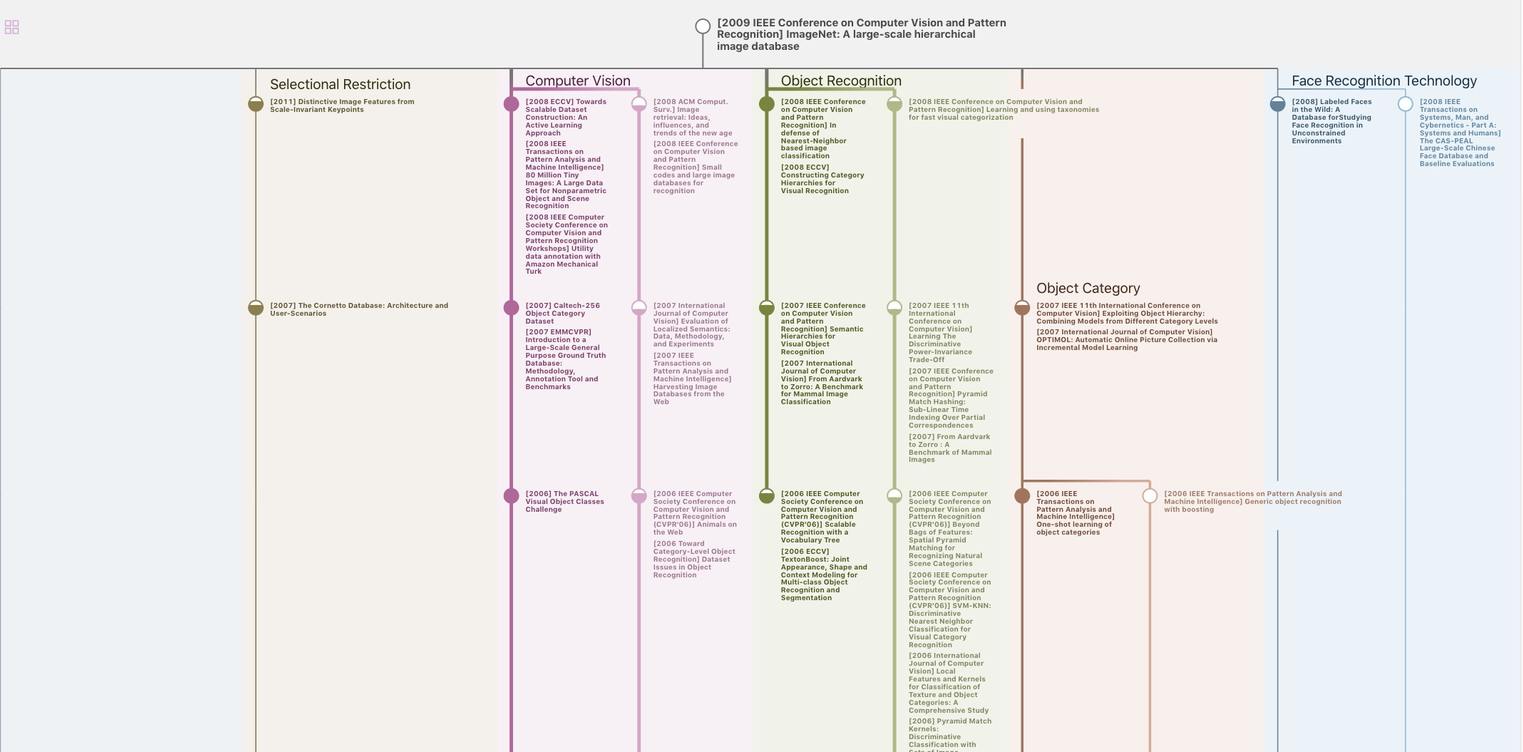
生成溯源树,研究论文发展脉络
Chat Paper
正在生成论文摘要