On the Performance of Machine Learning Fairness in Image Classification
ARTIFICIAL INTELLIGENCE AND MACHINE LEARNING FOR MULTI-DOMAIN OPERATIONS APPLICATIONS V(2023)
摘要
In recent years, computer vision has made significant strides in enabling machines to perform a wide range of tasks, from image classification and segmentation to image generation and video analysis. It is a rapidly evolving field that aims to enable machines to interpret and understand visual information from the environment. One key task in computer vision is image classification, where algorithms identify and categorize objects in images based on their visual features. Image classification has a wide range of applications, from image search and recommendation systems to autonomous driving and medical diagnosis. However, recent research has highlighted the presence of bias in image classification algorithms, particularly with respect to human-sensitive attributes such as gender, race, and ethnicity. Some examples are computer programmers being predicted better in the context of men in images compared to women, and the accuracy of the algorithm being better on greyscale images compared to colored images. This discrepancy in identifying objects is developed through correlation the algorithm learns from the objects in context known as contextual bias. This bias can result in inaccurate decisions, with potential consequences in areas such as hiring, healthcare, and security. In this paper, we conduct an empirical study to investigate bias in the image classification domain based on sensitive attribute gender using deep convolutional neural networks (CNN) through transfer learning and minimize bias within the image context using data augmentation to improve overall model performance. In addition, cross-data generalization experiments are conducted to evaluate model robustness across popular open-source image datasets.
更多查看译文
关键词
Convolutional Neural Network,Image Classification,Transfer Learning,Equitable AI,Trustworthy AI,Fairness,Ethics
AI 理解论文
溯源树
样例
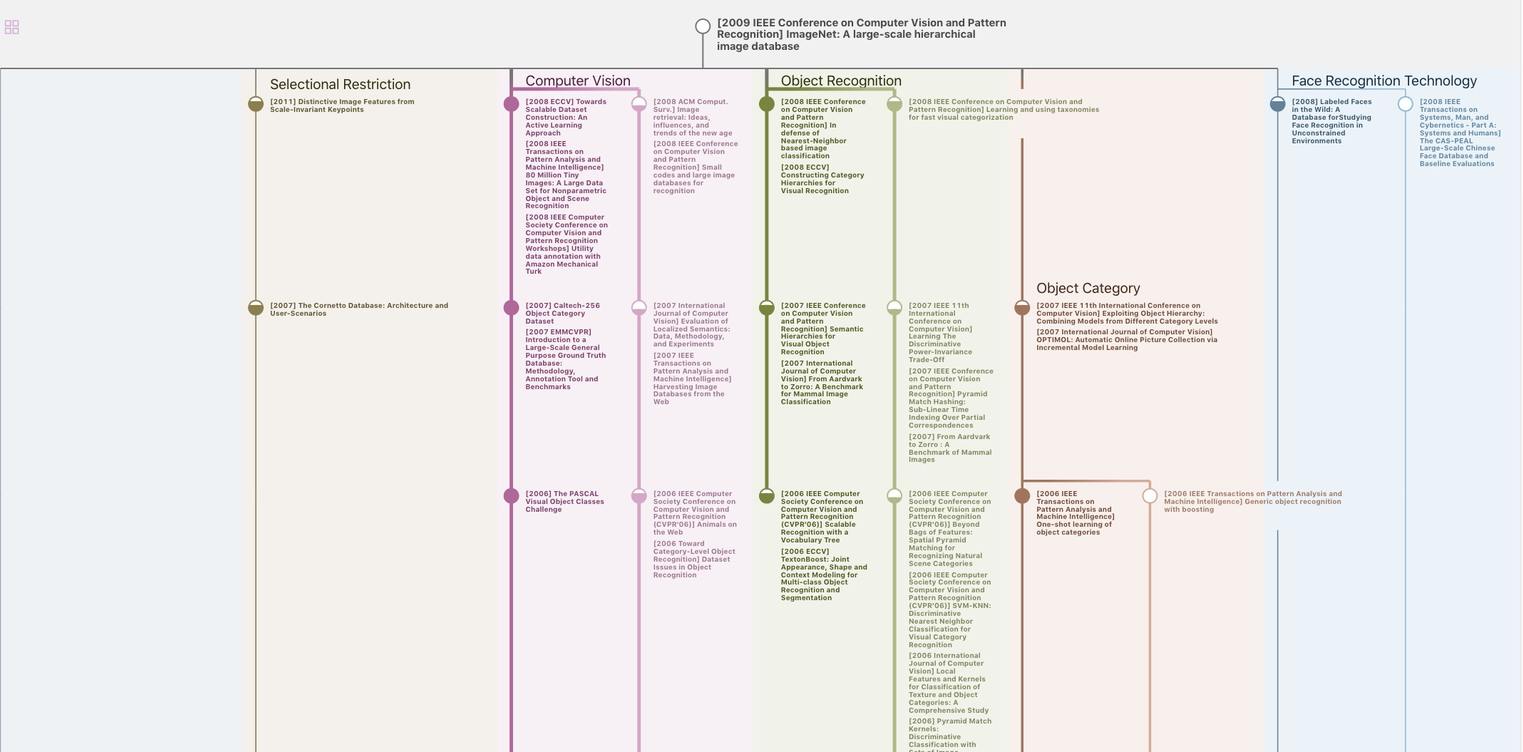
生成溯源树,研究论文发展脉络
Chat Paper
正在生成论文摘要