Paricle identification at VAMOS plus plus with machine learning techniques
NUCLEAR INSTRUMENTS & METHODS IN PHYSICS RESEARCH SECTION B-BEAM INTERACTIONS WITH MATERIALS AND ATOMS(2023)
摘要
Multi-nucleon transfer reaction between 136Xe beam and 198Pt target was performed using the VAMOS++ spectrometer at GANIL to study the structure of n-rich nuclei around N=126. Unambiguous charge state identification was obtained by combining two supervised machine learning methods, deep neural network (DNN) and positional correction using a gradient-boosting decision tree (GBDT). The new method reduced the complexity of the kinetic energy calibration and outperformed the conventional method improving the charge state resolution by 8%.
更多查看译文
关键词
VAMOS plus plus, Machine learning, Multi-nucleon transfer reaction
AI 理解论文
溯源树
样例
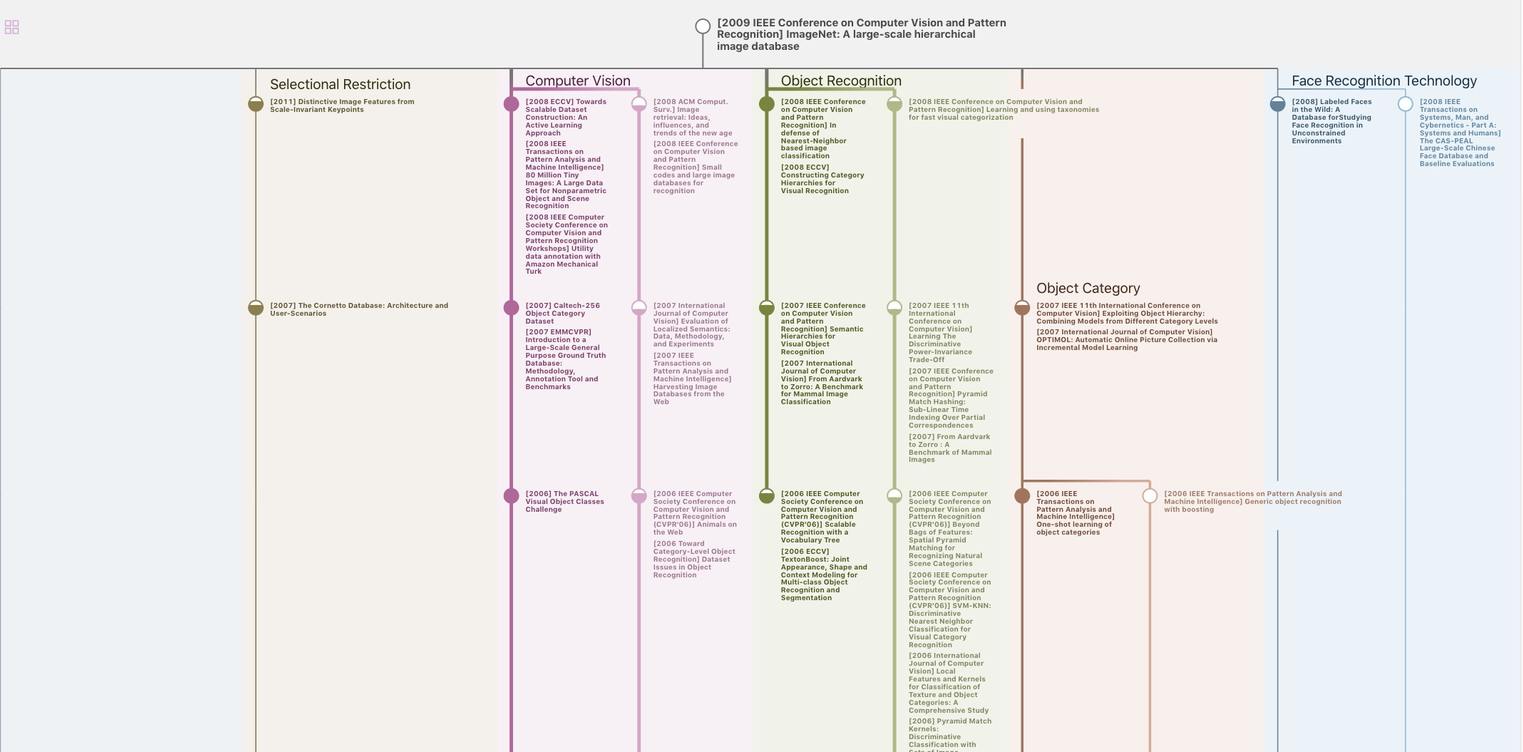
生成溯源树,研究论文发展脉络
Chat Paper
正在生成论文摘要