Classification of drainage crossings on high-resolution digital elevation models: A deep learning approach
GISCIENCE & REMOTE SENSING(2023)
摘要
High-Resolution Digital Elevation Models (HRDEMs) have been used to delineate fine-scale hydrographic features in landscapes with relatively level topography. However, artificial flow barriers associated with roads are known to cause incorrect modeled flowlines, because these barriers substantially increase the terrain elevation and often terminate flowlines. A common practice is to breach the elevation of roads near drainage crossing locations, which, however, are often unavailable. Thus, developing a reliable drainage crossing dataset is essential to improve the HRDEMs for hydrographic delineation. The purpose of this research is to develop deep learning models for classifying the images that contain the locations of flow barriers. Based on HRDEMs and aerial orthophotos, different Convolutional Neural Network (CNN) models were trained and compared to assess their effectiveness in image classification in four different watersheds across the U.S. Midwest. Our results show that most deep learning models can consistently achieve over 90% accuracies. The CNN model with HRDEMs as the sole input feature was found to be the best-fit one. The addition of aerial orthophotos and their derived spectral indices is insignificant to or even worsens the model's accuracy. The selected best-fit model exhibits excellent transferability over different geographic contexts. This work can be applied to improve elevation-derived hydrography mapping at fine spatial scales.
更多查看译文
关键词
drainage crossings,digital elevation models,deep learning,high-resolution
AI 理解论文
溯源树
样例
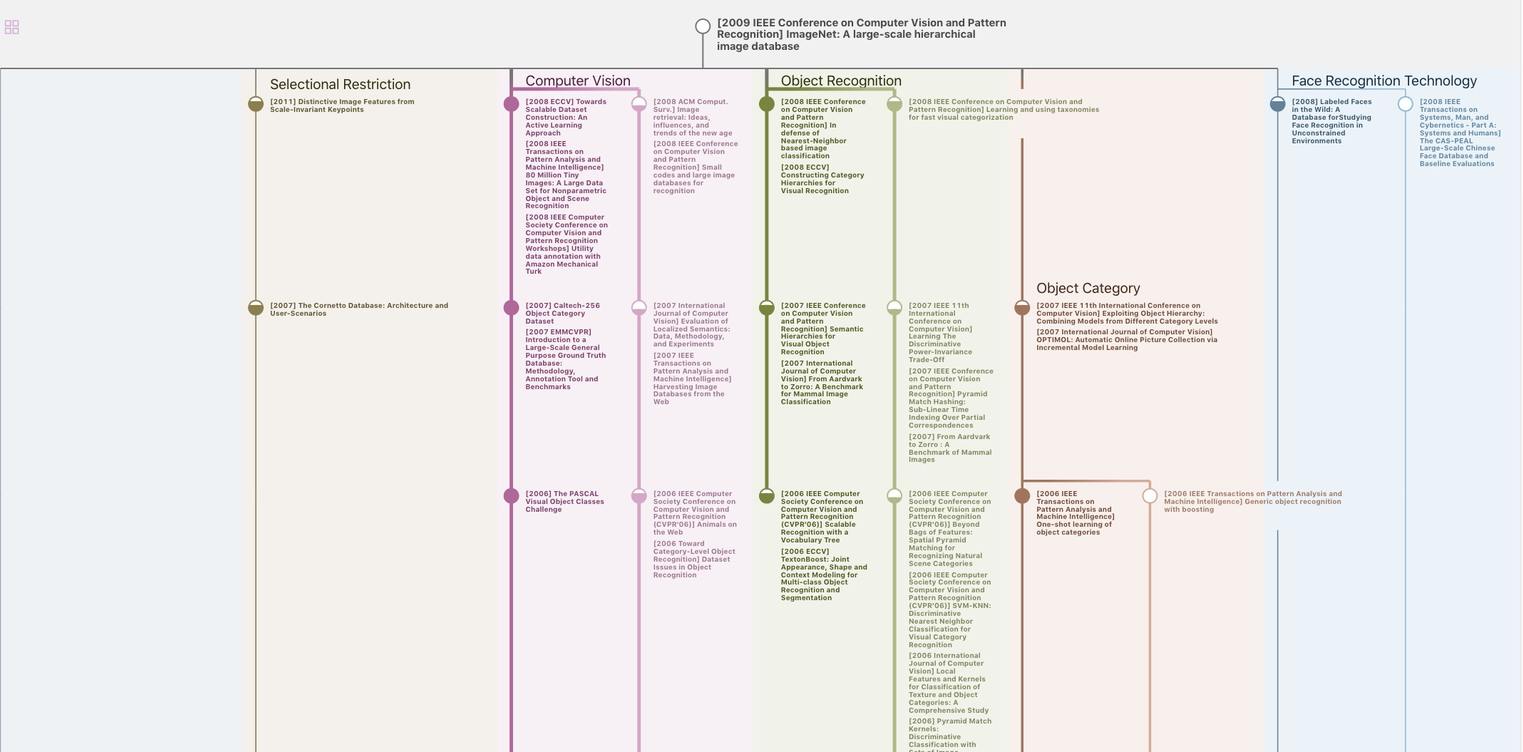
生成溯源树,研究论文发展脉络
Chat Paper
正在生成论文摘要