An Algorithm Adaptation Method for Multi-Label Stream Classification using Self-Organizing Maps
2022 21ST IEEE INTERNATIONAL CONFERENCE ON MACHINE LEARNING AND APPLICATIONS, ICMLA(2022)
摘要
Multi-label stream classification is the task of classifying instances in two or more classes simultaneously, with instances flowing continuously in high speed. This task imposes difficult challenges, such as the detection of concept drifts, where the distributions of the instances in the stream change with time, and infinitely delayed labels, when the ground truth labels of the instances are never available to help updating the classifiers. To solve such task, the methods from the literature use the problem transformation approach, which divides the multi-label problem into different sub-problems, associating one classification model for each class. In this paper, we propose a method based on self-organizing maps that, different from the literature, uses only one model to deal with all classes simultaneously. By using the algorithm adaptation approach, our proposal better considers label dependencies, improving the results over its counterparts. Experiments using different synthetic and real-world datasets showed that our proposal obtained the overall best performance when compared to different methods from the literature.
更多查看译文
关键词
Multi-Label Classification, Stream Classification, Concept Drift, Neural Networks
AI 理解论文
溯源树
样例
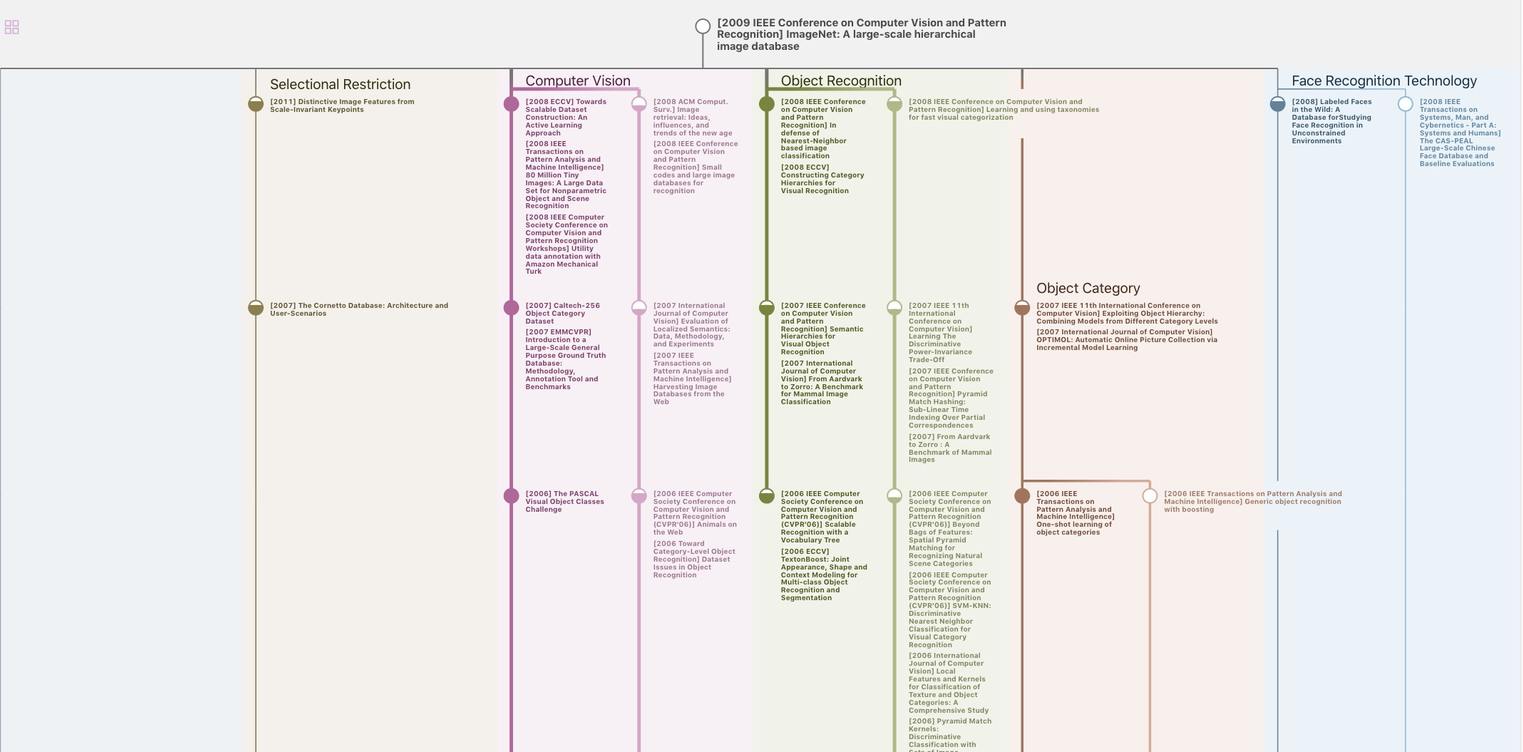
生成溯源树,研究论文发展脉络
Chat Paper
正在生成论文摘要