Remaining Useful Life Prognostics and Uncertainty Quantification for Aircraft Engines Based on Convolutional Bayesian Long Short-Term Memory Neural Network
2023 PROGNOSTICS AND HEALTH MANAGEMENT CONFERENCE, PHM(2023)
摘要
Remaining Useful Life (RUL) prognostics and pre-failure warning for complex industrial systems enables the timely detection of hidden problems and effectively avoids multiple accidents. Therefore, highly accurate and reliable RUL prediction is crucial. Bayesian neural networks can model the uncertainty in the process of equipment degradation while effectively assessing RUL, which helps to implement reliable risk analysis and maintenance decisions. In this paper, we propose a Convolutional Bayesian Long Short-Term Memory neural network (CB-LSTM)-based RUL prediction algorithm, which uses a Convolutional Neural Network (CNN) to implicitly extract features from training data, to generate an abstract representation of the input signal, and combine it with a Bayesian Long Short-Term Memory neural network (B-LSTM) to build a multivariate time series prediction model. The method is validated on the C-MAPSS dataset by NASA. The experimental results show that the method has good prediction accuracy and uncertainty quantification ability.
更多查看译文
关键词
CB-LSTM,CNN,RUL prognostics,uncertainty quantification,Aircraft engine
AI 理解论文
溯源树
样例
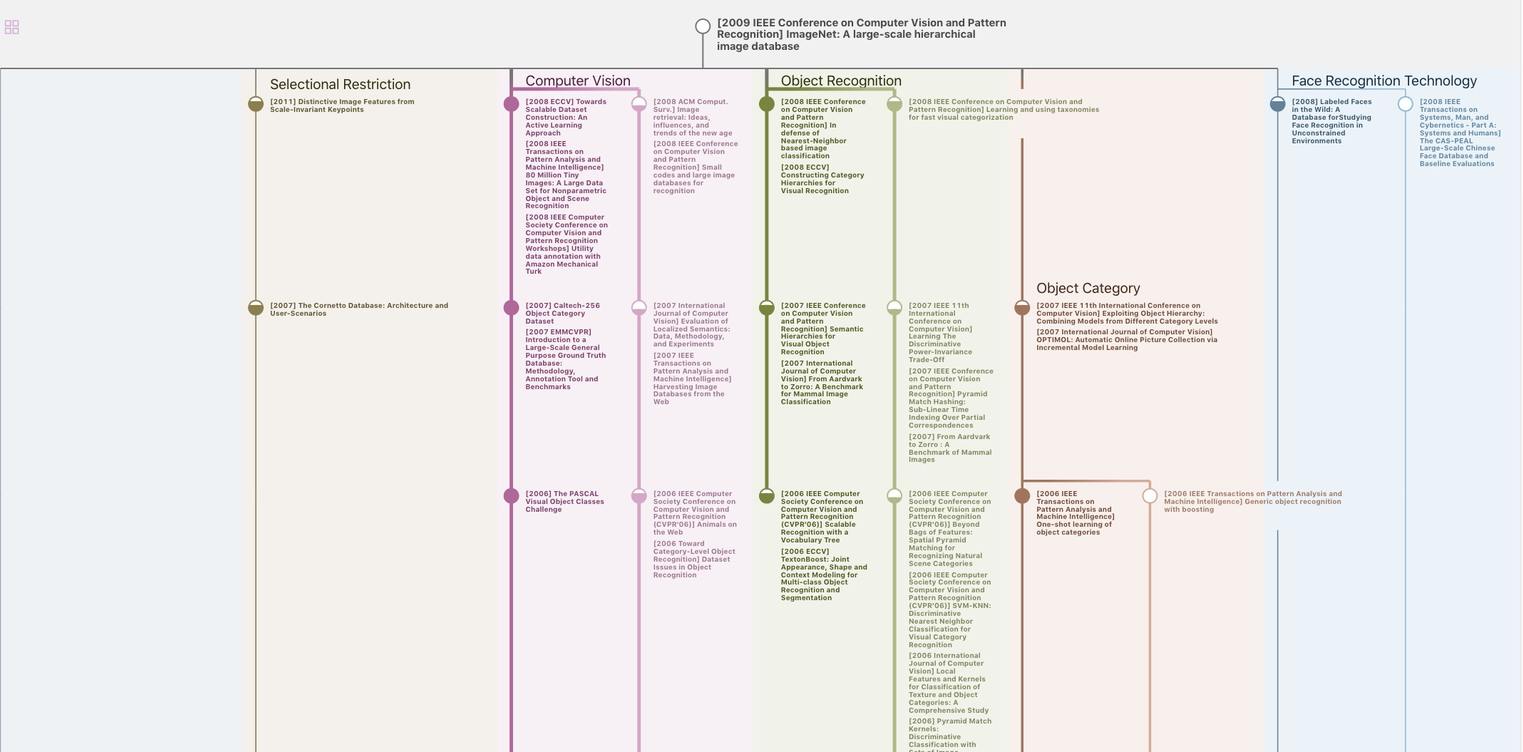
生成溯源树,研究论文发展脉络
Chat Paper
正在生成论文摘要